Posterior circulation ischemic stroke: radiomics-based machine learning approach to identify onset time from magnetic resonance imaging
Neuroradiology(2024)
摘要
Posterior circulation ischemic stroke (PCIS) possesses unique features. However, previous studies have primarily or exclusively relied on anterior circulation stroke cases to build machine learning (ML) models for predicting onset time. To date, there is no research reporting the effectiveness and stability of ML in identifying PCIS onset time. We aimed to build diffusion-weighted imaging-based ML models to identify the onset time of PCIS patients. Consecutive PCIS patients within 24 h of definite symptom onset were included (112 in the training set and 49 in the independent test set). Images were processed as follows: volume of interest segmentation, image feature extraction, and feature selection. Five ML models, naïve Bayes, logistic regression, tree ensemble, k-nearest neighbor, and random forest, were built based on the training set to estimate the stroke onset time (binary classification: ≤ 4.5 h or > 4.5 h). Relative standard deviations (RSD), receiver operating characteristic (ROC) curves, and the calibration plot was performed to evaluate the stability and performance of the five models. The random forest model had the best performance in the test set, with the highest area under the curve (AUC, 0.840; 95
更多查看译文
关键词
Ischemic stroke,Radiomics,Machine learning,Diffusion magnetic resonance imaging,Posterior circulation
AI 理解论文
溯源树
样例
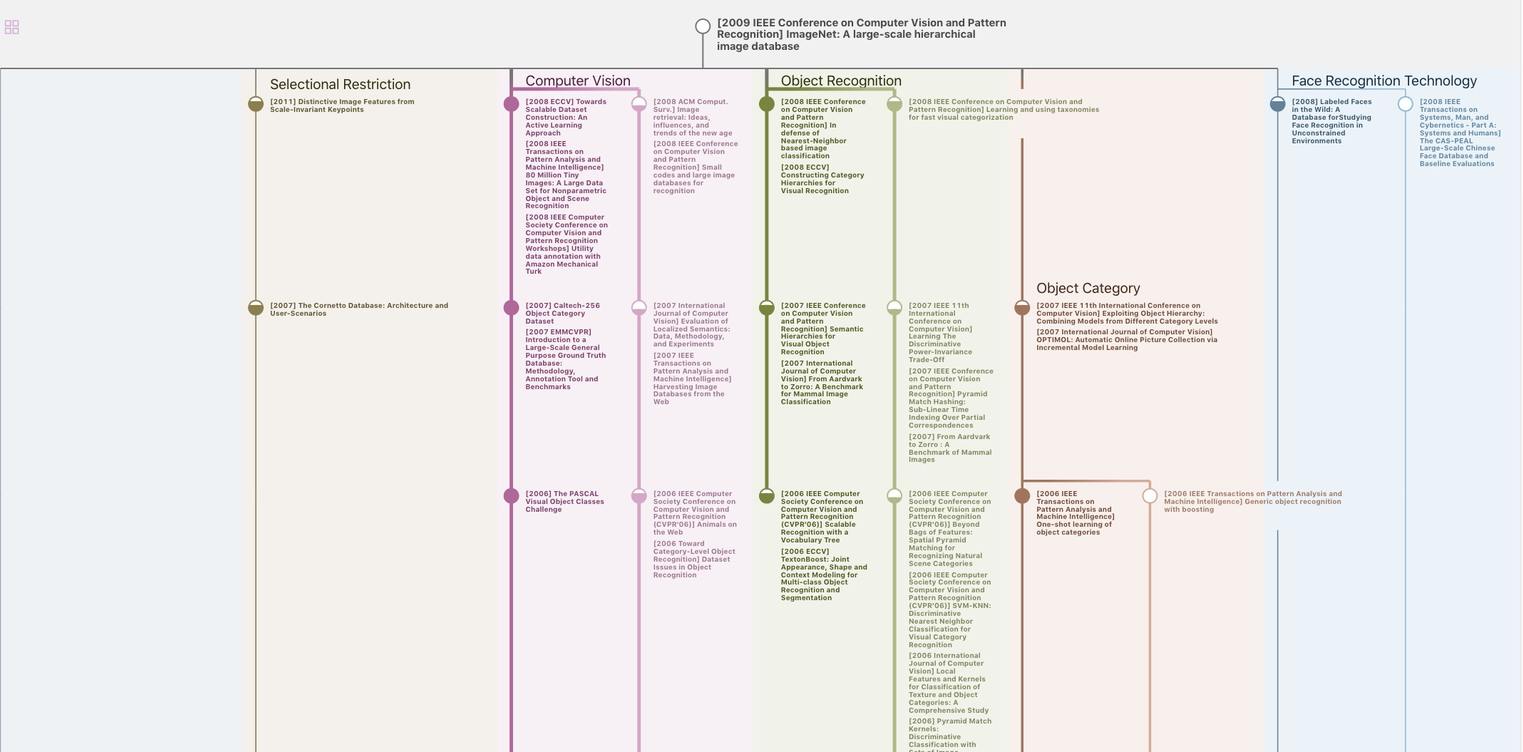
生成溯源树,研究论文发展脉络
Chat Paper
正在生成论文摘要