EME: Energy-Based Multiexpert Model for Long-Tailed Remote Sensing Image Classification
IEEE TRANSACTIONS ON GEOSCIENCE AND REMOTE SENSING(2024)
摘要
The distribution of remote sensing scene images often follows a long-tailed pattern, where there is an abundance of samples in a few dominant classes and a scarcity of samples in most other classes. This presents two major challenges when it comes to identifying this type of data: Head-Dominance: Models trained on such data tend to prioritize the dominant classes, overlooking the tail classes and resulting in poor performance when it comes to recognizing them. Tail-Interference: The presence of tail classes disrupts the learned representations for the head classes, acting as noise that negatively impacts the recognition accuracy of the head data. To address these challenges, we propose an innovative solution called the energy-based multiexpert (EME) model. The core concept behind this approach is to utilize energy-based discriminators (EDors) to separate the data into head and tail categories. Subsequently, we design multiple experts to classify the head and tail data separately, ensuring that the significant differences in data volume between these categories do not interfere with each other. Experimental results obtained by applying the EME model to three remote sensing datasets demonstrate its efficiency, outperforming current state-of-the-art (SOTA) methods. These findings underscore the effectiveness of our proposed approach in addressing the challenges posed by the long-tailed distribution in remote sensing scene images.
更多查看译文
关键词
Energy-based discriminators (EDors),head-dominance,long-tailed distribution,remote sensing image classification,tail-interference
AI 理解论文
溯源树
样例
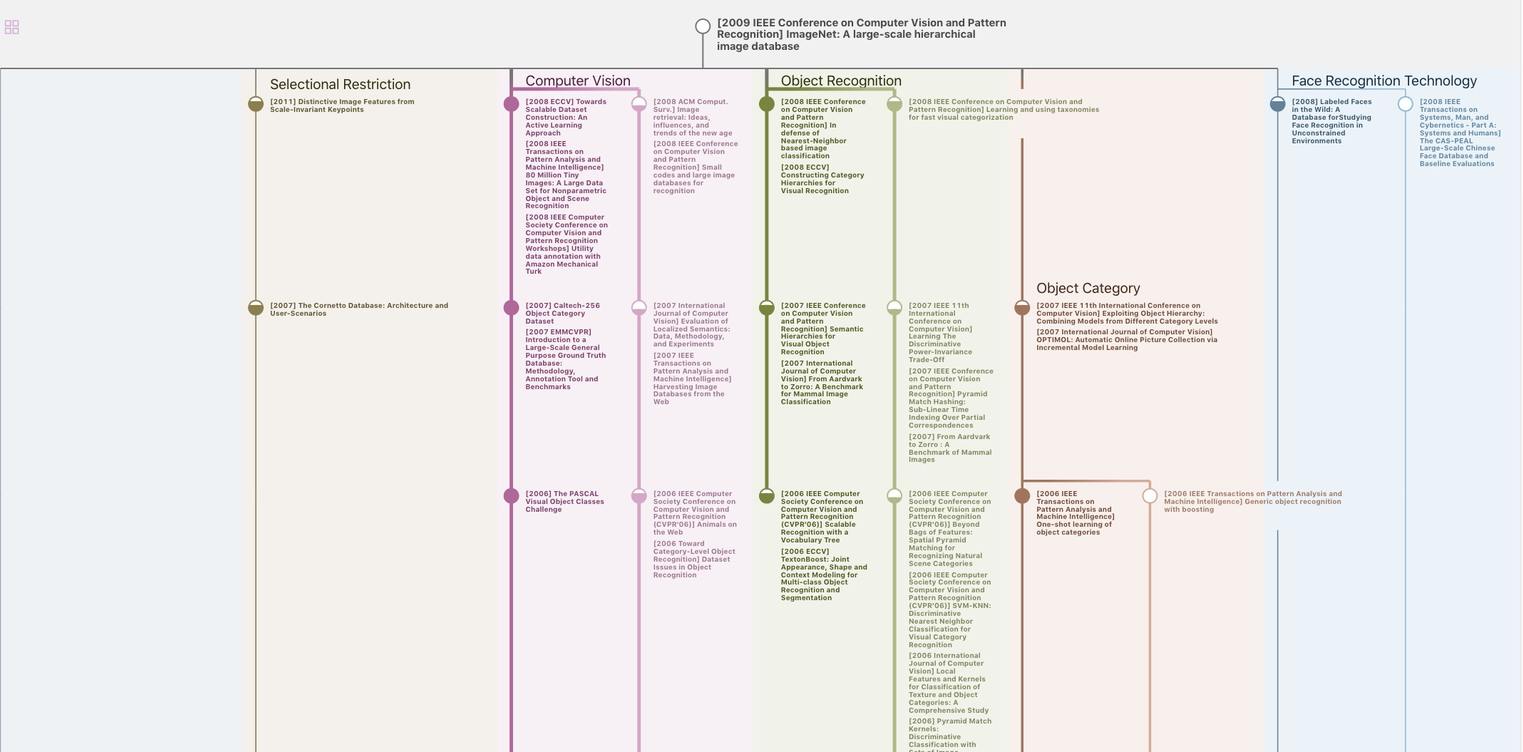
生成溯源树,研究论文发展脉络
Chat Paper
正在生成论文摘要