An unsupervised low-light image enhancement method for improving V-SLAM localization in uneven low-light construction sites
Automation in Construction(2024)
摘要
Construction robots have been increasingly adopted in construction projects to improve productivity, reduce risk, and speed up work cycles. Visual Simultaneous Localization and Mapping (V-SLAM) technology is widely used in construction robots due to its lightweight weight, cost-effectiveness, and provision of semantic information. On real construction sites, lighting conditions are often challenging due to factors such as uneven lighting intensity, inadequate exposure, or robots in backlit positions (e.g. roughcast houses without artificial lighting, underground car parks), which cause images captured under these conditions to exhibit low signal-to-noise ratio, uneven lighting, color distortion, noise, and other problems. These challenges make the extraction and matching of feature points in V-SLAM difficult, resulting in significant positioning errors or the inability to generate positioning trajectories for construction robotics. Although existing methods for enhancing low-light images can certainly improve image brightness, they still cannot effectively address the localization challenges brought about by low-light construction scenes with uneven illumination and noise. To address the interference of uneven and changing lighting conditions on V-SLAM localization in construction sites, this paper proposes the Unsupervised Reflectance Retinex and Noise model (URRN-Net) to enhance low-light construction images. By using a CNN model based on the Retinex theory to decompose the illumination characteristics of images, we achieve effective brightness restoration of uneven low-light images by utilizing unsupervised loss functions. URRE-Net can reduce the root mean square localization error by >65% compared to low-light images in the ORB-SLAM3 method, and the maximum error is reduced by >71% in on-site experiments. The proposed URRE-Net can be integrated with existing V-SLAM algorithms to provide more robust localization services for low-light construction site applications such as building operations (e.g., interior wall spraying robotic) or construction management tasks (e.g., automatic tunnel inspection).
更多查看译文
关键词
Low-light image enhancement,Construction robots,Retinex,Simultaneous localization and mapping
AI 理解论文
溯源树
样例
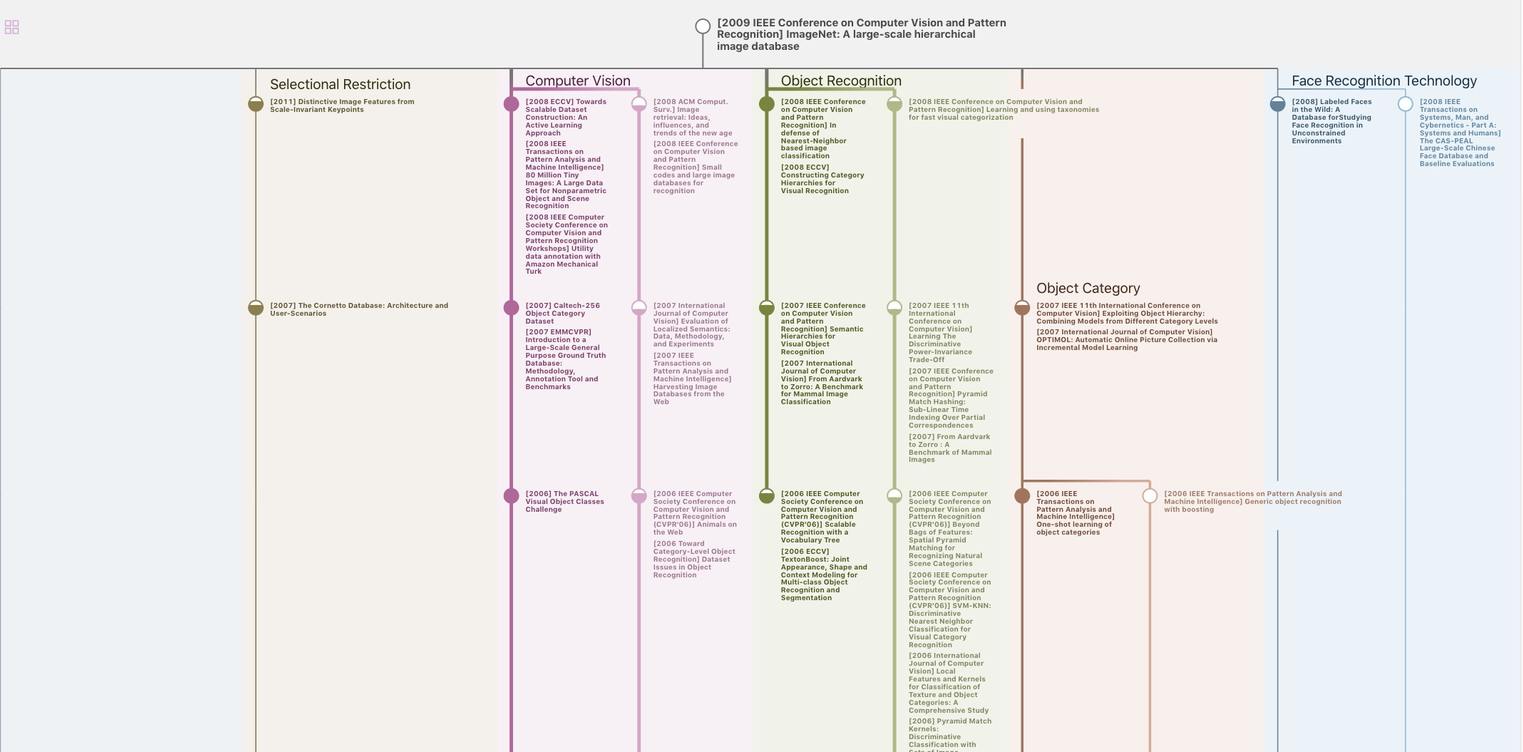
生成溯源树,研究论文发展脉络
Chat Paper
正在生成论文摘要