Combining Rosetta Sequence Design with Protein Language Model Predictions Using Evolutionary Scale Modeling (ESM) as Restraint
ACS SYNTHETIC BIOLOGY(2024)
摘要
Computational protein sequence design has the ambitious goal of modifying existing or creating new proteins; however, designing stable and functional proteins is challenging without predictability of protein dynamics and allostery. Informing protein design methods with evolutionary information limits the mutational space to more native-like sequences and results in increased stability while maintaining functions. Recently, language models, trained on millions of protein sequences, have shown impressive performance in predicting the effects of mutations. Assessing Rosetta-designed sequences with a language model showed scores that were worse than those of their original sequence. To inform Rosetta design protocols with language model predictions, we added a new metric to restrain the energy function during design using the Evolutionary Scale Modeling (ESM) model. The resulting sequences have better language model scores and similar sequence recovery, with only a minor decrease in the fitness as assessed by Rosetta energy. In conclusion, our work combines the strength of recent machine learning approaches with the Rosetta protein design toolbox.
更多查看译文
关键词
computational protein design,protein language models,Rosetta,evolutionary fitness,thermodynamicstability,de novo proteins,proteinlanguage model
AI 理解论文
溯源树
样例
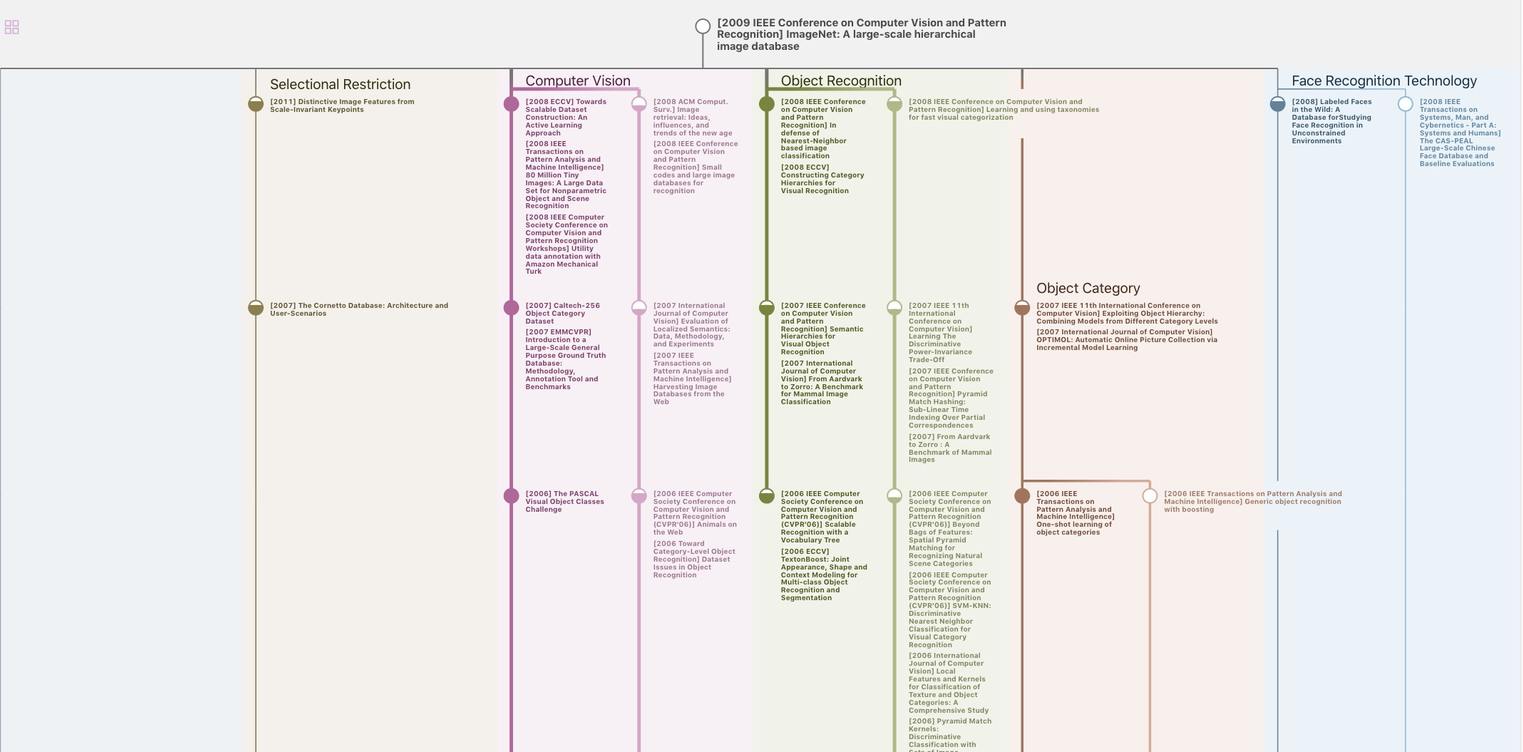
生成溯源树,研究论文发展脉络
Chat Paper
正在生成论文摘要