PhISH-Net: Physics Inspired System for High Resolution Underwater Image Enhancement.
IEEE/CVF Winter Conference on Applications of Computer Vision(2024)
摘要
Underwater imaging presents numerous challenges due to refraction, light absorption, and scattering, resulting in color degradation, low contrast, and blurriness. Enhancing underwater images is crucial for high-level computer vision tasks, but existing methods either neglect the physics-based image formation process or require expensive computations. In this paper, we propose an effective framework that combines a physics-based Underwater Image Formation Model (UIFM) with a deep image enhancement approach based on the retinex model. Firstly, we remove backscatter by estimating attenuation coefficients using depth information. Then, we employ a retinex model-based deep image enhancement module to enhance the images. To ensure adherence to the UIFM, we introduce a novel Wideband Attenuation prior. The proposed PhISH-Net framework achieves real-time processing of high-resolution underwater images using a lightweight neural network and a bilateral-grid-based upsampler. Extensive experiments on two underwater image datasets demonstrate the superior performance of our method compared to state-of-the-art techniques. Additionally, qualitative evaluation on a cross-dataset scenario confirms its generalization capability. Our contributions lie in combining the physics-based UIFM with deep image enhancement methods, introducing the wideband attenuation prior, and achieving superior performance and efficiency.
更多查看译文
关键词
Algorithms,Low-level and physics-based vision,Algorithms,Computational photography,image and video synthesis
AI 理解论文
溯源树
样例
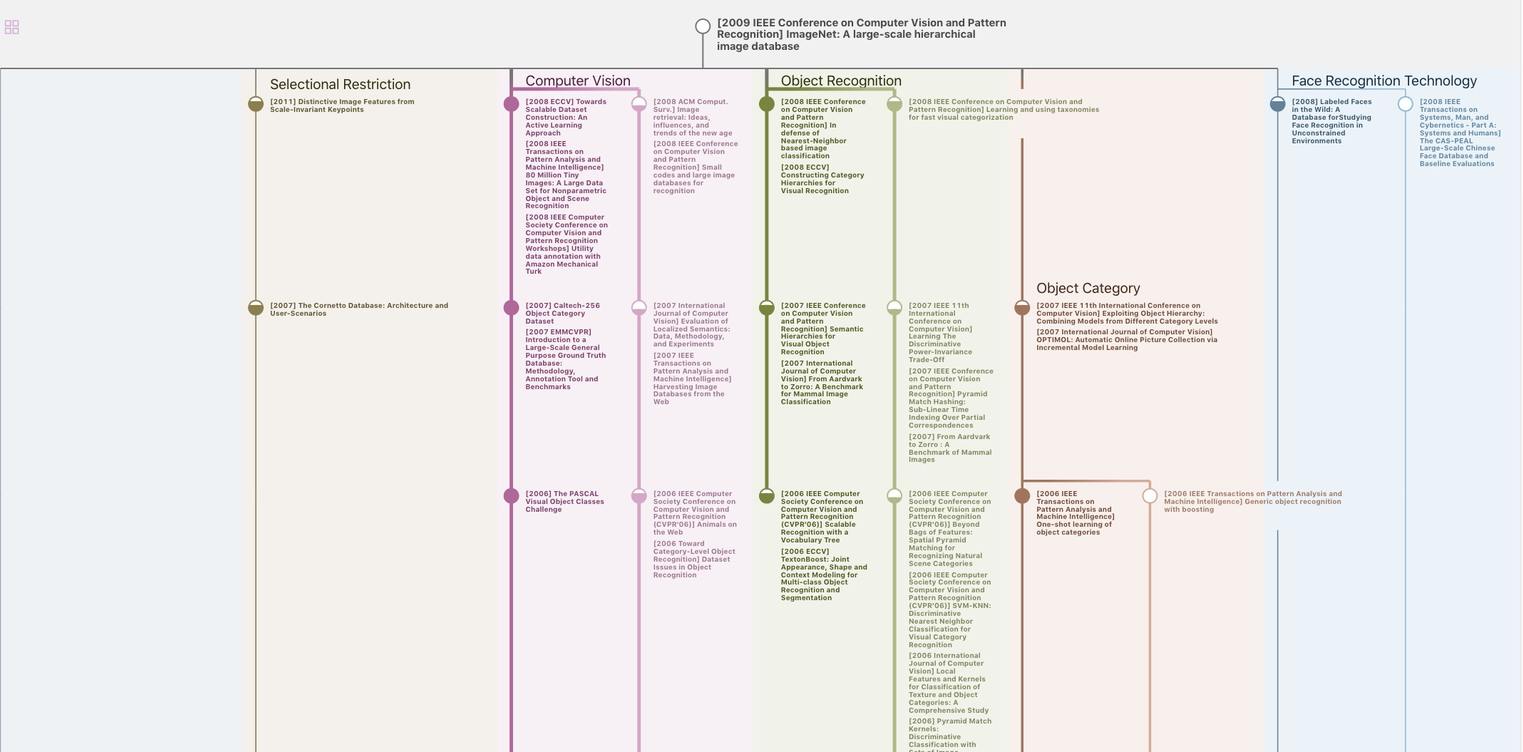
生成溯源树,研究论文发展脉络
Chat Paper
正在生成论文摘要