Multi-stream pyramid collaborative network for spectral unmixing
INTERNATIONAL JOURNAL OF REMOTE SENSING(2024)
摘要
Convolutional autoencoder, which can well model the spatial correlation of the data, have been widely applied to spectral unmixing task and achieved desirable performance. However, the fixed geometric structure of convolution kernels makes it difficult to capture global context. To address this issue, strategies such as dilated convolution or transformer are often employed, but this may result in minor loss of local details. Therefore, we propose a collaborative unmixing network with a multi-scale pyramid structure to capture both global and local features simultaneously. To integrate features from different scales in the unmixing process, we employ a cross-stream fusion feature strategy, which not only promote collaborative representations but also capture long-range dependencies while preserving local details. Meanwhile, we also design the residual spectral attention mechanism to refine the features from different scales and facilitate their fine-grained fusion. In the proposed network, each convolutional stream undergoes effective collaborative training using a convolutional autoencoder structure. The collaborative strategies include cross-stream feature fusion mechanism and alternating training strategy with weight sharing for endmember information. Experiments over three real hyperspectral datasets indicate the effectiveness of our method compared to other unmixing techniques.
更多查看译文
关键词
Spectral unmixing,deep learning,convolutional autoencoder,multi-scale,feature fusion,collaborative strategies
AI 理解论文
溯源树
样例
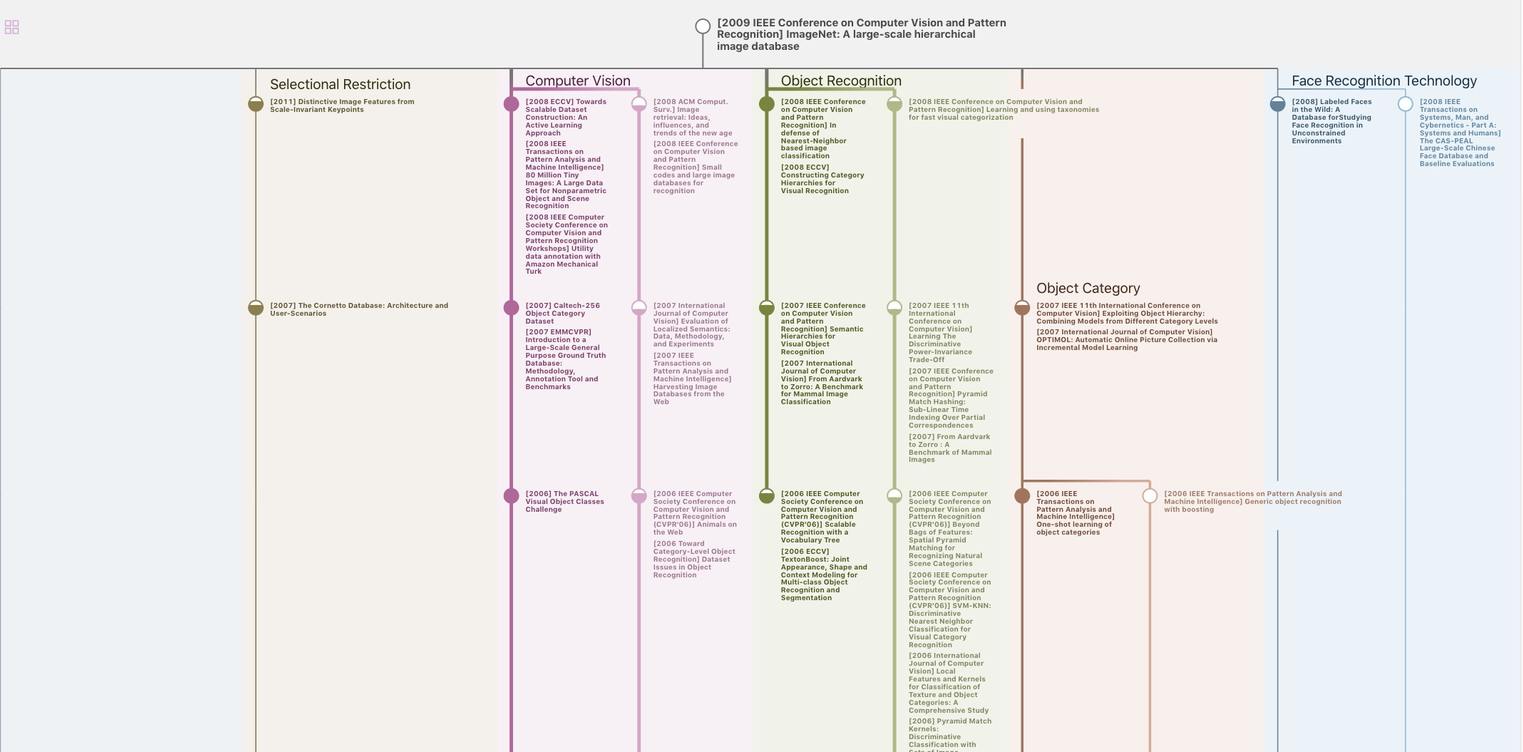
生成溯源树,研究论文发展脉络
Chat Paper
正在生成论文摘要