An Attention Mechanism and Adaptive Accuracy Triple-Dependent MADDPG Formation Control Method for Hybrid UAVs
IEEE TRANSACTIONS ON INTELLIGENT TRANSPORTATION SYSTEMS(2024)
摘要
With the further development of Unmanned Aerial Vehicle (UAV) technologies, research on multi-UAV formations have also received more attention. Unmanned Aerial Vehicles (UAVs) cooperate with each other to form a formation group, which can give full play to the advantages that a single UAV does not have, and more capable of working in multi-task scenarios. Based on the Sierpinski fractal structure, this paper proposes a hybrid formation control architecture. To address the aggregation problem of UAV swarms, a multi-agent deep reinforcement learning (MADRL) method is used for aggregation control. To improve the efficiency, accuracy, effectiveness and scalability of MADRL in environments with a large number of UAVs, a multi-intelligent deep deterministic policy gradient method based on attention mechanism and adaptive accuracy (3A-MADDPG) is proposed. The method enables each agent to selectively focus on the information of other agents, with adaptive learning rate to dynamically learn its own critic network. The algorithm has a large learning rate in the early stage and converges quickly. The later stage of the algorithm has small learning rate and high accuracy, and combined with the fixed variable hybrid reward function designed in the paper, so the UAV can fly to the center of the expected region more accurately, making the whole cluster more accurate. Experiments show that the 3A algorithm proposed in the paper converges faster and achieves higher accuracy than the benchmark algorithm, whether it is the cluster aggregation formation or the separation and aggregation of sub-formations.
更多查看译文
关键词
UAV swarm,Sierpinski fractal structure,multi-agent deep reinforcement learning,attention mechanism,adaptive learning rate
AI 理解论文
溯源树
样例
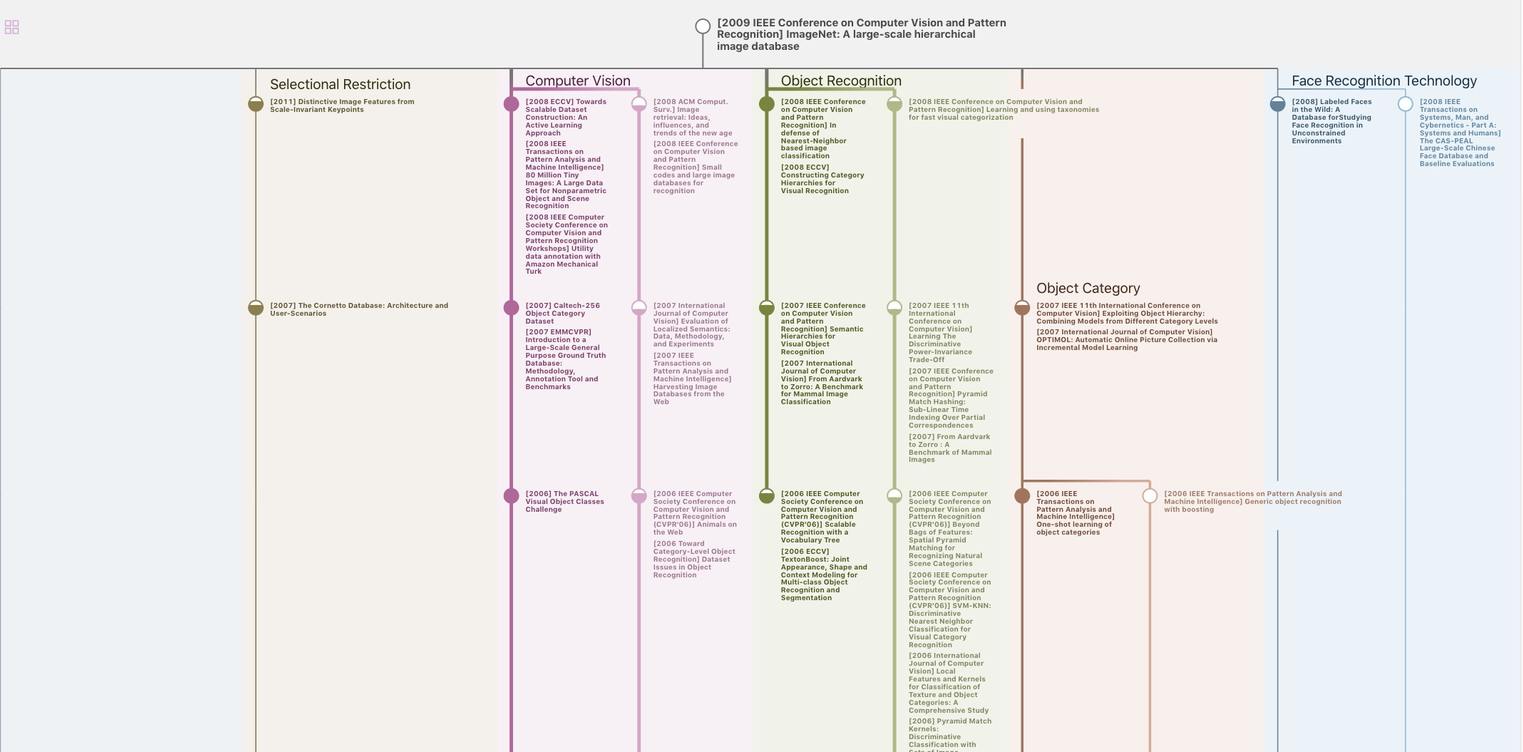
生成溯源树,研究论文发展脉络
Chat Paper
正在生成论文摘要