SoK: Gradient Leakage in Federated Learning
arxiv(2024)
摘要
Federated learning (FL) enables collaborative model training among multiple
clients without raw data exposure. However, recent studies have shown that
clients' private training data can be reconstructed from the gradients they
share in FL, known as gradient inversion attacks (GIAs). While GIAs have
demonstrated effectiveness under ideal settings and auxiliary
assumptions, their actual efficacy against practical FL systems remains
under-explored. To address this gap, we conduct a comprehensive study on GIAs
in this work. We start with a survey of GIAs that establishes a milestone to
trace their evolution and develops a systematization to uncover their inherent
threats. Specifically, we categorize the auxiliary assumptions used by existing
GIAs based on their practical accessibility to potential adversaries. To
facilitate deeper analysis, we highlight the challenges that GIAs face in
practical FL systems from three perspectives: local training,
model, and post-processing. We then perform extensive
theoretical and empirical evaluations of state-of-the-art GIAs across diverse
settings, utilizing eight datasets and thirteen models. Our findings indicate
that GIAs have inherent limitations when reconstructing data under practical
local training settings. Furthermore, their efficacy is sensitive to the
trained model, and even simple post-processing measures applied to gradients
can be effective defenses. Overall, our work provides crucial insights into the
limited effectiveness of GIAs in practical FL systems. By rectifying prior
misconceptions, we hope to inspire more accurate and realistic investigations
on this topic.
更多查看译文
AI 理解论文
溯源树
样例
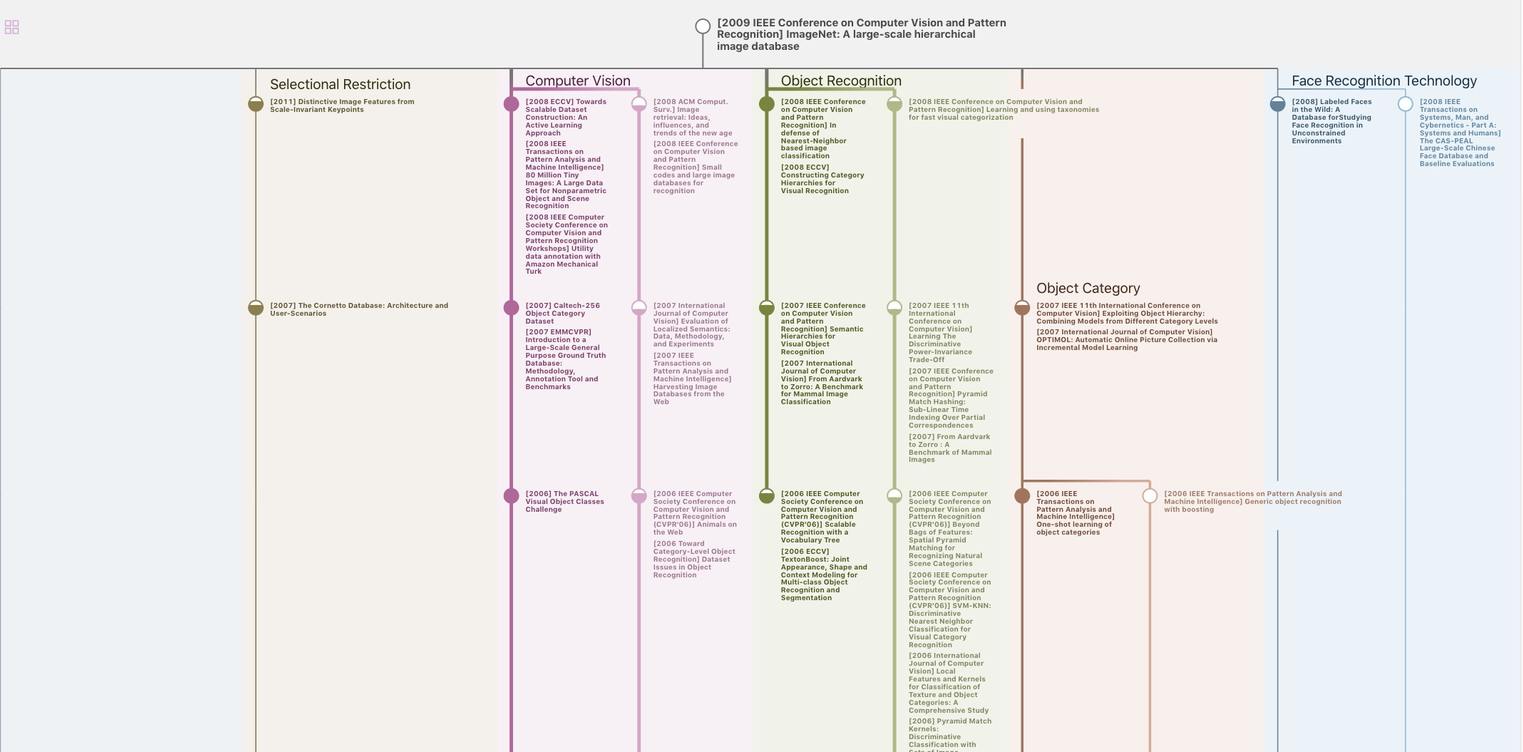
生成溯源树,研究论文发展脉络
Chat Paper
正在生成论文摘要