A Multi-modal Classification Method for Early Diagnosis of Mild Cognitive Impairment and Alzheimer’s Disease Using Three Paradigms with Various Task Difficulties
IEEE Transactions on Neural Systems and Rehabilitation Engineering(2024)
摘要
Alzheimer’s Disease (AD) accounts for the majority of dementia, and Mild Cognitive Impairment (MCI) is the early stage of AD. Early and accurate diagnosis of dementia plays a vital role in more targeted treatments and effectively halting disease progression. However, the clinical diagnosis of dementia requires various examinations, which are expensive and require a high level of expertise from the doctor. In this paper, we proposed a classification method based on multi-modal data including Electroencephalogram (EEG), eye tracking and behavioral data for early diagnosis of AD and MCI. Paradigms with various task difficulties were used to identify different severity of dementia: eye movement task and resting-state EEG tasks were used to detect AD, while eye movement task and delayed match-to-sample task were used to detect MCI. Besides, the effects of different features were compared and suitable EEG channels were selected for the detection. Furthermore, we proposed a data augmentation method to enlarge the dataset, designed an extra ERPNet feature extract layer to extract multi-modal features and used domain-adversarial neural network to improve the performance of MCI diagnosis. We achieved an average accuracy of 88.81% for MCI diagnosis and 100% for AD diagnosis. The results of this paper suggest that our classification method can provide a feasible and affordable way to diagnose dementia.
更多查看译文
关键词
Dementia,Multi-modal,Machine learning,Domain-Adversarial Neural Network
AI 理解论文
溯源树
样例
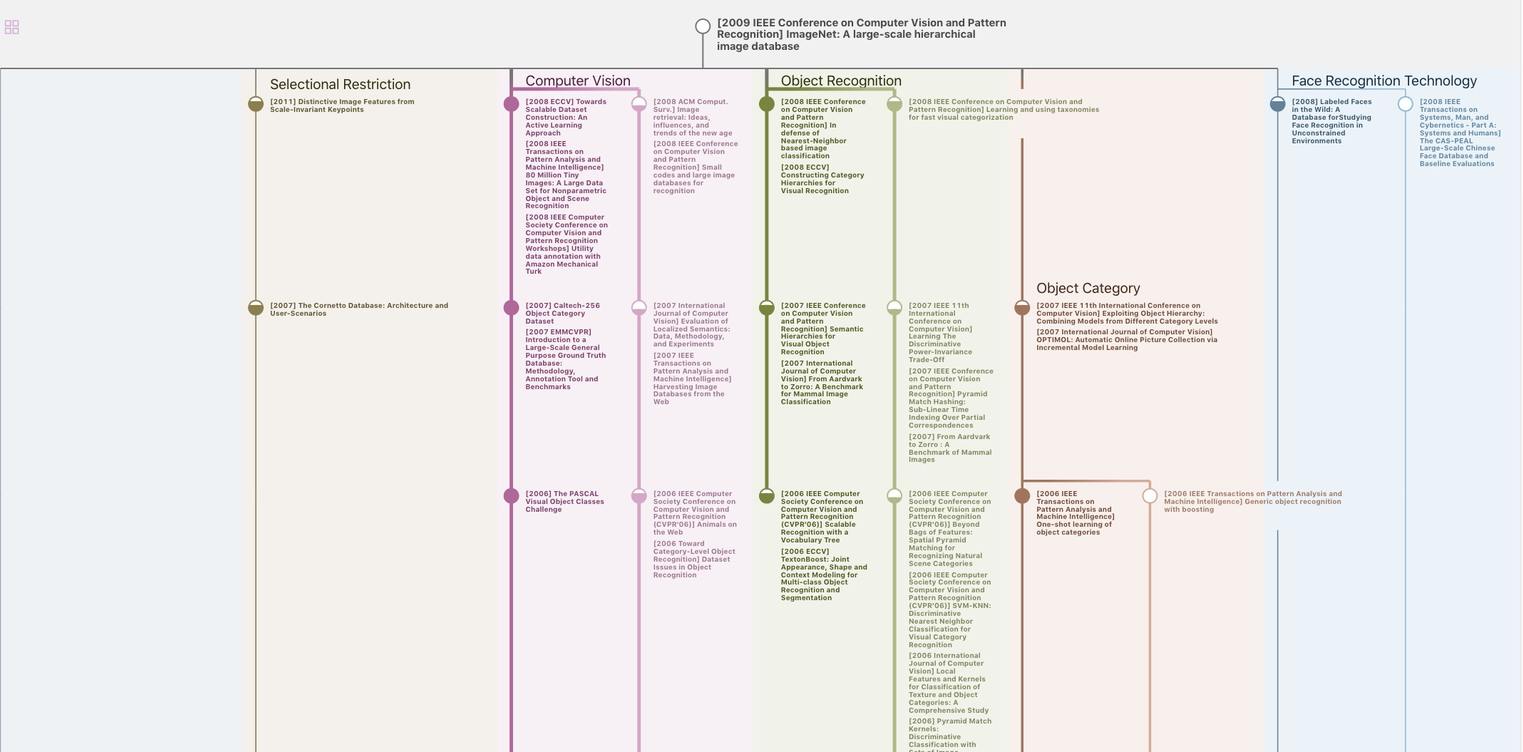
生成溯源树,研究论文发展脉络
Chat Paper
正在生成论文摘要