Multi-DORGP for fast uncertainty quantification of multi-scale irregular defects in super large-scale fiber-reinforced composite
Composites Part A: Applied Science and Manufacturing(2024)
摘要
The presence of randomly distributed irregular defects in fiber-reinforced composites significantly impacts the structural response, yet traditional machine learning schemes often require an extensive number of samples, resulting in time-consuming processes. Therefore, this paper introduces a novel approach, termed the dual order-reduced Gaussian Process emulators (Multi-DORGP), aimed at efficiently quantifying the uncertainty of multi-scale irregular defects in fiber-reinforced composites. This is achieved by accurately characterizing the multi-scale irregular defects through precise geometric representations, utilizing massive discrete nodes and fine meshes to address the limitations of parameterization methods that may overlook shape and size differences of defects. Moreover, we comprehensively quantify uncertainty across micro, meso, and macro scales, considering spatially randomly distributed locations, sizes, and irregular shapes of defects. Notably, the Multi-DORGP scheme is presented to alleviate the computational burden associated with extremely high-dimensional data (e.g., up to 23.3 million variables). In which, we decouple and build the latent spaces for raw data assisted by principal component analysis, and Gaussian Process regression is built and trained by the coefficients between the latent spaces. Through illustrative examples, including a real-life application involving an airplane wing, we validate the proposed scheme's capability to accurately quantify the uncertainty of multi-scale irregular defects in large-scale fiber-reinforced composites using significantly few samples (e.g., 100).
更多查看译文
关键词
Carbon fibres,Mechanical properties,Computational modelling,Data-driven prediction using limited data
AI 理解论文
溯源树
样例
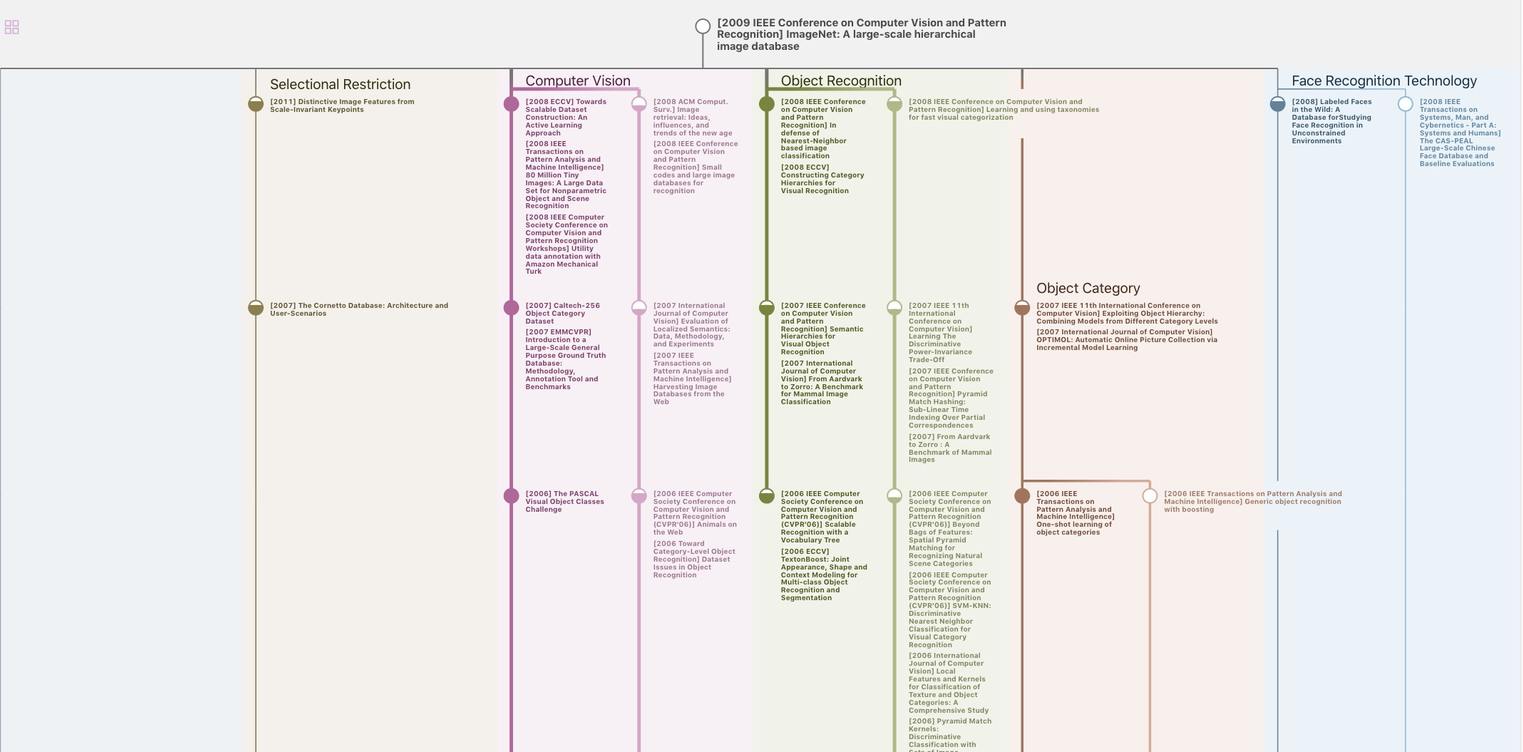
生成溯源树,研究论文发展脉络
Chat Paper
正在生成论文摘要