Intelligent Reflecting Surface Aided Target Localization With Unknown Transceiver-IRS Channel State Information
arxiv(2024)
摘要
Integrating wireless sensing capabilities into base stations (BSs) has become
a widespread trend in the future beyond fifth-generation (B5G)/sixth-generation
(6G) wireless networks. In this paper, we investigate intelligent reflecting
surface (IRS) enabled wireless localization, in which an IRS is deployed to
assist a BS in locating a target in its non-line-of-sight (NLoS) region. In
particular, we consider the case where the BS-IRS channel state information
(CSI) is unknown. Specifically, we first propose a separate BS-IRS channel
estimation scheme in which the BS operates in full-duplex mode (FDM), i.e., a
portion of the BS antennas send downlink pilot signals to the IRS, while the
remaining BS antennas receive the uplink pilot signals reflected by the IRS.
However, we can only obtain an incomplete BS-IRS channel matrix based on our
developed iterative coordinate descent-based channel estimation algorithm due
to the "sign ambiguity issue". Then, we employ the multiple hypotheses testing
framework to perform target localization based on the incomplete estimated
channel, in which the probability of each hypothesis is updated using Bayesian
inference at each cycle. Moreover, we formulate a joint BS transmit waveform
and IRS phase shifts optimization problem to improve the target localization
performance by maximizing the weighted sum distance between each two
hypotheses. However, the objective function is essentially a quartic function
of the IRS phase shift vector, thus motivating us to resort to the
penalty-based method to tackle this challenge. Simulation results validate the
effectiveness of our proposed target localization scheme and show that the
scheme's performance can be further improved by finely designing the BS
transmit waveform and IRS phase shifts intending to maximize the weighted sum
distance between different hypotheses.
更多查看译文
AI 理解论文
溯源树
样例
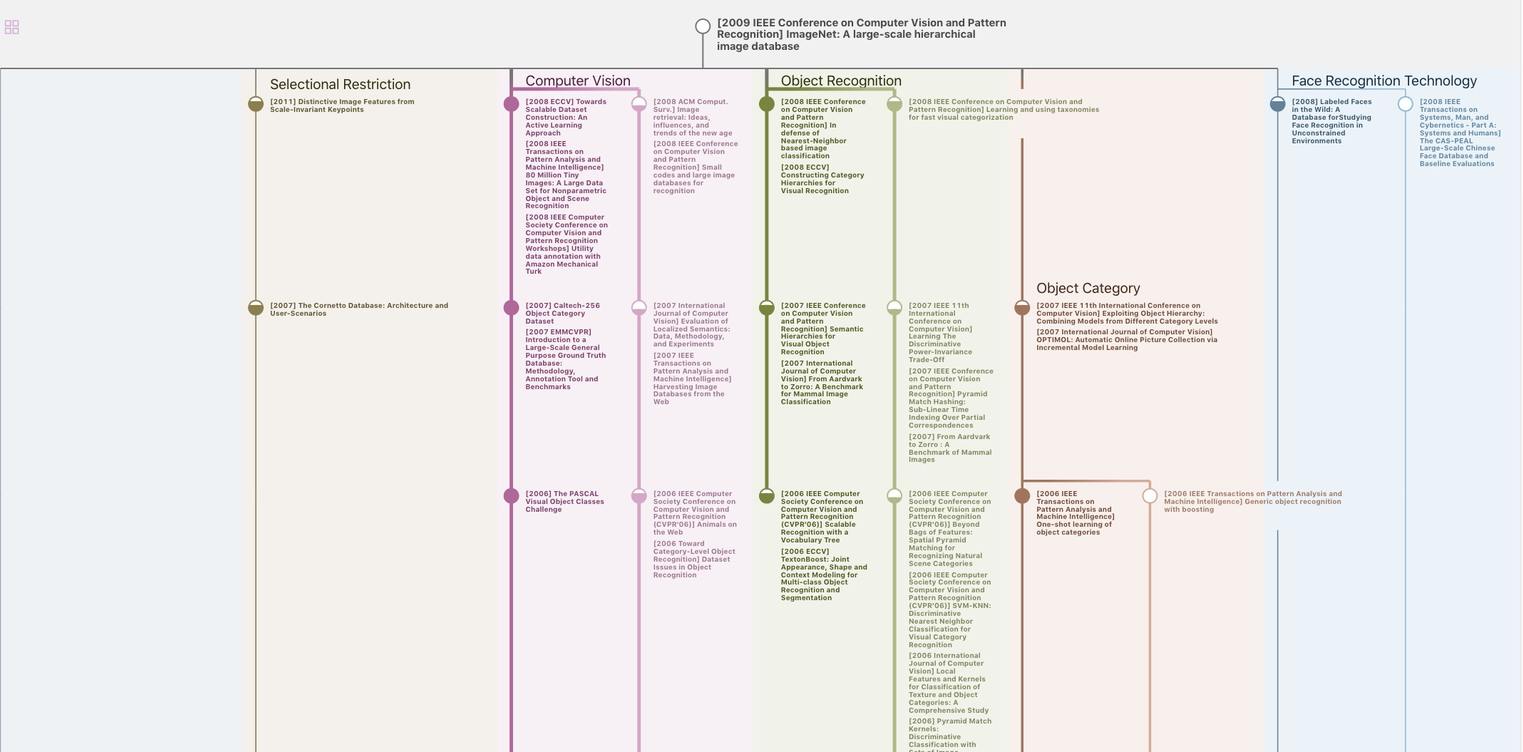
生成溯源树,研究论文发展脉络
Chat Paper
正在生成论文摘要