An Empirical-informed Model for the Early Degradation Trajectory Prediction of Lithium-ion Battery
IEEE Transactions on Energy Conversion(2024)
摘要
Early prediction of the lithium-ion (Li-ion) battery degradation trajectory is of great importance to arrange the maintenance of battery energy storage systems (BESSs). Although extensive data driven methods have achieved a super good performance in state of health (SOH) and remaining useful life (RUL) prediction, the nonlinear characteristics of the Li-ion battery degradation trajectory still prevent an accurate prediction once very limited cycling data known in advance. To solve this issue, this paper proposes an empirical-informed model for the degradation trajectory prediction with only few data from the Li-ion battery's early cycling stage, which integrates the experience based knowledge to train the data driven model. A novel experience based model is proposed to describe the battery degradation curve, which further guides the training procedure of the long-short term memory (LSTM) network. In addition, XGBoost is selected to use a perceptually important point (PIP) based feature for providing the reference capacities. In this way, the proposed method can implement an end-to-end early prediction of the battery trajectory using only partial charging voltage as the input. The performance of the proposed method is verified on three datasets.
更多查看译文
关键词
Battery degradation trajectory,early prediction,empirical-inform,transferable model
AI 理解论文
溯源树
样例
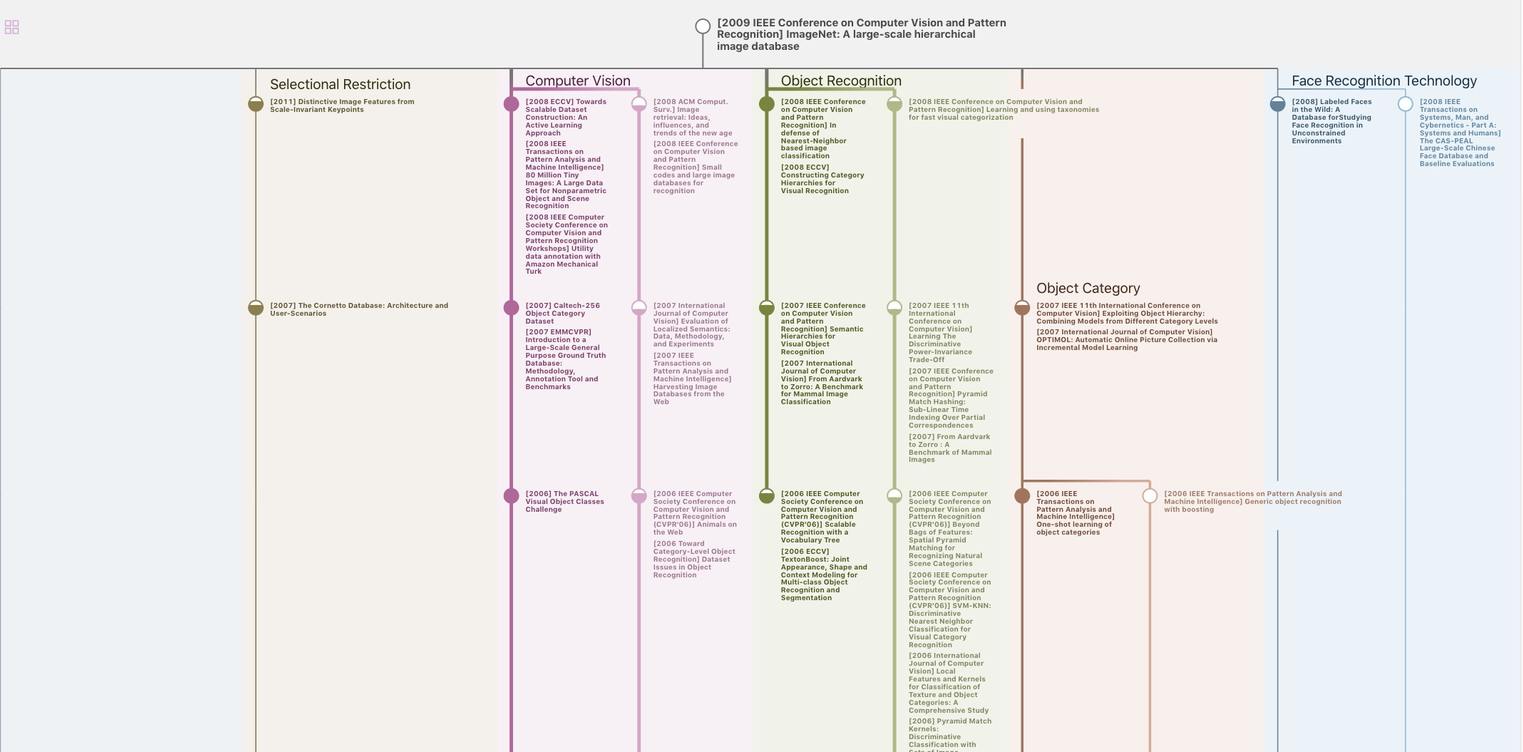
生成溯源树,研究论文发展脉络
Chat Paper
正在生成论文摘要