Fusion of hierarchical class graphs for remote sensing semantic segmentation
Information Fusion(2024)
摘要
Semantic segmentation of remote sensing images aim to assign a specific label or class to each pixel in an image, which plays an extremely important role in scene understanding. Currently, many advanced deep learning-based semantic segmentation methods have been developed. However, these methods are always based on disjoint labels to identify ground objects while ignoring the correlation (e.g., semantic, shapes, materials, etc.) among different ground objects, which limits the segmentation performance of remote sensing images. To solve this issue, we propose a hierarchical class graph for semantic segmentation of high resolution remote sensing images, which can learn structured relation among different ground objects. Specifically, first, we construct hierarchical class graphs based on different attributes and layers. Then, a three-layer hierarchical segmentation framework is developed to learn the correlation among different ground objects. Finally, a decision fusion method is designed to fuse the benefits of different hierarchical attributes and layers. More importantly, the influence of different hierarchical class graphs on the segmentation performance is detailedly analyzed. Extensive experiments on the iSAID and Vaihingen datasets reveal that all studied segmentation methods with hierarchical class graph can obtain better segmentation performance compared to ones without hierarchical class graph. The limitation of the proposed method is that the training time of the segmentation model tends to increase a bit because of considering the correlation among different ground objects.
更多查看译文
关键词
Remote sensing images,Semantic segmentation,Hierarchical class representation
AI 理解论文
溯源树
样例
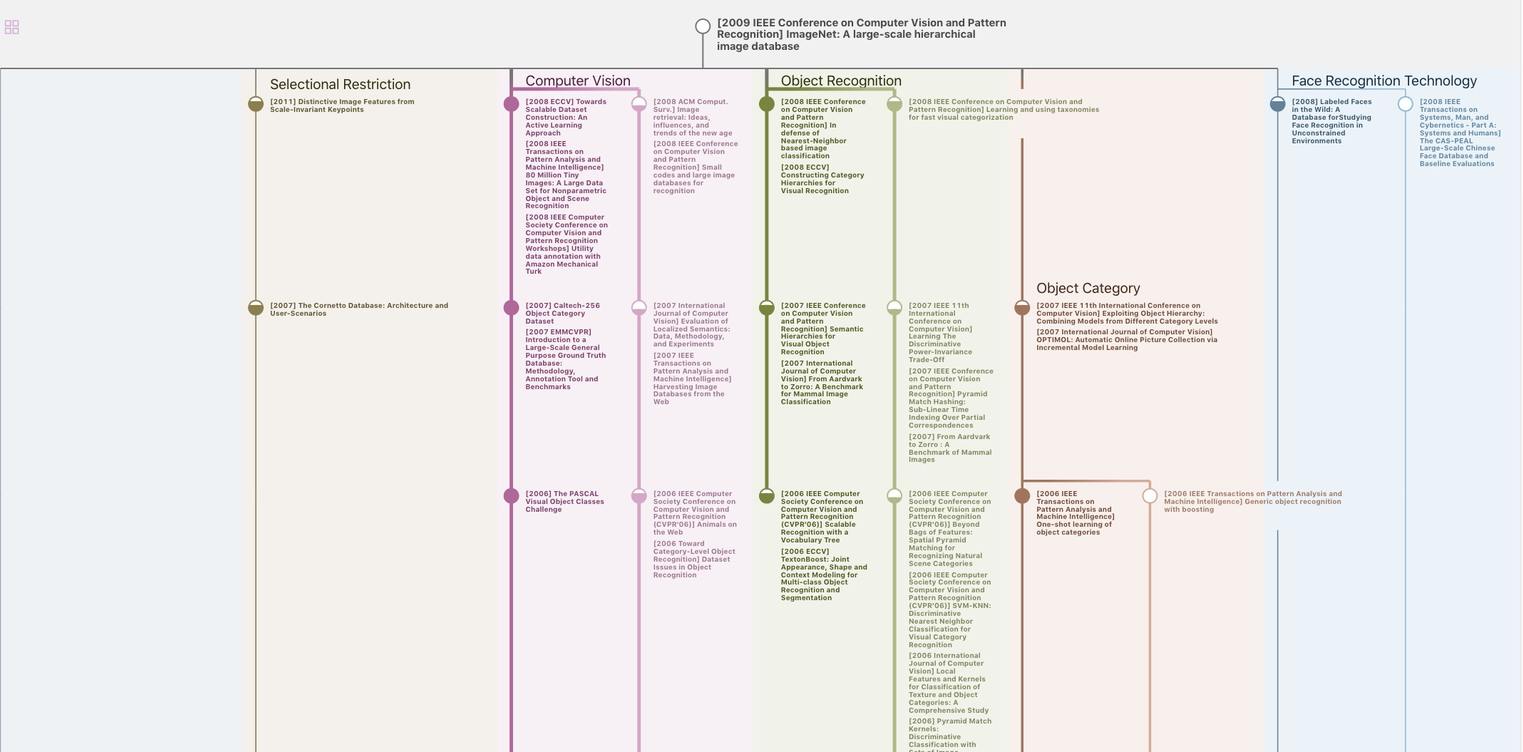
生成溯源树,研究论文发展脉络
Chat Paper
正在生成论文摘要