Eagle and Finch: RWKV with Matrix-Valued States and Dynamic Recurrence
arxiv(2024)
摘要
We present Eagle (RWKV-5) and Finch (RWKV-6), sequence models improving upon
the RWKV (RWKV-4) architecture. Our architectural design advancements include
multi-headed matrix-valued states and a dynamic recurrence mechanism that
improve expressivity while maintaining the inference efficiency characteristics
of RNNs. We introduce a new multilingual corpus with 1.12 trillion tokens and a
fast tokenizer based on greedy matching for enhanced multilinguality. We
trained four Eagle models, ranging from 0.46 to 7.5 billion parameters, and two
Finch models with 1.6 and 3.1 billion parameters and find that they achieve
competitive performance across a wide variety of benchmarks. We release all our
models on HuggingFace under the Apache 2.0 license. Models at:
https://huggingface.co/RWKV Training code at: https://github.com/RWKV/RWKV-LM
Inference code at: https://github.com/RWKV/ChatRWKV Time-parallel training code
at: https://github.com/RWKV/RWKV-infctx-trainer
更多查看译文
AI 理解论文
溯源树
样例
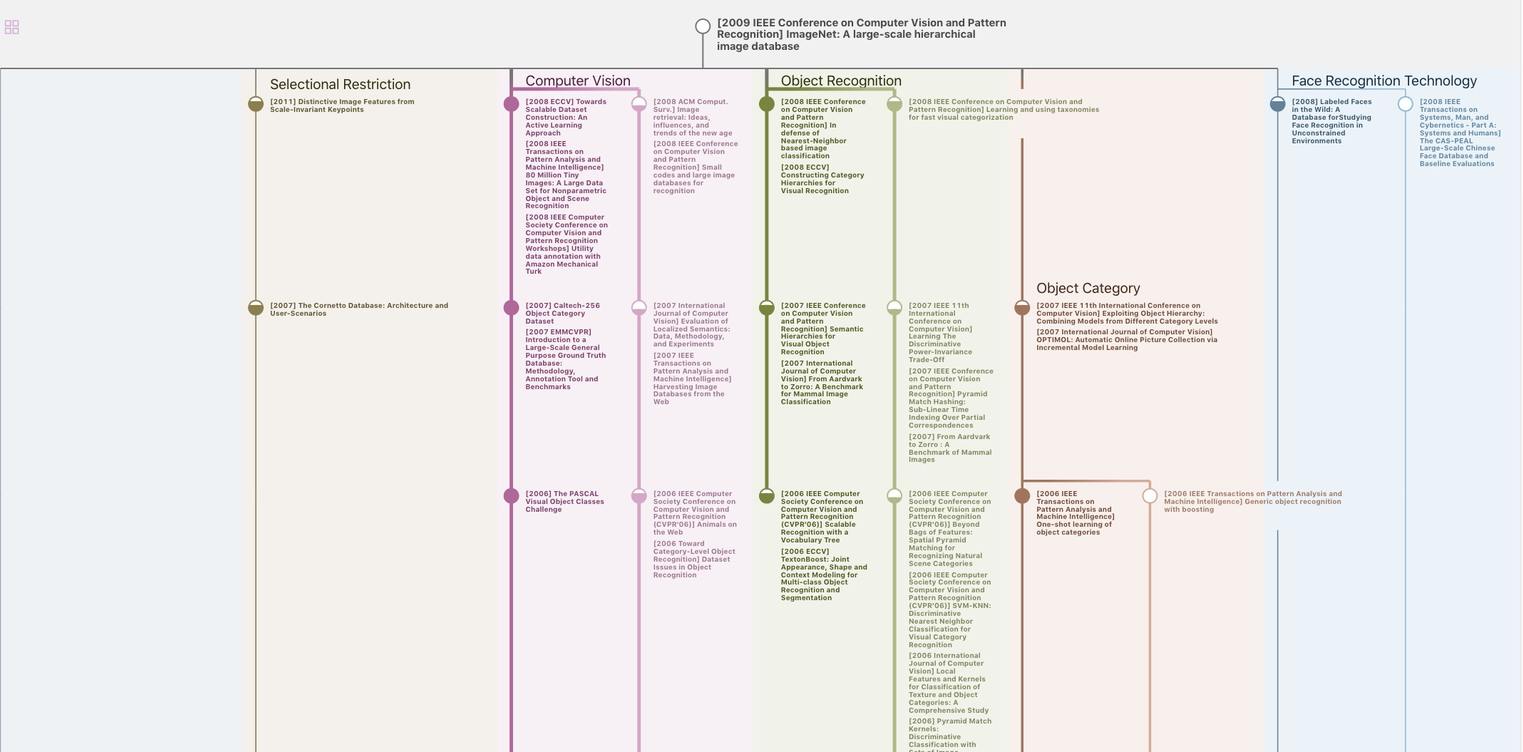
生成溯源树,研究论文发展脉络
Chat Paper
正在生成论文摘要