Distributed Scatterer Interferometry for Fast Decorrelation Scenarios Based on Sparsity Regularization.
IEEE Trans. Geosci. Remote. Sens.(2024)
摘要
How to improve the phase signal-to-noise ratio (SNR) of distributed scatterers (DSs) is a key topic in DS interferometry (DSI). Although some state-of-the-art phase linking (PL) estimators have been proposed, their performance is still limited by the accuracy of the estimated sample covariance matrix (SCM). The key challenges arise from the biased estimation of the near-zero coherence matrix (the magnitude matrix of SCM) under conditions of small sample sizes and heterogeneous samples. To overcome this limitation, we present a sparse regularization-based PL estimator that considers the potential sparsity structure of the inverse covariance matrix. In this new estimator, we first introduced the graphical lasso (GLasso) algorithm into the small samples estimation problem of SCM, which suppresses the biased estimation of the sparse inverse covariance matrix by introducing
L
1
-norm regularization, significantly reducing the impact of weakly coherent interferograms in fast decorrelation scenarios. Furthermore, we also attempt to generalize this scheme to long-term coherence cases through the utilization of
L
2
-norm regularization. Both synthetic data tests and real Sentinel-1 data covering Changi Airport, Singapore, demonstrate the validity of the proposed approach.
更多查看译文
关键词
Phase linking (PL),sparsity regularization,distributed scatterer interferometry (DSI),fast decorrelation
AI 理解论文
溯源树
样例
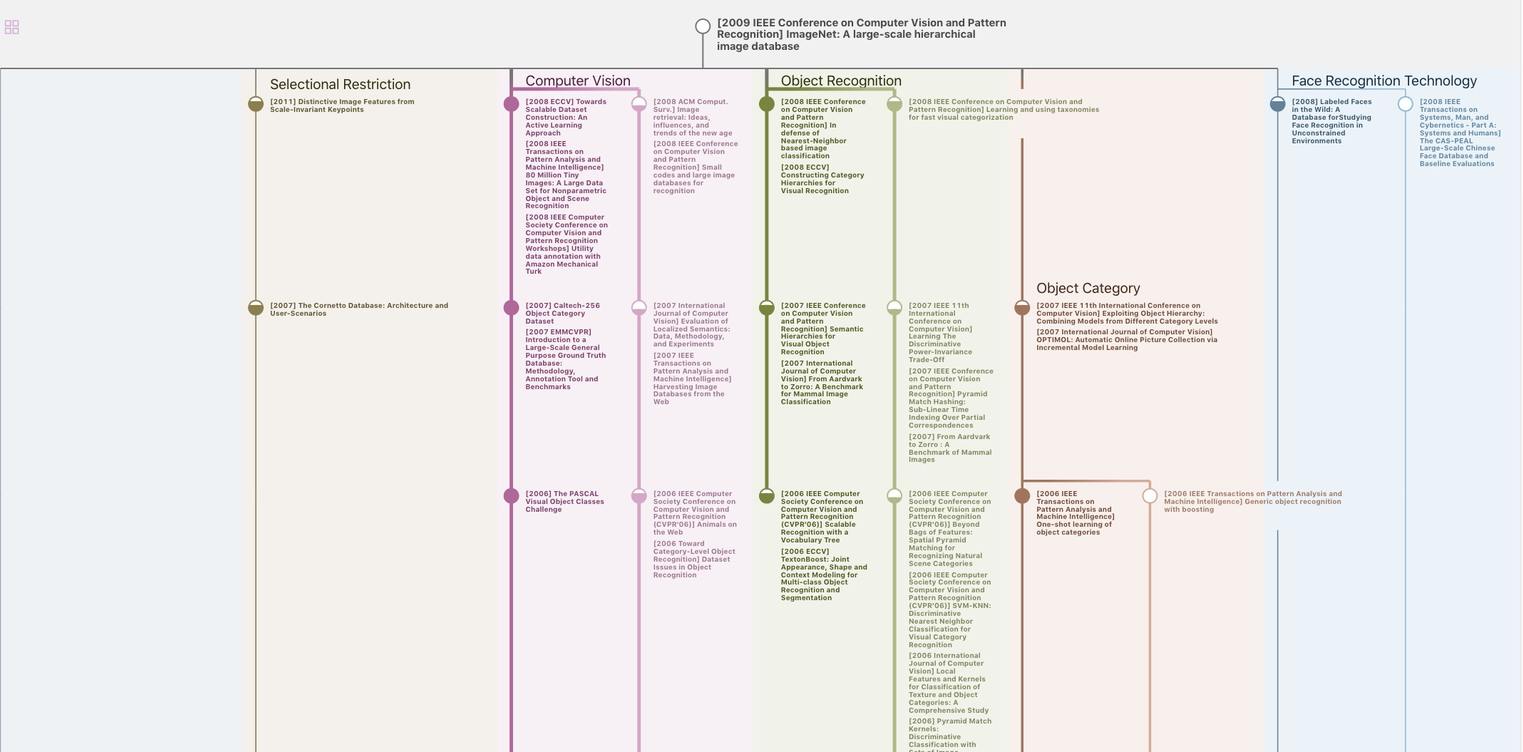
生成溯源树,研究论文发展脉络
Chat Paper
正在生成论文摘要