Predicting Phosphoglycerylation with Transformer Features and Deep Learning
2023 IEEE Asia-Pacific Conference on Computer Science and Data Engineering (CSDE)(2023)
摘要
Understanding protein sequences can advance treatments for various diseases. However experimentally obtaining this information is laborious, time-consuming, and expensive. Traditional machine learning techniques, like support vector machine, random forest and logistic regression, offer potential to fast-track this process but are sometimes limited by data complexity. Deep learning algorithms, in contrast, tend to yield higher performance. In this study, we employed a convolutional neural network to predict protein phosphoglycerylation. Features were extracted from pre-trained transformer models and compared with conventional features, such as evolutionary information and physicochemical/biochemical properties. Our results indicate significant performance improvements across all feature types, with the combination of transformerbased features and the convolutional neural network being especially effective. This methodology holds potential for other protein property prediction tasks. Our software and datasets used in this study are publicly available at https://github.com/abelavit/DL-PhosphoglycerylationPrediction.git.
更多查看译文
关键词
machine learning,deep learning,transformer network,convolutional neural network,proteomics,phosphoglycerylation
AI 理解论文
溯源树
样例
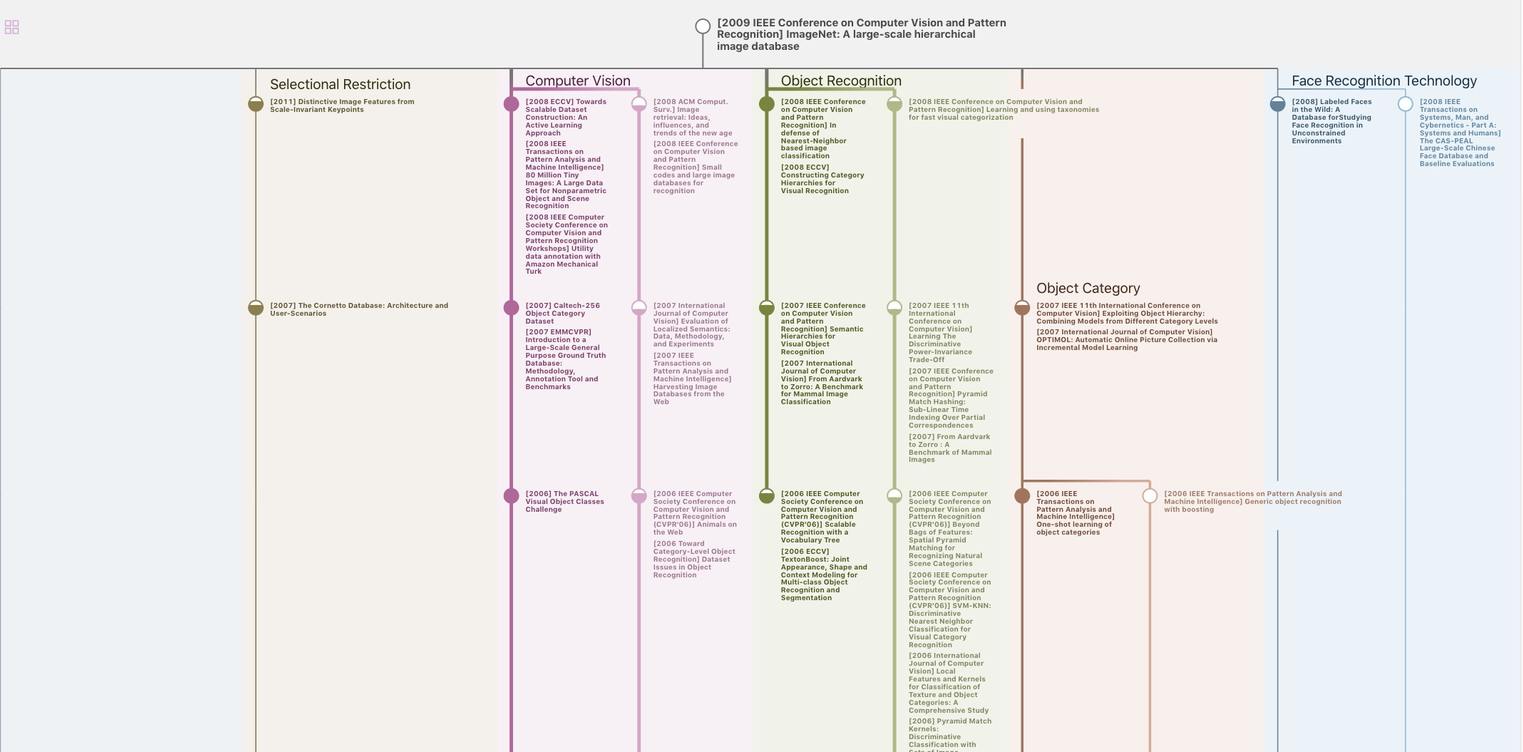
生成溯源树,研究论文发展脉络
Chat Paper
正在生成论文摘要