External validation of a multivariable prediction model for positive resection margins in breast-conserving surgery
crossref(2024)
摘要
Abstract Background: Positive resection margins after breast-conserving surgery (BCS) most often demands a repeat surgery. Identifying patients at risk of positive margins allow for surgical techniques using wider resection margins, which may reduce the need of additional surgeries. A prediction model by Ellbrant et al. with seven preoperative clinical-pathological and imaging variables predicts positive margins after BCS with an area under the ROC curve (AUC) of 0.80. The aim of the current study was to externally validate this prediction model and to explore if additional available preoperative variables can further improve the accuracy of the model. Methods: This retrospective cohort study comprises 225 invasive breast cancer patients who underwent BCS and included at Aarhus University Hospital, Aarhus, Denmark during 2020-2022. Receiver operating characteristic (ROC) and calibration analysis were used to validate the multivariable prediction model. Univariable logistic regression was used to evaluate if additional variables available in the validation cohort were associated with positive margins and backward elimination to explore if these variables could further improve the model´s predictive accuracy. Results: The AUC of the model was 0.60 (95% CI: 0.50-0.70) in the validation cohort. High versus low mammographic breast density was associated with positive margins (p=0.009), however the AUC did not further increase, when adding this as an additional variable to the model. Conclusion: The accuracy of the model to predict positive margins after BCS in the validation cohort was lower as compared to in the development cohort, most likely due to differences in patient and tumor characteristics between the sites. High mammographic breast density was found to be associated with positive margins after BCS but did not further improve the model´s predictive accuracy.
更多查看译文
AI 理解论文
溯源树
样例
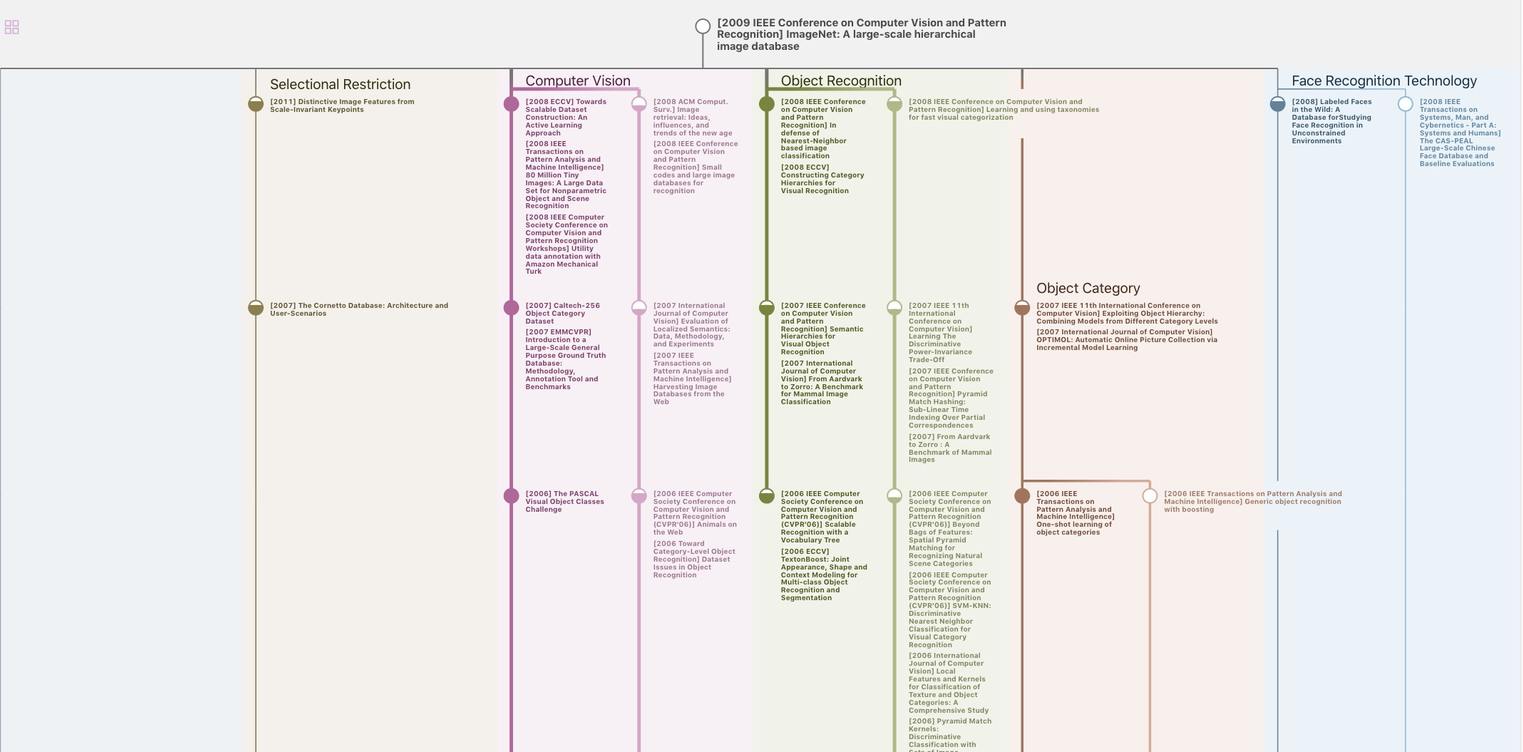
生成溯源树,研究论文发展脉络
Chat Paper
正在生成论文摘要