Stellar: Energy-Efficient and Low-Latency SNN Algorithm and Hardware Co-Design with Spatiotemporal Computation.
International Symposium on High-Performance Computer Architecture(2024)
摘要
The brain-inspired Spiking Neural Network (SNN) has great potential to reduce energy consumption in AI applications. However, the state-of-the-art SNN algorithms focus on high accuracy and large sparsity by constructing complex neuron models with sparse spike generation, leading to low energy efficiency and high latency. The state-of-the-art SNN hardware designs are hard to exploit high data reuse and parallel processing dataflows due to the irregularity and time-dependency of the spikes. To address the above issues, in this work we propose STELLAR, an algorithm-hardware co-design framework exploiting rich spatiotemporal dynamics of the SNN for high energy efficiency and low latency while maintaining high accuracy. Firstly, based on the Few Spikes (FS) neuron, we propose few spikes backpropagation (FSBP) and its training flow with strong hardware awareness to adaptively train the deep SNN for a short time window and few spikes. The resulting sparse SNN enjoys rapid inference with few synaptic operations and competitive accuracy. Secondly, we propose a dedicated SNN architecture and spatiotemporal Row Stationary (stRS) dataflow to exploit large sparsity brought by the proposed algorithm for highly parallel and energy -efficient computation. Several techniques have been proposed to boost energy efficiency and speedup while maintaining accuracy, including the window-based parallel processing technique and the spatiotemporal encoding-based computation architecture. The experimental results show that 1) on the algorithm level, STELLAR outperforms the state-of-the-art SNN models with significantly fewer spikes and shorter time window on both static and neuromorphic datasets with higher or comparable accuracy; 2) on the architecture level, compared with several SOTA SNN hardware designs, STELLAR achieves up to 8.1 × energy efficiency and 7.1 × speedup.
更多查看译文
AI 理解论文
溯源树
样例
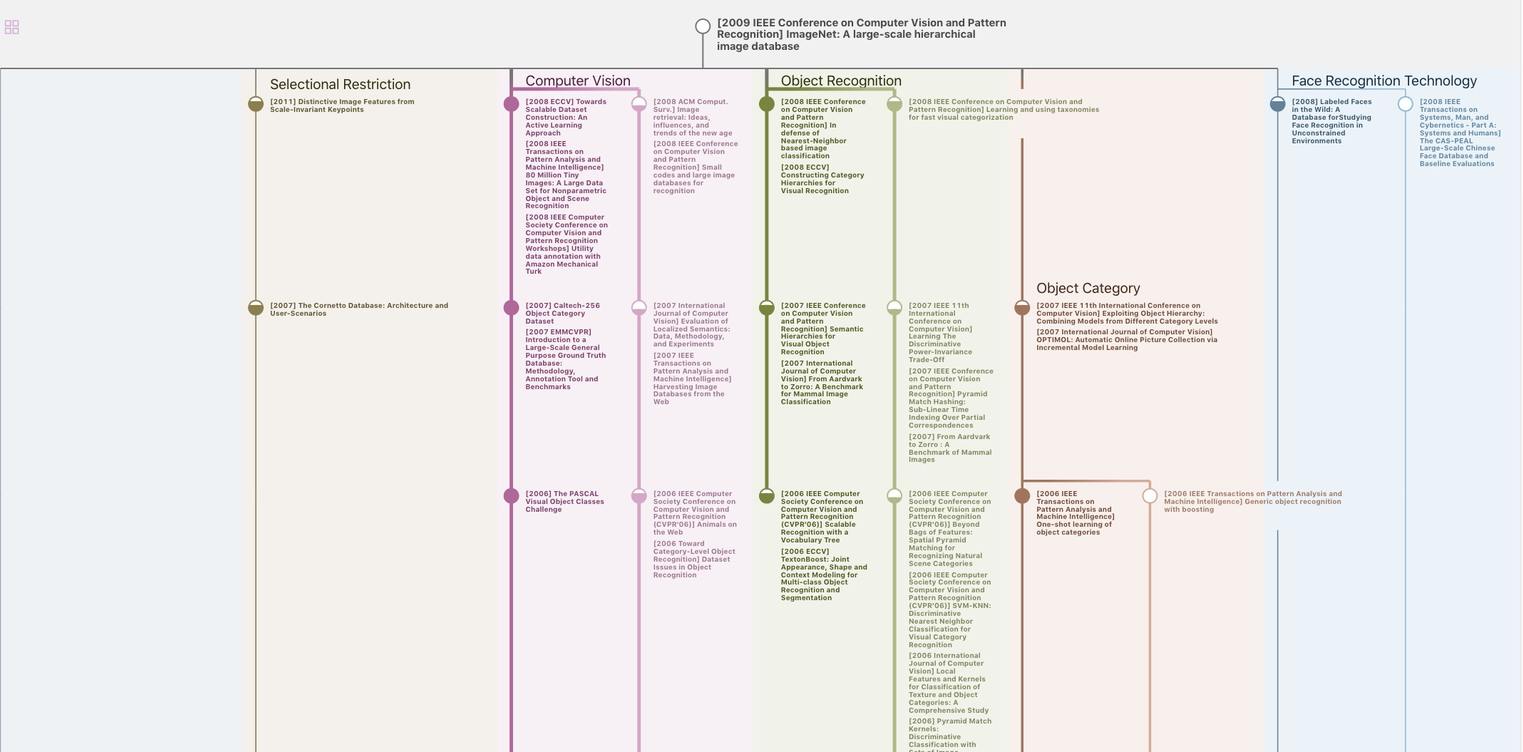
生成溯源树,研究论文发展脉络
Chat Paper
正在生成论文摘要