Lightweight boundary-assisted network for freespace segmentation in unstructured road scenes
Displays(2024)
摘要
Freespace segmentation is of paramount importance for robust and safe autonomous navigation. However, existing research often focuses on structured road environments, where freespace boundaries are relatively straightforward. In contrast, freespace segmentation in unstructured environments poses greater challenges due to complex boundaries. To improve segmentation accuracy in unstructured scenes, researchers often resort to using large and deep networks, resulting in heightened model complexity and deployment challenges. In this paper, we propose a novel Lightweight Boundary-Assisted Segmentation Network (LB-ASN) to efficiently address freespace segmentation in unstructured environments. Firstly, we propose a multitask framework assisted by boundary information and optimize the network through deep supervision using boundary masks. Additionally, we introduce the Channel Split-Regroup Module (CS-RM), a novel component leveraging boundary information for feature fusion. This module aims to enhance feature representation by integrating fine-grained details from shallow layers and semantic information from deeper layers. Furthermore, we introduce a new lightweight structure, called Multi-head Hadamard Attention Module (MHAM), efficiently capturing spatial information from various perspectives while simultaneously enhancing the model’s efficiency. Extensive evaluations on the WVD, RUGD, and RELLIS datasets, representative of unstructured environments, demonstrate that LB-ASN achieves state-of-the-art performance with high accuracy and low parameter count. Specifically, LB-ASN, with only 0.46M parameters, achieves an IoU of 97.41% on the WVD dataset and 95.44% on the RUGD dataset, underscoring its effectiveness and superiority in unstructured environments.
更多查看译文
关键词
Freespace segmentation,Lightweight,Boundary-assist
AI 理解论文
溯源树
样例
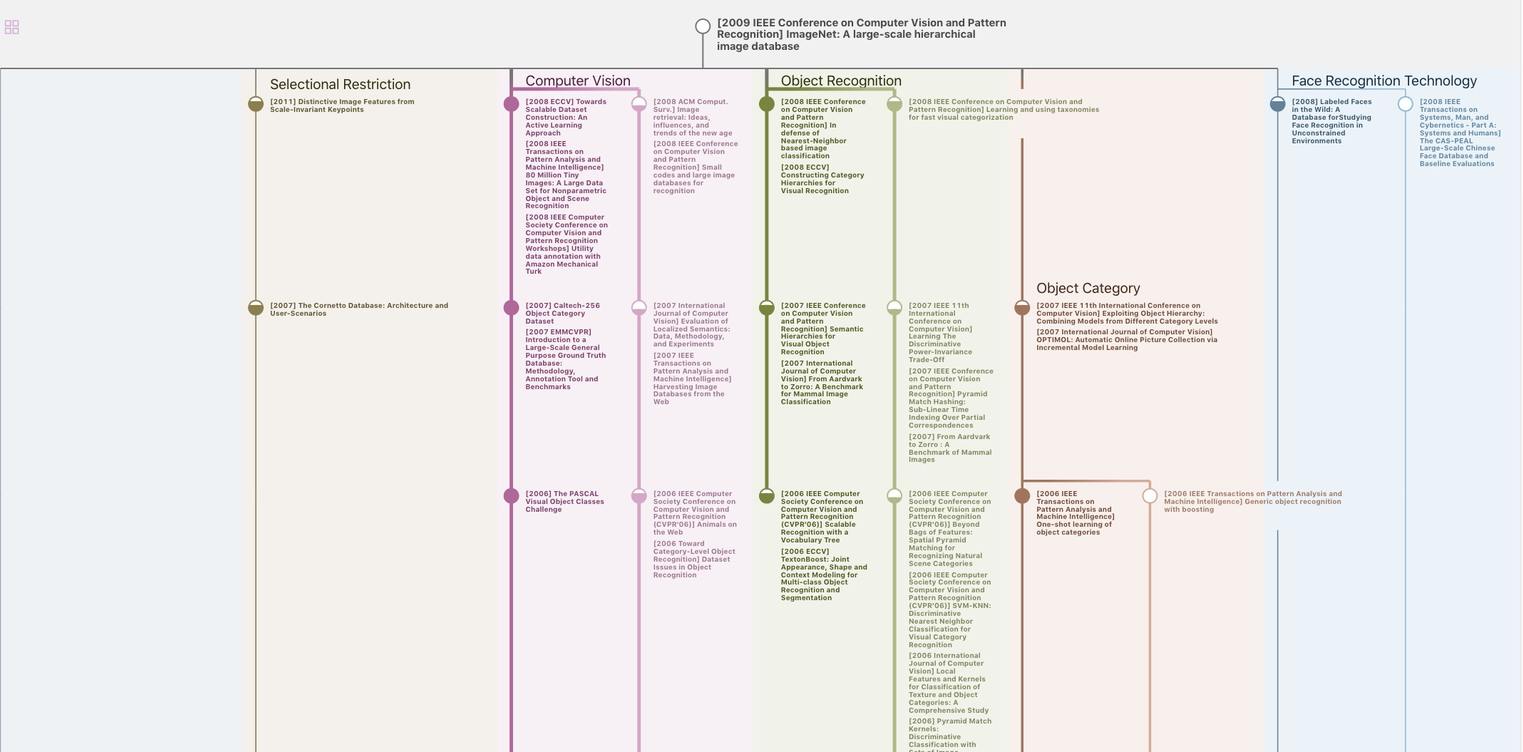
生成溯源树,研究论文发展脉络
Chat Paper
正在生成论文摘要