Improving ionic conductivity of garnet solid-state electrolytes using Gradient boosting regression optimized machine learning
Journal of Power Sources(2024)
摘要
Garnet solid-state electrolytes have become one of the most promising electrolyte materials due to their high ionic conductivity, wide electrochemical window, and excellent electrochemical stability. However, the trial-and-error method used to screen high-performance garnet solid-state electrolytes has the disadvantages of a long development cycle and high cost. Machine learning methods based on big data can be independent of the physical mechanism of the material, and improved the efficiency of material development. In this work, the effect of structural factor (t), first ionization energy, and other feature descriptors on ionic conductivity were studied by using the Gradient boosting regression (GBR), Random Forest (RF), eXtreme Gradient Boosting (XGB), and other models. Machine learning models can improve the accuracy of predicting the ionic conductivity of garnet solid-state electrolytes, and have guided the preparation of five garnet-type solid electrolyte materials, the ionic conductivity of Li6·2La3Zr2Fe0·25O12 is 1.08 × 10−4 S cm−1, while the predicted value is 1.37 × 10−4 S cm−1, the descriptors in the model will provide a reference for other researchers.
更多查看译文
关键词
Solid-state batteries,Ionic conductivity,Machine learning,Garnet electrolytes,GBR optimization
AI 理解论文
溯源树
样例
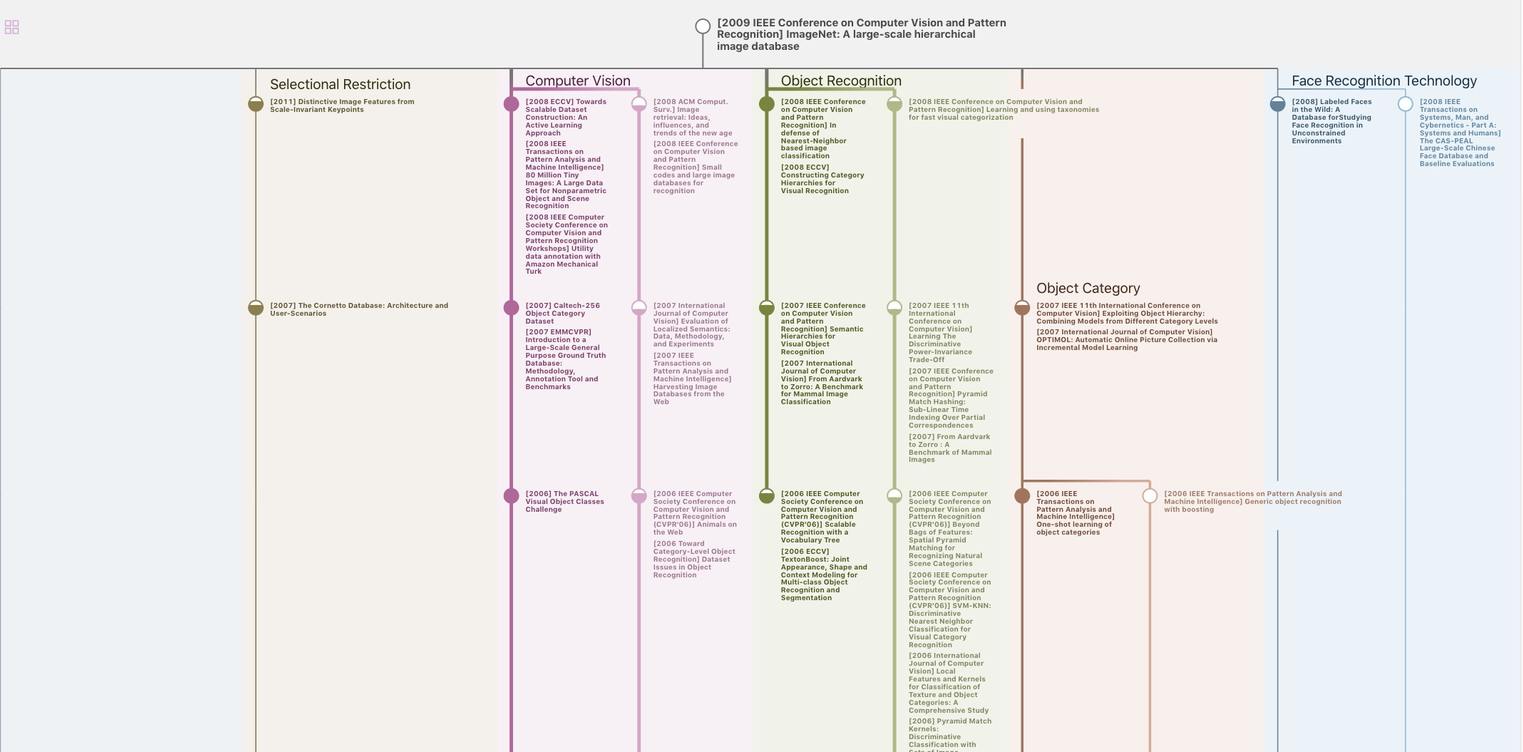
生成溯源树,研究论文发展脉络
Chat Paper
正在生成论文摘要