Vibration Signal-Based Fusion Residual Attention Model for Power Transformer Fault Diagnosis
IEEE Sensors Journal(2024)
摘要
In recent years, power transformer fault diagnosis based on deep learning has gradually become mainstream. However, inadequate use of the data collected by sensors and difficulties in extracting the key fault features lead to poor accuracy of the model. To address this problem, a fusion residual attention diagnosis (FRAD) model is proposed to evaluate power transformer faults using vibration signals. Specifically, a gradient guided filtering module (GGFM) is designed to generate images from the original vibration signals and to fuse different position images. An up-dimensioning convolutional block attention module (UCBAM) is proposed to improve atrous deep residual network (IADRN), based on which the fused images are used to complete the fault diagnosis of the transformer. Datasets collected from a 10kV real power transformer validate the proposed model. The experimental results show that the proposed model outperforms comparison methods in terms of Precision, Recall, F1 score, and Accuracy. Especially, the fault diagnosis accuracy of the proposed model is 99.38%.
更多查看译文
关键词
Power transformers,fault diagnosis,image fusion,deep residual network,convolutional block attention module
AI 理解论文
溯源树
样例
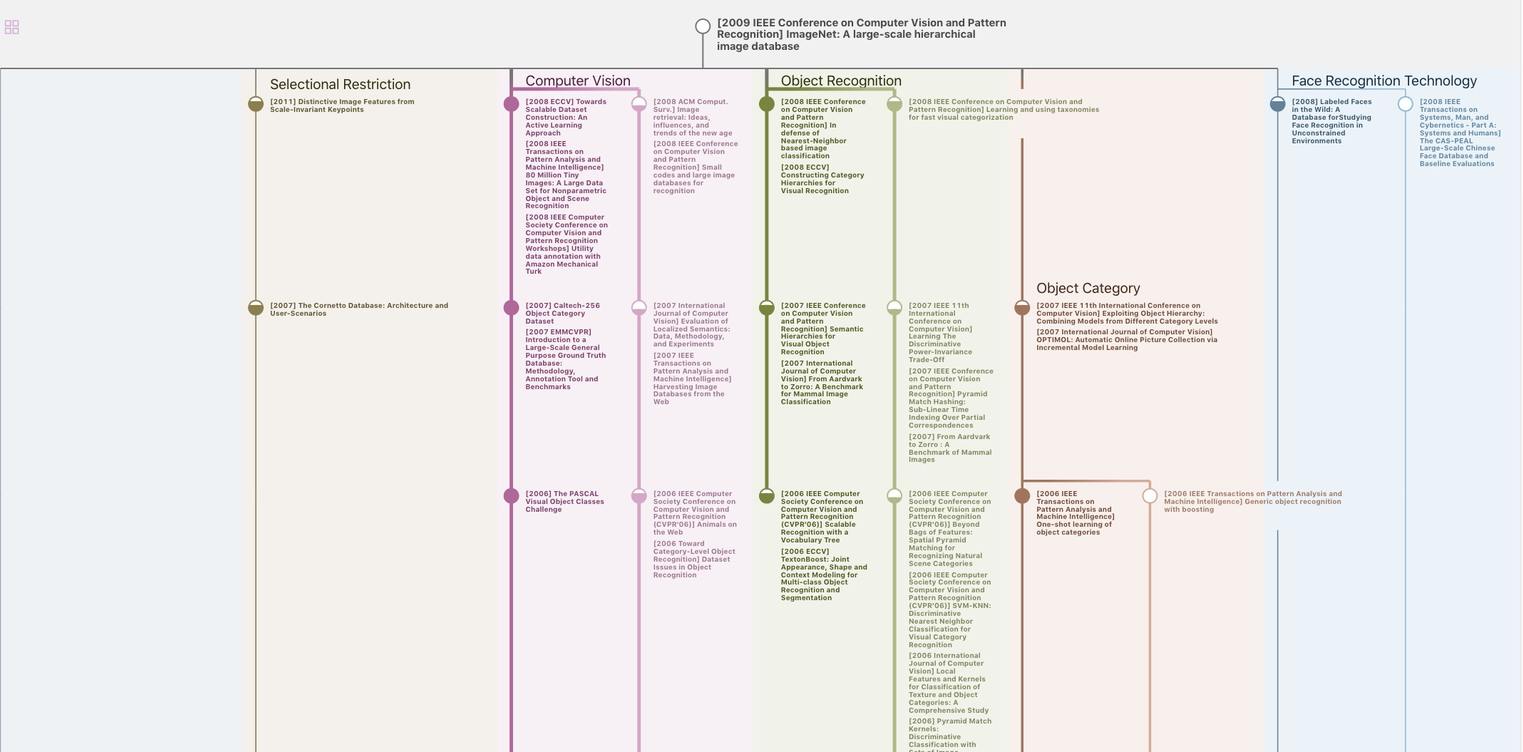
生成溯源树,研究论文发展脉络
Chat Paper
正在生成论文摘要