Technical indicator enhanced ultra-short-term wind power forecasting based on long short-term memory network combined XGBoost algorithm
IET RENEWABLE POWER GENERATION(2024)
摘要
The growing integration of renewable energy sources into the power grid has introduced unprecedented uncertainty. Ensuring an appropriately scheduled reserve is essential to accommodate renewable energy's intermittent and volatile nature. This study introduces an innovative approach to ultra-short-term wind power forecasting, which relies on feature engineering and a hybrid model. The effectiveness of this proposed method is showcased through a case study involving a utility-scale wind farm in Inner Mongolia, China. The findings indicate that the hybrid model, which combines the XGBoost (Extreme Gradient Boosting) algorithm and LSTM (Long Short-Term Memory) network with KDJ (Stochastic Oscillator), and MACD (Moving Average Convergence and Divergence), achieves the highest forecasting accuracy. Specifically, the proposed model yields a normalized mean absolute error of 0.0396 for wind power forecasting. The modelling and forecasting process takes approximately 550 s. Furthermore, the suggested method is employed to predict wind power and wind speed for a wind farm in the USA. The experimental results consistently indicate that the proposed model maintains a dependable performance across various raw datasets; it is suitable for use in power system operations. An increasing penetration of renewable energy has brought unprecedented uncertainty to today's grid, and properly scheduled reserve is a key to accommodate the intermittency and volatility of renewable energy. In this manuscript, the authors propose a new method for ultra-short-term wind power forecasting based on the long short-term memory network with XGBoost algorithm and combining future wind speed and wind power technical indicators. The authors develop a case study using wind power datasets from two different countries, and experiments show that the proposed method outperforms common machine learning and deep learning models in terms of prediction accuracy and computational efficiency, and has strong generalization capabilities. image
更多查看译文
关键词
data analysis,learning (artificial intelligence),prediction theory,wind power
AI 理解论文
溯源树
样例
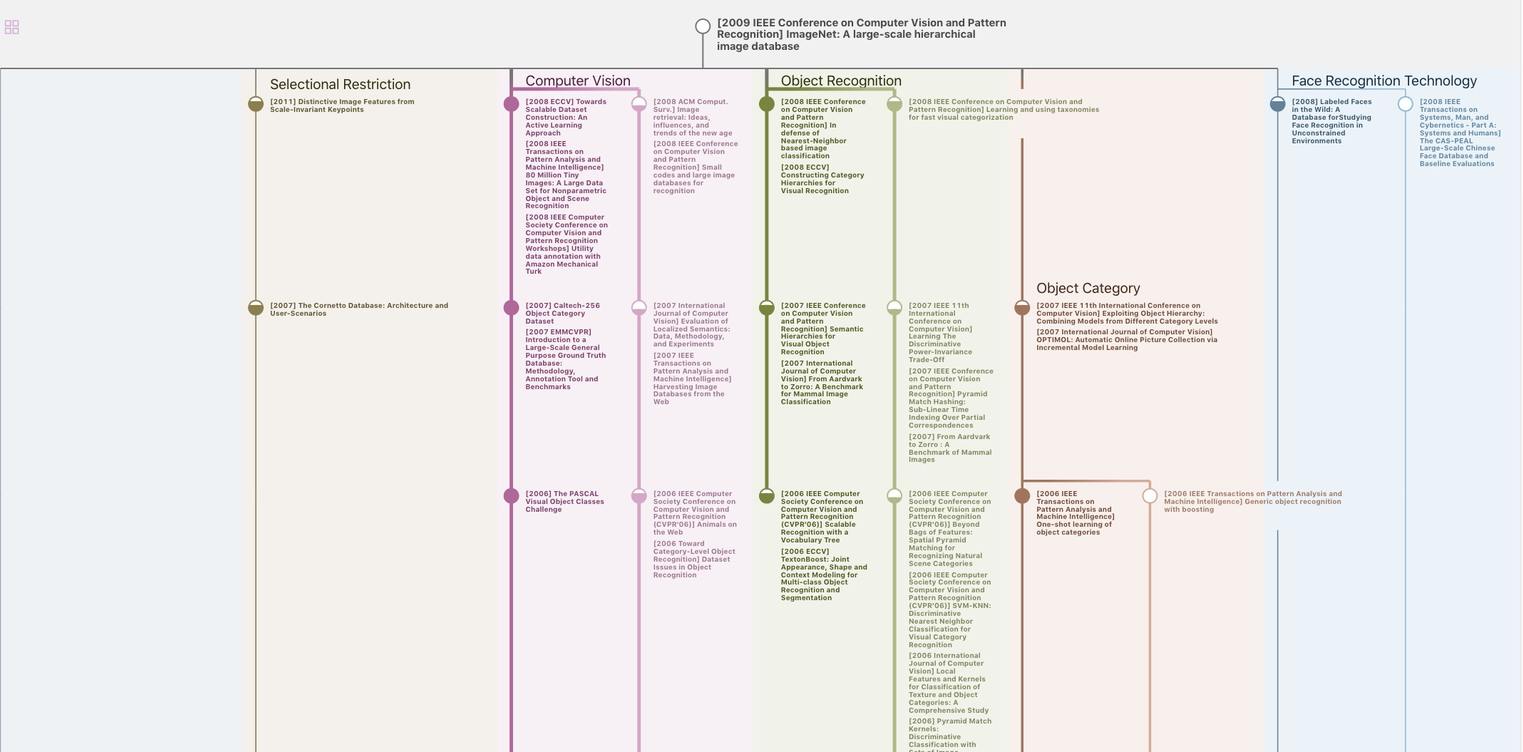
生成溯源树,研究论文发展脉络
Chat Paper
正在生成论文摘要