A lightweight network for abdominal multi-organ segmentation based on multi-scale context fusion and dual self-attention
Information Fusion(2024)
摘要
Segmenting the organs from abdominal CT images is a vital procedure for computer-aided diagnosis and treatment. Accurate and simultaneous segmentation of multiple abdominal organs remains challenging due to the complex structures, varying sizes, and fuzzy boundaries. Currently, most methods aiming at improving segmentation accuracy involve either deepening the network or employing large-scale models, which results in a heavy computation burden and a huge number of model parameters. It is difficult to deploy these methods in a medical environment. In this paper, we present a lightweight network based on multi-scale context fusion and dual self-attention. The dual self-attention mechanism is used to obtain target organ responses from channel domain, while also strengthening the correlation of global information from spatial domain. Considering the complex structure of abdominal organs, we design a multi-scale context fusion module comprised of a pyramid pooling (PP) and an anisotropic strip pooling (ASP). The PP is used to acquire rich local features by aggregating context information from different receptive fields, while the ASP is proposed to extract strip features in different directions to help the network establish long-distance dependencies and capture the characteristics of elongated organs, such as pancreas and spleen. Moreover, a residual module is designed in the skip connection to learn features related to edges and small objects. The proposed method achieves averaged Dice of 90.1% and 82.5% on the FLARE and BTCV datasets, respectively, with only 6.25M model parameters and 21.40G FLOPs, outperforming many state-of-the-art methods.
更多查看译文
关键词
CT image,Segmentation,Context-aware,Feature fusion,Self-attention
AI 理解论文
溯源树
样例
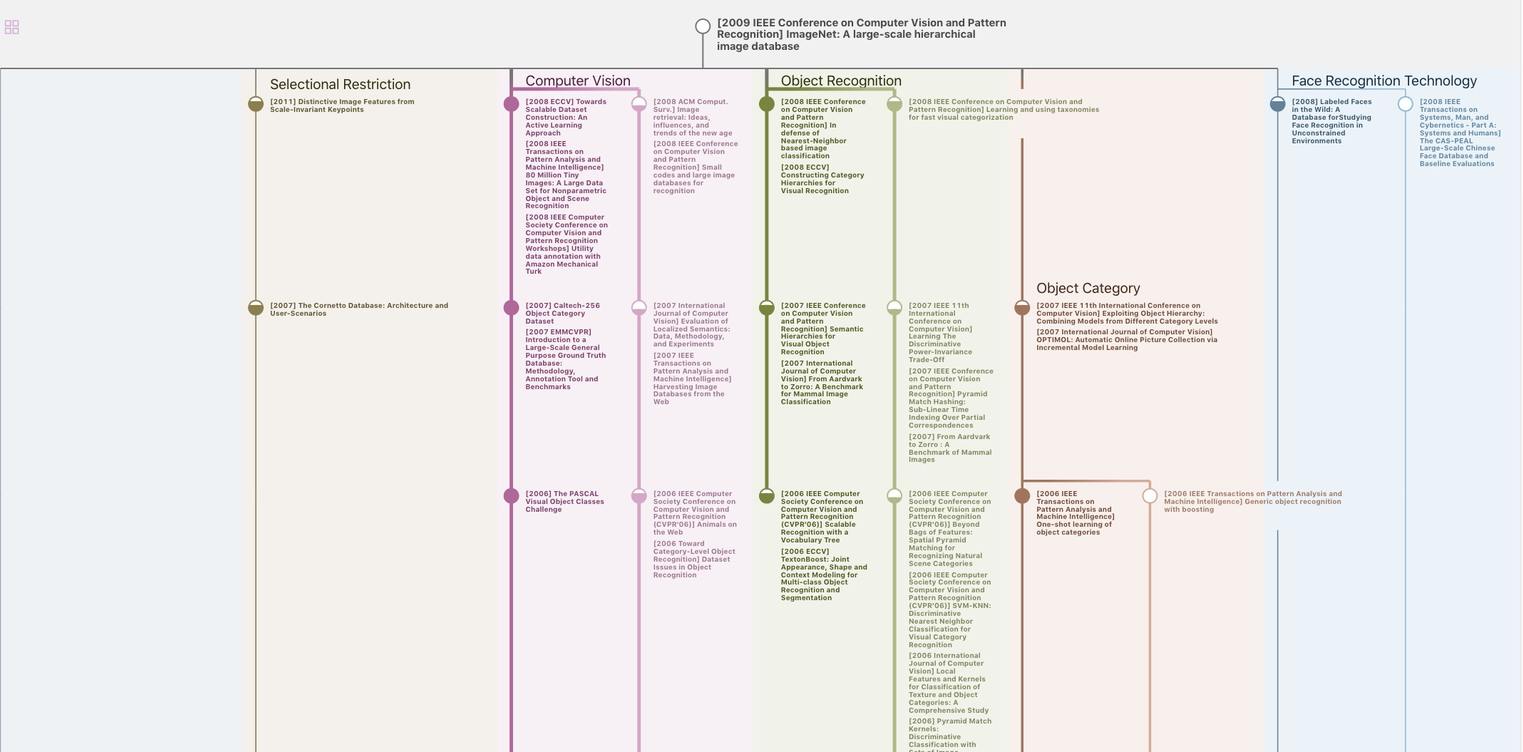
生成溯源树,研究论文发展脉络
Chat Paper
正在生成论文摘要