Predictive Control of Diesel Oxidation Catalysts with Federated Learning in Connected Vehicles
2024 Forum for Innovative Sustainable Transportation Systems (FISTS)(2024)
摘要
In various industries, diesel engines continue to be indispensable due to their exceptional fuel efficiency and torque characteristics, all while meeting stringent emissions standards through advanced aftertreatment technologies like Diesel Oxidation Catalysts (DOC), Diesel Particulate Filters (DPF), and Selective Catalytic Reduction (SCR). The critical role of hydrocarbon dosing in Diesel Oxidation Catalysts (DOC) lies in effectively reducing emissions such as hydrocarbons, carbon monoxide, and soluble organic fractions in particulate matter, converting them into H
2
O and CO
2
. However, traditional DOC control strategies often struggle to adapt to the dynamic nature of diesel engine operation, resulting in temperature overshoots during transient engine conditions. To address this challenge, an approach is proposed that utilizes Long Short-Term Memory (LSTM) networks within a Federated Learning (FL) framework to predict and control DOC temperature overshoots in realtime, enabling adjustments to the hydrocarbon dosing strategy. This approach not only aims to minimize overshoots but also extends the lifespan of aftertreatment components and reduces emissions. Moreover, when applied to connected vehicles, Federated Learning ensures rigorous data privacy by conducting model training locally within each vehicle, preserving the confidentiality of sensitive information while fostering collaborative improvements in emissions control.
更多查看译文
关键词
Predictive Control,Federated Learning,Diesel Oxidation Catalyst,Bicarbonate,Control Strategy,Short-term Memory,Data Privacy,Learning Framework,Long Short-term Memory,Long Memory,Memory Network,Long Short-term Memory Network,Short-term Memory Network,Diesel Engine,Diesel Exhaust Particles,Selective Catalytic Reduction,Prediction Model,Training Set,Validation Set,Machine Learning Models,Constant Learning Rate,Data Security,Long Short-term Memory Model,Temperature Prediction,Global Model,Individual Vehicles,Communication Rounds,Model Predictive Control,Collaborative Model,Stochastic Gradient Descent
AI 理解论文
溯源树
样例
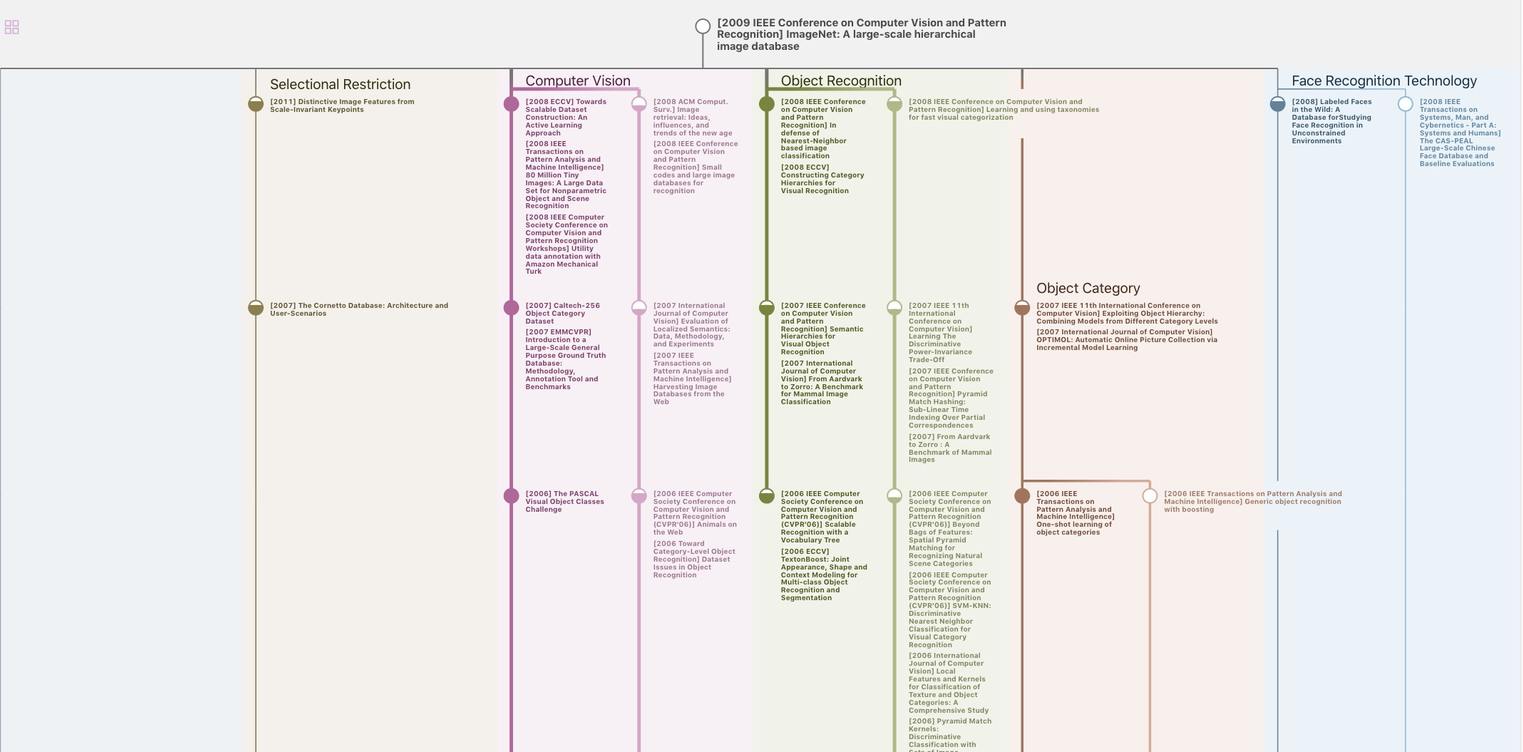
生成溯源树,研究论文发展脉络
Chat Paper
正在生成论文摘要