Improvement of Performance in Freezing of Gait detection in Parkinsons Disease using Transformer networks and a single waist worn triaxial accelerometer
arxiv(2024)
摘要
Freezing of gait (FOG) is one of the most incapacitating symptoms in
Parkinsons disease, affecting more than 50 percent of patients in advanced
stages of the disease. The presence of FOG may lead to falls and a loss of
independence with a consequent reduction in the quality of life. Wearable
technology and artificial intelligence have been used for automatic FOG
detection to optimize monitoring. However, differences between laboratory and
daily-life conditions present challenges for the implementation of reliable
detection systems. Consequently, improvement of FOG detection methods remains
important to provide accurate monitoring mechanisms intended for free-living
and real-time use. This paper presents advances in automatic FOG detection
using a single body-worn triaxial accelerometer and a novel classification
algorithm based on Transformers and convolutional networks. This study was
performed with data from 21 patients who manifested FOG episodes while
performing activities of daily living in a home setting. Results indicate that
the proposed FOG-Transformer can bring a significant improvement in FOG
detection using leave-one-subject-out cross-validation (LOSO CV). These results
bring opportunities for the implementation of accurate monitoring systems for
use in ambulatory or home settings.
更多查看译文
AI 理解论文
溯源树
样例
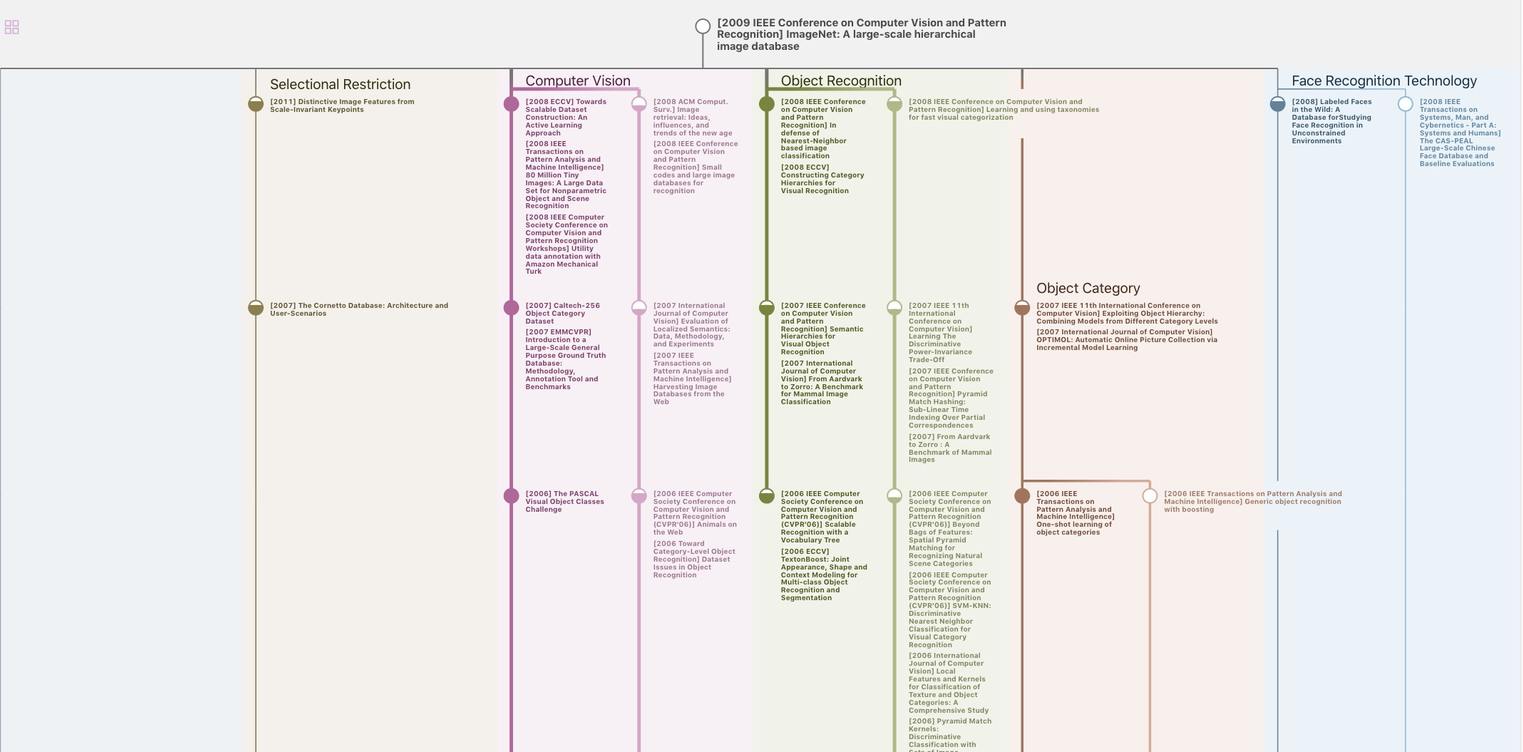
生成溯源树,研究论文发展脉络
Chat Paper
正在生成论文摘要