SA-STT: A Structure-Aware Spatio-Temporal Transformer for Traffic Prediction.
International Conference on Intelligent Systems and Knowledge Engineering(2023)
摘要
Predicting traffic flow is central to alleviating congestion, optimizing routes, and supporting traffic planning and urban management. However, this task remains challenging, particularly given the vast and intricate topologies of urban traffic networks. This paper presents a novel model-Structure Aware Spatio-Temporal Transformer (SASTT)-that leverages graph convolutional networks, attention mechanisms, and traffic transfer embedding to effectively capture complex spatio-temporal dependencies in traffic data. SASTT employs a local information sampling approach before computing global relevance, ensuring a robust representation of traffic propagation features. Furthermore, we develop a traffic transfer embedding method that aligns with the spatial structure of traffic data by incorporating road network information. Experiments conducted on four real-world datasets from different cities and compared with several state-of-the-art baselines show that our model achieves significant improvements in terms of mean absolute error (MAE) and root mean squared error (RMSE), confirming the effectiveness of our method.
更多查看译文
关键词
Intelligent Transportation Systems,Traffic Prediction,Deep Learning,Spatio-Temporal Transformer
AI 理解论文
溯源树
样例
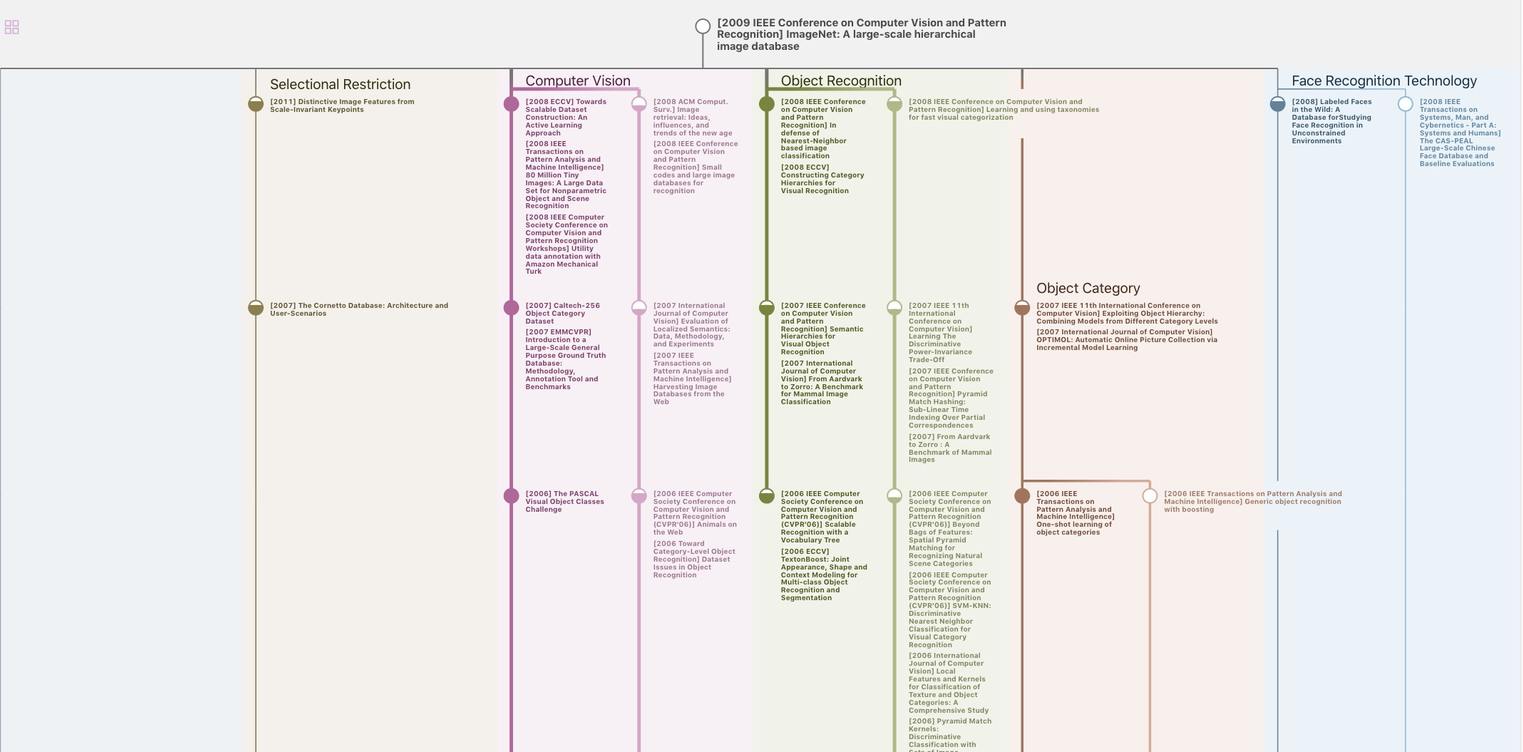
生成溯源树,研究论文发展脉络
Chat Paper
正在生成论文摘要