Unmasking Correlations in Nuclear Cross Sections with Graph Neural Networks
arxiv(2024)
摘要
In this work, we explore the use of deep learning techniques to learn the
relationships between nuclear cross-sections across the chart of isotopes. As a
proof of principle, we focus on the neutron-induced reactions in the fast
energy regime that are the most important in nuclear science and engineering.
We use variational autoencoders (VAEs) and implicit neural representations
(INRs) to build a learned feature representation space of nuclear cross
sections and reduce the dimensionality of the problem. We then train graph
neural networks (GNNs) on the resulting latent space to leverage the
topological information encoded in the chart of isotopes and to capture the
relationships between cross sections in different nuclei. We find that
hypernetworks based on INRs significantly outperforms VAEs in encoding nuclear
cross-sections. This superiority is attributed to INR's ability to model
complex, varying frequency details, which enables lower prediction errors when
combined with GNNs. We also observe that GNN optimization is much more
successful when performed in the latent space, whether using INRs or VAEs.
However VAEs' continuous representation also allows for direct GNN training in
the original input space. We leverage these representational learning
techniques and successfully predict cross sections for a 17x17 block of nuclei
with high accuracy and precision. These findings suggest that both
representation encoding of cross-sections and the prediction task hold
significant potential in augmenting nuclear theory models, e.g., providing
reliable estimates of covariances of cross sections, including cross-material
covariances.
更多查看译文
AI 理解论文
溯源树
样例
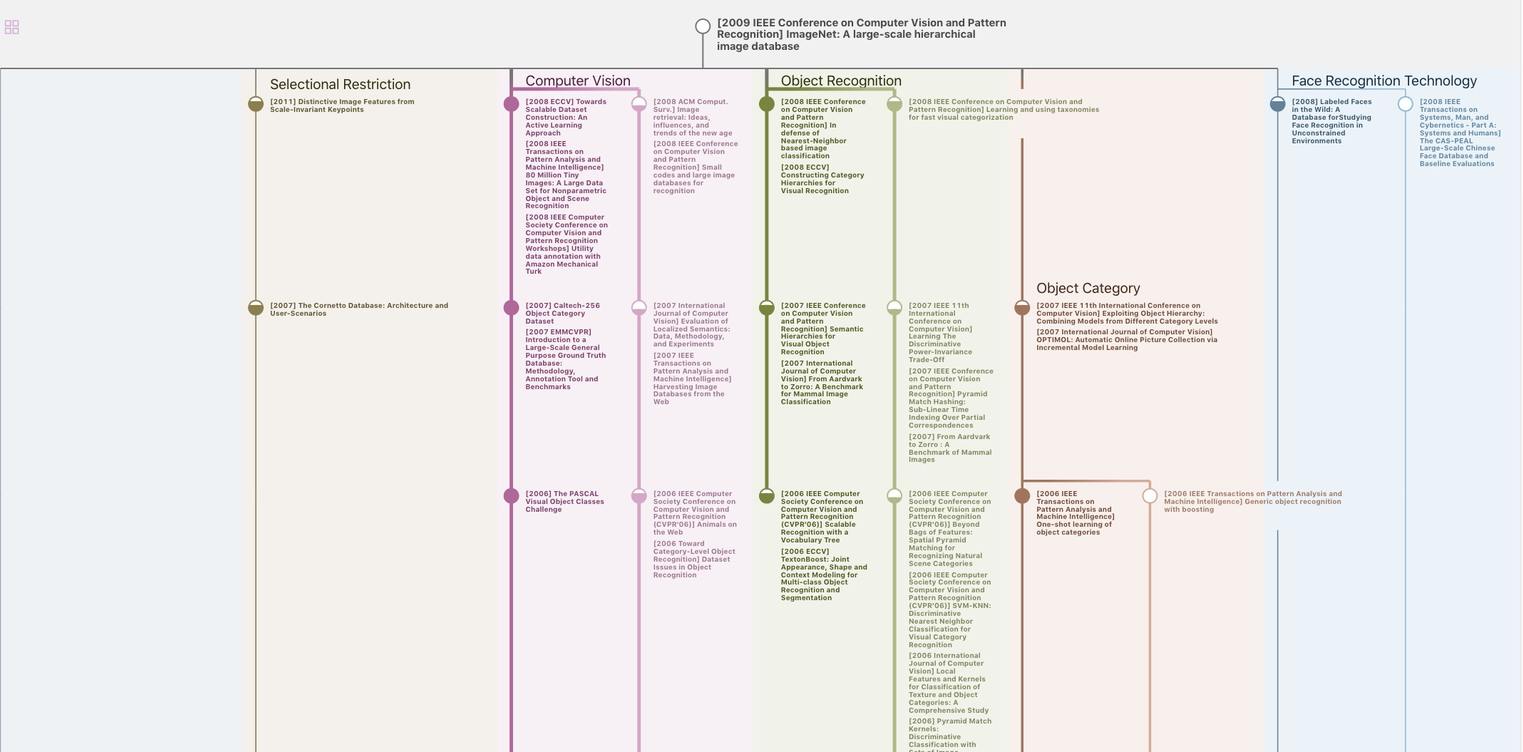
生成溯源树,研究论文发展脉络
Chat Paper
正在生成论文摘要