Integrating optimized item selection with active learning for continuous exploration in recommender systems
Annals of Mathematics and Artificial Intelligence(2024)
摘要
Recommender Systems have become the backbone of personalized services that provide tailored experiences to individual users, yet designing new recommendation applications with limited or no available training data remains a challenge. To address this issue, we focus on selecting the universe of items for experimentation in recommender systems by leveraging a recently introduced combinatorial problem. On the one hand, selecting a large set of items is desirable to increase the diversity of items. On the other hand, a smaller set of items enables rapid experimentation and minimizes the time and the amount of data required to train machine learning models. We first present how to optimize for such conflicting criteria using a multi-level optimization framework. Then, we shift our focus to the operational setting of a recommender system. In practice, to work effectively in a dynamic environment where new items are introduced to the system, we need to explore users’ behaviors and interests continuously. To that end, we show how to integrate the item selection approach with active learning to guide randomized exploration in an ongoing fashion. Our hybrid approach combines techniques from discrete optimization, unsupervised clustering, and latent text embeddings. Experimental results on well-known movie and book recommendation benchmarks demonstrate the benefits of optimized item selection and efficient exploration.
更多查看译文
关键词
Recommender systems,Exploration-exploitation,Active learning,68T01
AI 理解论文
溯源树
样例
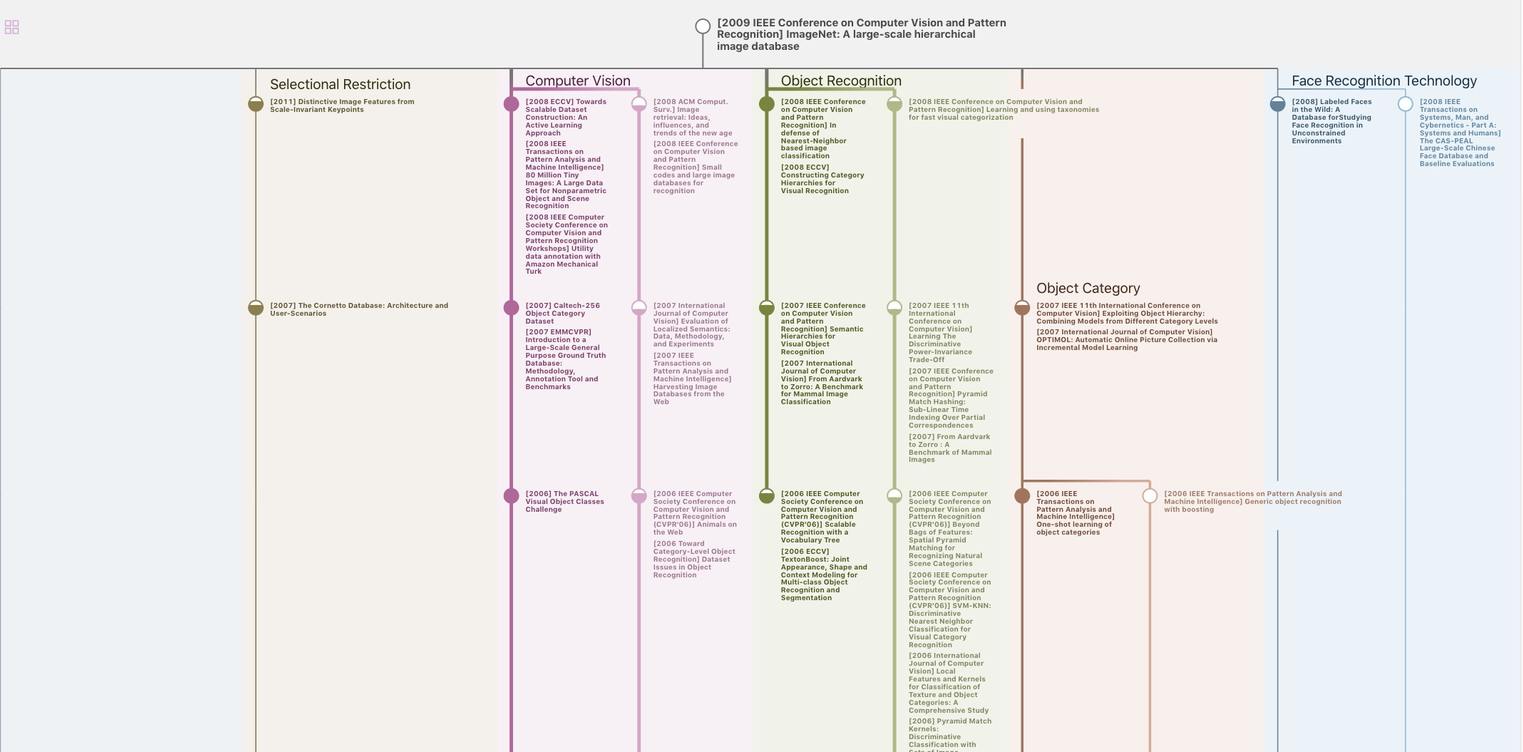
生成溯源树,研究论文发展脉络
Chat Paper
正在生成论文摘要