Double Wins: Boosting Accuracy and Fifficiency of Graph Neural Networks by Reliable Knowledge Distillation
23RD IEEE INTERNATIONAL CONFERENCE ON DATA MINING, ICDM 2023(2023)
摘要
The recent breakthrough achieved by graph neural networks (GNNs) with few labeled data accelerates the pace of deploying GNNs on real-world applications. While several efforts have been made to scale GNNs training for large-scale graphs, GNNs still suffer from the scalability challenge of model inference, due to the graph dependency issue incurred by the message-passing mechanism, therefore hindering its deployment in resource-constrained applications. An intuitive remedy is compressing the cumbersome GNN model into inference -friendly multi-layer perceptrons (MLPs) using knowledge distillation (KD). However, the standard KI) strategy, i.e., training MLPs using the soft labels of labeled and unlabeled nodes from the teacher, is suboptimal, since the GNN teacher would inevitably make wrong predictions for unlabeled data, especially in the semisupervised scenario. To address this, we propose a novel Reliable Knowledge Distillation framework for MLP optimization (RKDMLP), which shows strong promise in achieving a "sweet point" in co-optimizing model accuracy and efficiency. Its core insight is to use a meta -policy to filter out those unreliable soft labels. To train the meta-policy, we design a reward -driven objective based on a meta -set and adopt policy gradient to optimize the expected reward. Then we apply the meta -policy to the unlabeled nodes and select the most reliable soft labels for distillation. Extensive experiments across various GNN backbones, on 7 small graphs and 2 large-scale datasets from the challenging ()pen Graph Benchmark, demonstrate the superiority of our proposal. Moreover, RKD-MLP also shows good robustness w.r.t. graph topology and node feature noises.
更多查看译文
关键词
Graph neural networks,knowledge distillation,learnable data distillation
AI 理解论文
溯源树
样例
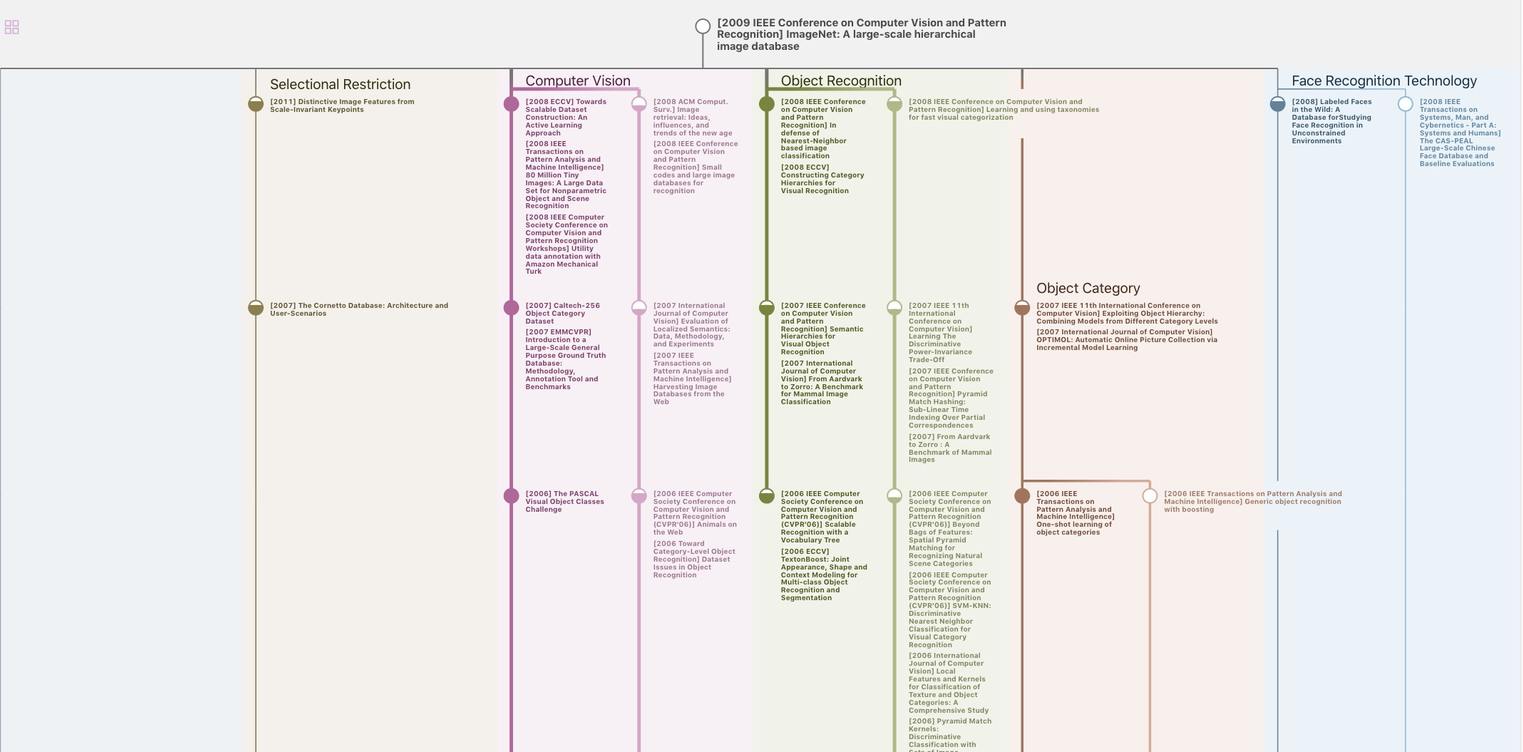
生成溯源树,研究论文发展脉络
Chat Paper
正在生成论文摘要