Machine-learning methods for detecting tuberculosis in Ziehl-Neelsen stained slides: A systematic literature review
Intelligent Systems with Applications(2024)
摘要
Tuberculosis (TB) remains a global health threat, and rapid, automated and accurate diagnosis is crucial for effective control. The tedious and subjective nature of Ziehl-Neelsen (ZN) stained smear microscopy for identifying Mycobacterium tuberculosis (MTB) motivates the exploration of alternative approaches. In recent years, machine learning (ML) methods have emerged as promising tools for automated TB detection in ZN-stained images. This systematic literature review (SLR) comprehensively examines the application of ML methods for TB detection between 2017 and 2023, focusing on their performance metrics and employed dataset characteristics. The study identifies advancements, establishes the state of the art, and pinpoints areas for future research and development in this domain. It sheds light on the discussion about the readiness of machine-learning methods to be confidently, reliably and cost-effectively used to automate the process of tuberculosis detection in ZN slides, being it significant for the health systems worldwide.Following established SLR guidelines, we defined research questions, retrieved 175 papers from 7 well-known sources, and discarded those not complying with the inclusion criteria. Data extraction and analysis were performed on the resulting 65 papers to address our research questions. The key contributions of this review are as follows. First, it presents a characterization of the state of the art of ML methods for ZN-stained TB detection, especially in sputum and tissue. Second, it analyzes top-performing methods and pre-processing techniques. Finally, it pinpoints key research gaps and opportunities.
更多查看译文
关键词
Tuberculosis,Digital pathology,Medical image processing,Artificial intelligence,Computer vision,Machine learning
AI 理解论文
溯源树
样例
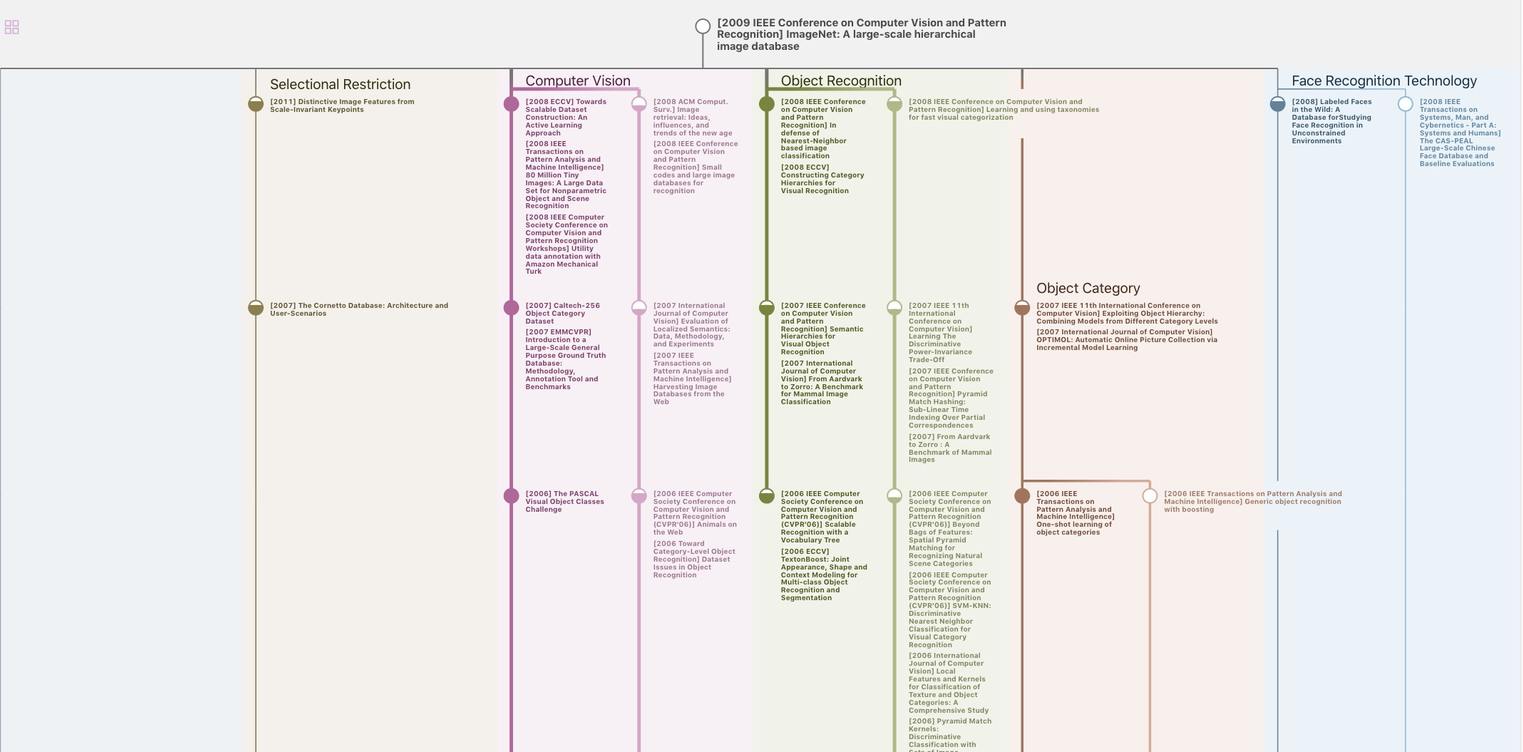
生成溯源树,研究论文发展脉络
Chat Paper
正在生成论文摘要