GAP: A Grammar and Position-Aware Framework for Efficient Recognition of Multi-Line Mathematical Formulas
PROCEEDINGS OF THE 17TH ACM INTERNATIONAL CONFERENCE ON WEB SEARCH AND DATA MINING, WSDM 2024(2024)
摘要
Formula recognition endeavors to automatically identify mathematical formulas from images. Currently, the Encoder-Decoder model has significantly advanced the translation from image to corresponding formula markups. Nonetheless, previous research primarily concentrated on single-line formula recognition, ignoring the recognition of multi-line formulas, which presents additional challenges such as more stringent grammatical restrictions and twodimensional positions. In this work, we present GAP (Grammar And Position-Aware formula recognition), a comprehensive framework designed to tackle the challenges in multi-line mathematical formula recognition. First, to overcome the limitations imposed by grammar, we design a novel Grammar Aware Contrastive Learning (GACL) module, integrating complex grammar rules into the transcription model through a contrastive learning mechanism. Furthermore, primitive contrastive learning lacks clear directions for comprehending grammar rules and can lead to unstable convergence or prolonged training cycles. To enhance training efficiency, we propose Rank-Based Sampling (RBS) specialized for multi-line formulas, which guides the learning process by the importance ranking of different grammar errors. Finally, spatial location information is critical considering the two-dimensional nature of multiline formulas. To aid the model in keeping track of that global information, we introduced a Visual Coverage (VC) mechanism that incorporates historical attention information into the image features via a parameter-free way. To validate the effectiveness of our GAP framework, we construct a new dataset Multi-Line containing 12,002 multi-line formulas and conduct extensive experiments to show the efficacy of our GAP framework in capturing grammatical rules, enhancing recognition accuracy, and enhancing training efficiency. Codes and datasets are available at https://github.com/Sinon02/GAP.
更多查看译文
关键词
Formula Recognition,Contrastive Learning,Encoder-Decoder
AI 理解论文
溯源树
样例
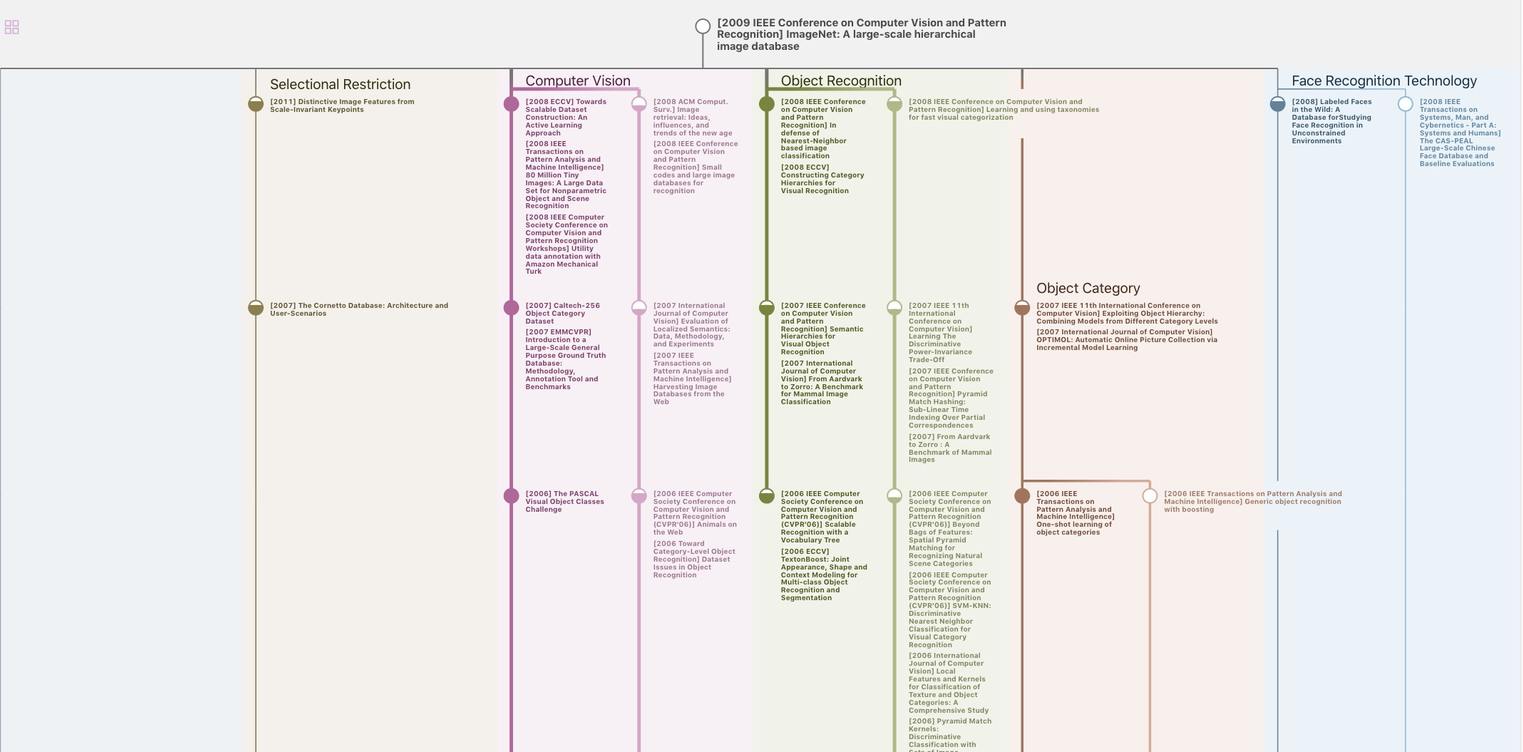
生成溯源树,研究论文发展脉络
Chat Paper
正在生成论文摘要