Energy-Efficient Carrier Aggregation in 5G Using Constrained Multi-Agent MDP
IEEE Transactions on Green Communications and Networking(2024)
摘要
Carrier Aggregation (CA) is a promising technology in LTE and 5G networks that enhances the throughput of the users. However, since each User Equipment (UE) has to continuously monitor the activated Component Carriers (CCs) in CA, the UE energy consumption increases. To reduce the energy consumption while maximizing the throughput of UEs, we propose a dynamic and proactive CC management scheme for 5G, using a Q-Learning algorithm. To address our problem, we first model the corresponding Constrained Multi-agent Markov Decision Process (CMMDP) model and then utilize the Q-Learning algorithm to solve it. The time inter-arrival and the size of the next incoming bursts of data are proactively predicted and, along with the data in the buffer, are considered in the state space and the reward function of the machine learning model. Our proposed scheme is compared to three baseline schemes. In the first and second baseline algorithms, all CCs and only single CC are activated for each UE, respectively. For the last baseline algorithm, we simplify our Reinforcement Learning (RL) algorithm, in which the remaining data in the scheduling buffer of users is not considered and also the throughput and the number of activated CCs is balanced in the low traffic load. Simulation results reveal that our proposed Q-Learning algorithm outperforms the baselines. It achieves the same throughput as the all CC activation algorithm while reducing the UE power consumption by about 20%. These benefits are achieved by dynamically activating and deactivating CCs according to the UE traffic pattern.
更多查看译文
关键词
5G,Carrier activation/deactivation,component carrier manager,traffic splitting,Reinforcement Learning,constrained multi-agent MDP
AI 理解论文
溯源树
样例
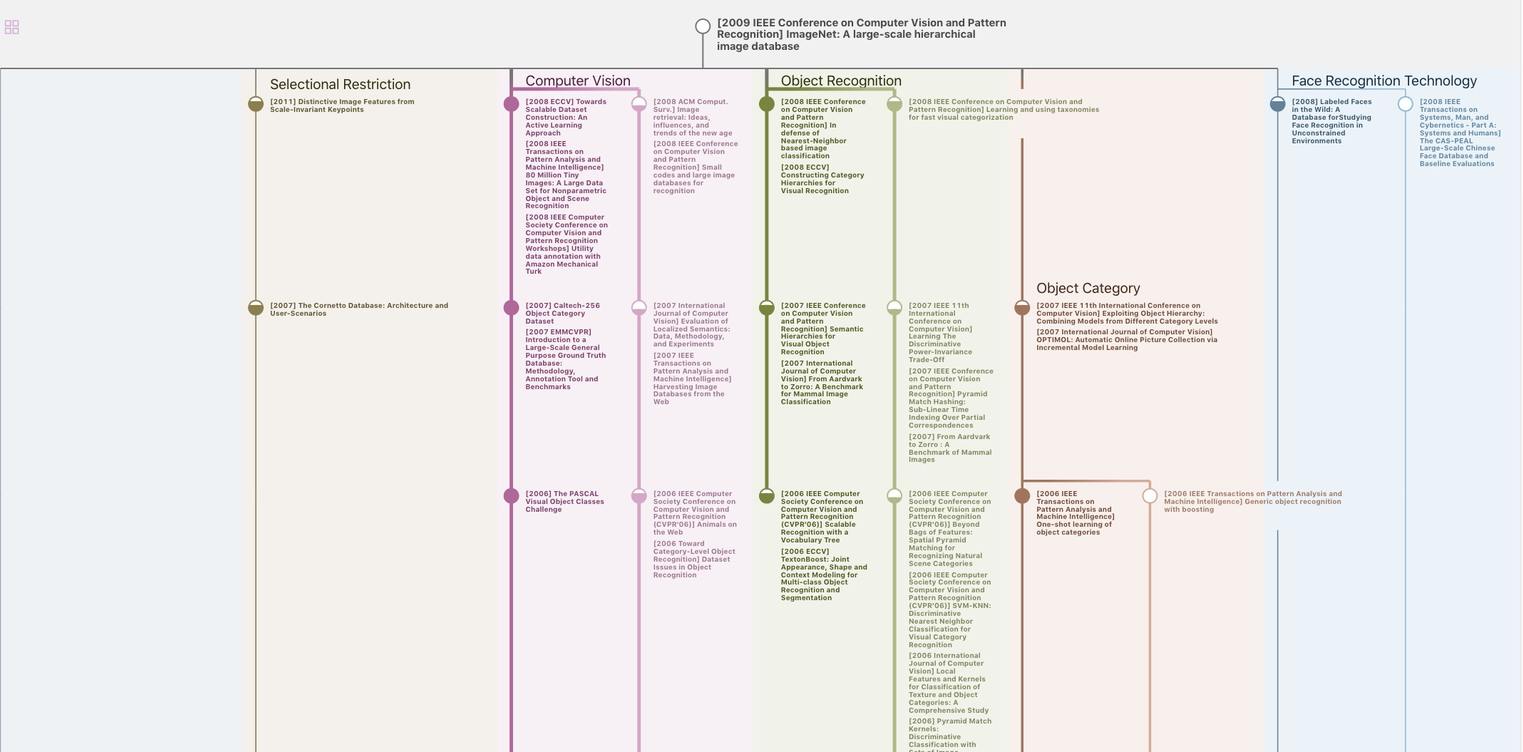
生成溯源树,研究论文发展脉络
Chat Paper
正在生成论文摘要