Universal Approximation Abilities of a Modular Differentiable Neural Network
IEEE TRANSACTIONS ON NEURAL NETWORKS AND LEARNING SYSTEMS(2024)
摘要
Approximation ability is one of the most important topics in the field of neural networks (NNs). Feedforward NNs, activated by rectified linear units and some of their specific smoothed versions, provide universal approximators to convex as well as continuous functions. However, most of these networks are investigated empirically, or their characteristics are analyzed based on specific operation rules. Moreover, an adequate level of interpretability of the networks is missing as well. In this work, we propose a class of new network architecture, built with reusable neural modules (functional blocks), to supply differentiable and interpretable approximators for convex and continuous target functions. Specifically, first, we introduce a concrete model construction mechanism with particular blocks based on differentiable programming and the composition essence of the max operator, extending the scope of existing activation functions. Moreover, explicit block diagrams are provided for a clear understanding of the external architecture and the internal processing mechanism. Subsequently, the approximation behavior of the proposed network to convex functions and continuous functions is rigorously proved as well, by virtue of mathematical induction. Finally, plenty of numerical experiments are conducted on a wide variety of problems, which exhibit the effectiveness and the superiority of the proposed model over some existing ones.
更多查看译文
关键词
Artificial neural networks,Optimization,Network architecture,Vectors,Petroleum,Deep learning,Approximation algorithms,Approximation,differentiable programming (DP),functional blocks,interpretability,neural network (NN),rectified linear unit (ReLU)
AI 理解论文
溯源树
样例
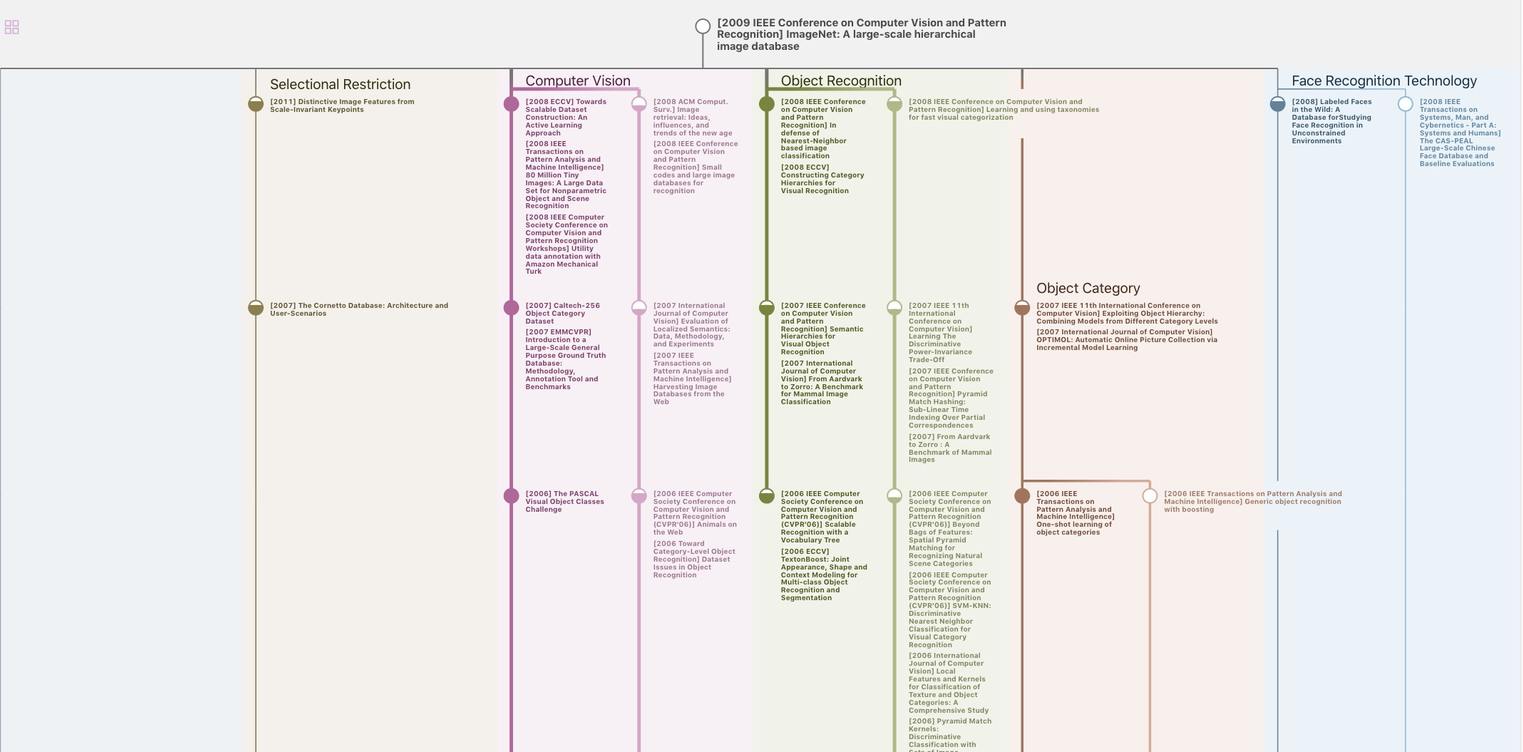
生成溯源树,研究论文发展脉络
Chat Paper
正在生成论文摘要