Unsupervised Exemplar-Based Image-to-Image Translation and Cascaded Vision Transformers for Tagged and Untagged Cardiac Cine MRI Registration.
IEEE/CVF Winter Conference on Applications of Computer Vision(2024)
摘要
Multi-modal registration between tagged and untagged cardiac cine magnetic resonance (MR) images remains difficult, due to the domain gap and large deformations between the two modalities. Recent work using an image-to-image translation (I2I) module to overcome the domain gap can convert the multi-modal into a mono-modal registration task and take advantage of advanced mono-modal registration architectures. However, they often ignore two issues: the sample-specific style of each image to be registered during I2I and large hybrid rigid and non-rigid deformations between modalities. We first propose an exemplar-based I2I module capable of unsupervised cross-domain correspondence learning to enforce the style consistency between the fake image and the image to be registered. Then we propose an efficient cascaded vision transformer-based registration network to predict both the affine and non-rigid deformations, in which a single feature embedding subnetwork is shared by the two stages of deformation prediction. We validated our method on a clinical cardiac MR dataset with paired but unaligned untagged and tagged MR images. The results show that our method outperforms traditional methods significantly in terms of the I2I quality and multi-modal image registration accuracy.
更多查看译文
关键词
Applications,Biomedical / healthcare / medicine,Algorithms,Generative models for image,video,3D,etc
AI 理解论文
溯源树
样例
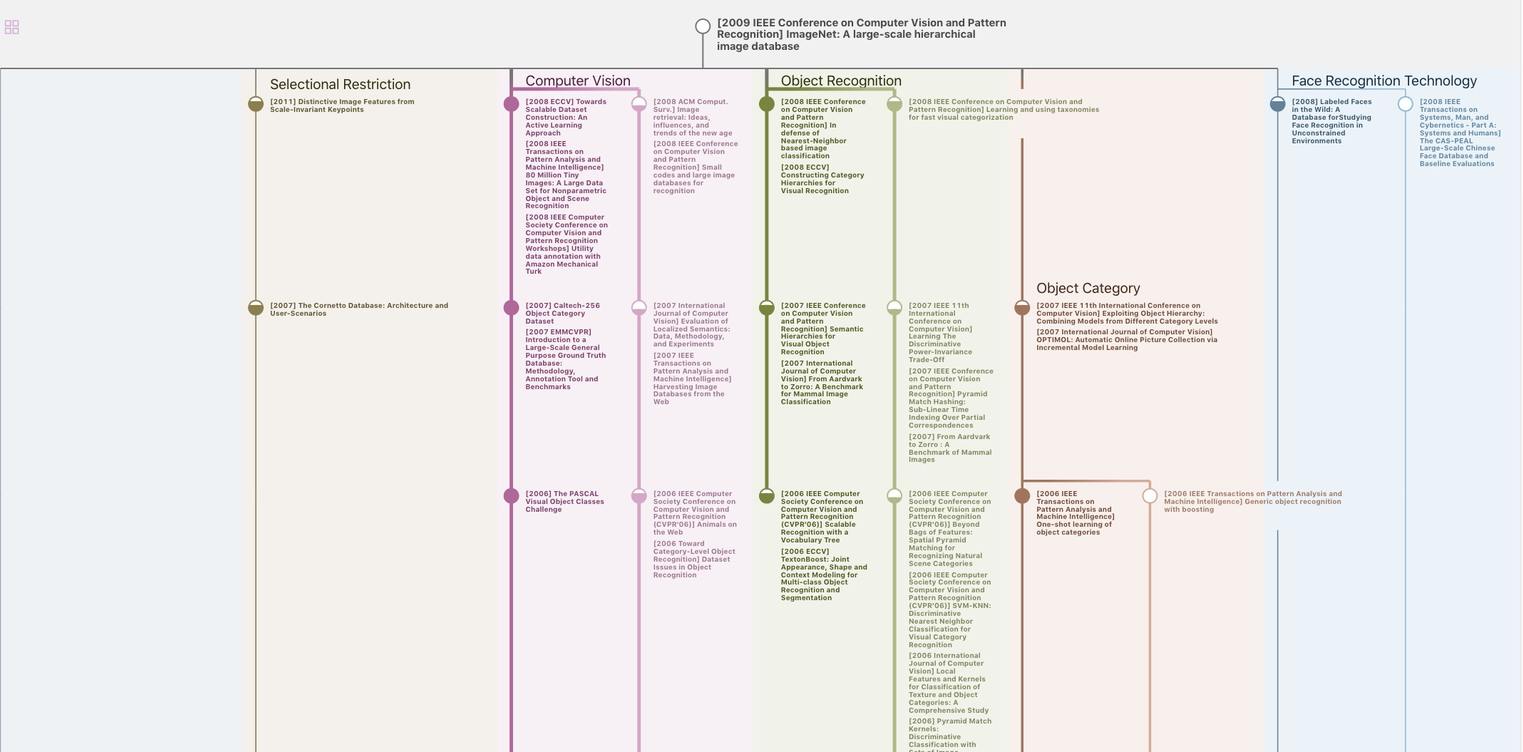
生成溯源树,研究论文发展脉络
Chat Paper
正在生成论文摘要