Improving Factual Accuracy of Neural Table-to-Text Output by Addressing Input Problems in ToTTo
arxiv(2024)
摘要
Neural Table-to-Text models tend to hallucinate, producing texts that contain
factual errors. We investigate whether such errors in the output can be traced
back to problems with the input. We manually annotated 1,837 texts generated by
multiple models in the politics domain of the ToTTo dataset. We identify the
input problems that are responsible for many output errors and show that fixing
these inputs reduces factual errors by between 52
model). In addition, we observe that models struggle in processing tabular
inputs that are structured in a non-standard way, particularly when the input
lacks distinct row and column values or when the column headers are not
correctly mapped to corresponding values.
更多查看译文
AI 理解论文
溯源树
样例
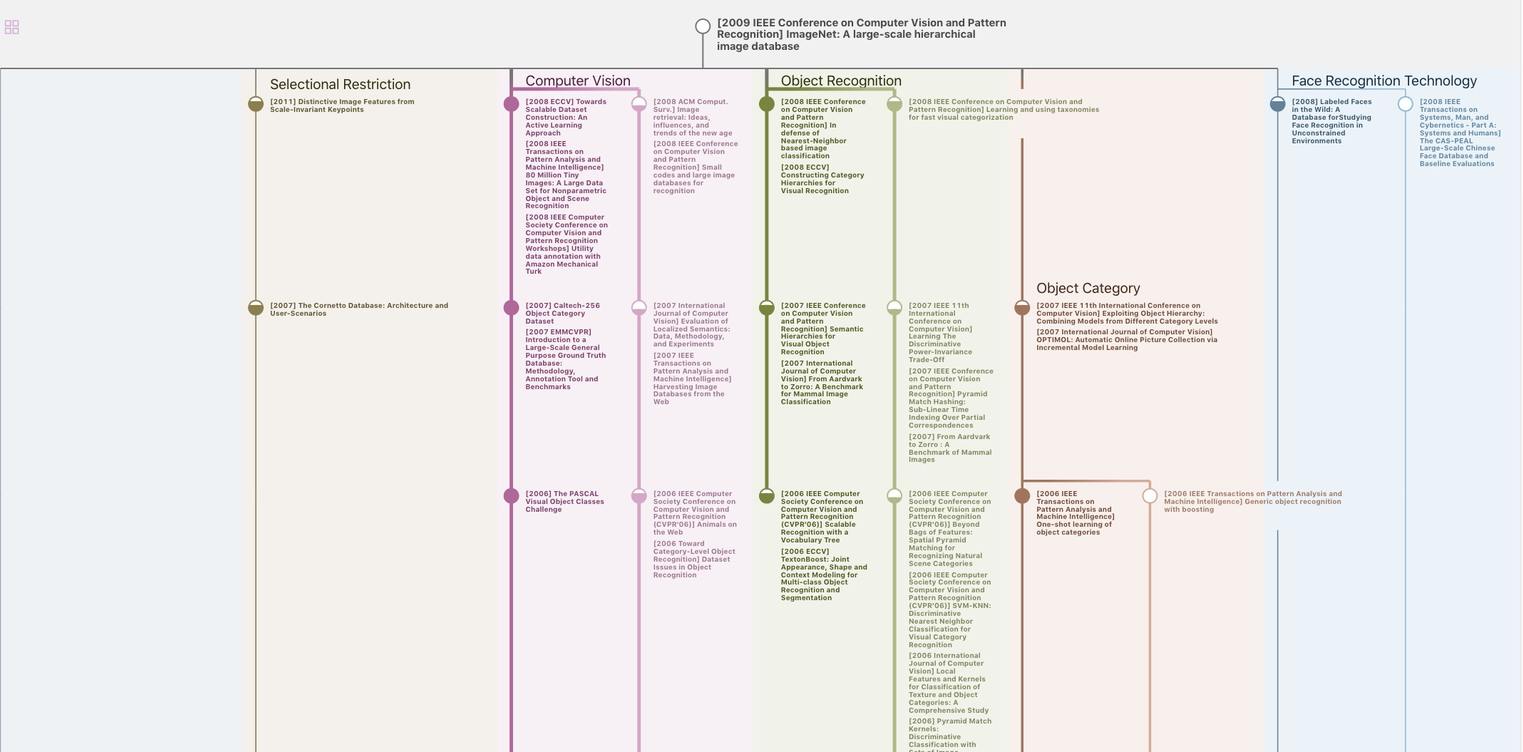
生成溯源树,研究论文发展脉络
Chat Paper
正在生成论文摘要