SMDC-Net: Saliency-Guided Multi-Head Distribution Calibration Network for Few-Shot Object Detection on Remote Sensing Images
IEEE Geoscience and Remote Sensing Letters(2024)
摘要
Object detection on remote sensing images (RSIs) is a critical component of remote sensing image processing techniques. Nonetheless, as the complexity of the model structure increases, more training data is required to prevent a severe decline in object detection performance. This requirement highlights the potential of few-shot object detection techniques. At present, few researches have been investigated for few-shot object detection on RSIs. To address this situation, we propose the saliency-guided multi-head distribution calibration network (SMDC-Net) by building upon the existing fine-tuning-based two-stage few-shot object detection approach. We employ an RSIs salient object detection (SOD) branch that leverages the feature of RSIs to predict the saliency maps. These predicted saliency maps are used as an attention map to augment the feature of RSIs. Meanwhile, to guarantee the ability of the network to identify objects within both the base and novel categories accurately, we design a multi-head detector which can recognize objects within base and novel categories separately while introducing a consistency loss to supervise the consistency of the multi-head predicted labels. We evaluate the performance of two widely recognized RSIs datasets (i.e., DIOR and NWPU VHR-10) within various experimental settings for comparison and ablation analysis. The results illustrate that SMDC-Net enhances the detection performance of novel categories approximately 3% compared to the state-of-the-art MM-RCNN, while preserving the performance of base categories.
更多查看译文
关键词
few-shot object detection,remote sensing images,salient object detection,consistency supervision
AI 理解论文
溯源树
样例
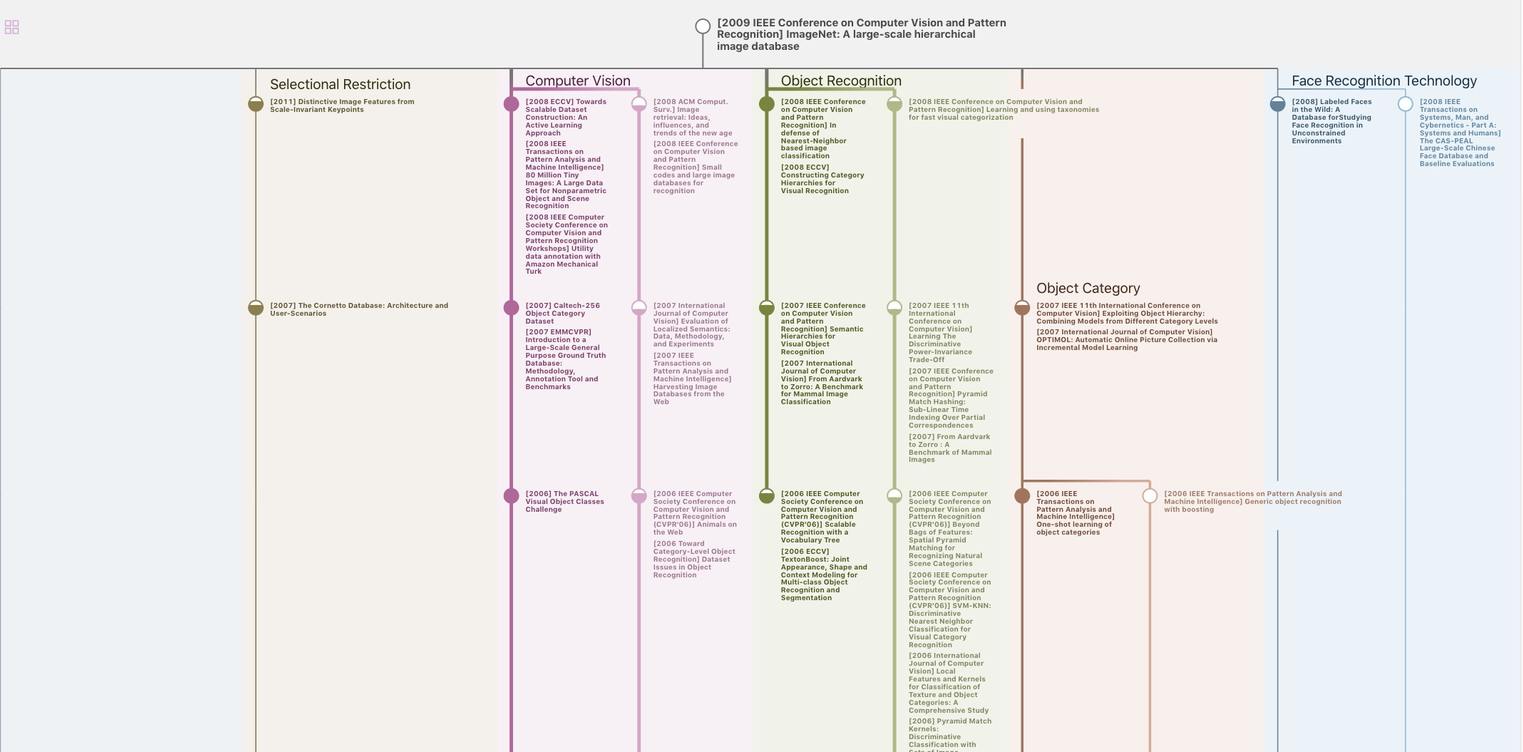
生成溯源树,研究论文发展脉络
Chat Paper
正在生成论文摘要