All about Sample-Size Calculations for A/B Testing: Novel Extensions & Practical Guide
PROCEEDINGS OF THE 32ND ACM INTERNATIONAL CONFERENCE ON INFORMATION AND KNOWLEDGE MANAGEMENT, CIKM 2023(2023)
摘要
While there exists a large amount of literature on the general challenges and best practices for trustworthy onlineA/Btesting, there are limited studies on sample size estimation, which plays a crucial role in trustworthy and efficient A/B testing that ensures the resulting inference has a sufficient power and type I error control. For example, when sample size is under-estimated, the statistical inference, even with the correct analysis methods, will not be able to detect the true significantimprovementleading to misinformedandcostly decisions. Thispaper addresses this fundamental gapbydeveloping newsample size calculation methods for correlated data, aswell as absolute vs. relative treatment effects, both ubiquitous in online experiments. Additionally, we address a practical question of the minimal observed difference that will be statistically significant and howit relates to average treatment effect and sample size calculation. All proposed methods are accompanied by mathematical proofs, illustrative examples, and simulations. We end by sharing some best practices on various practical topics on sample size calculation and experimental design.
更多查看译文
关键词
A/B testing,online controlled experiments,sample size calculation,correlated data,absolute lift,relative lift,minimally observed difference
AI 理解论文
溯源树
样例
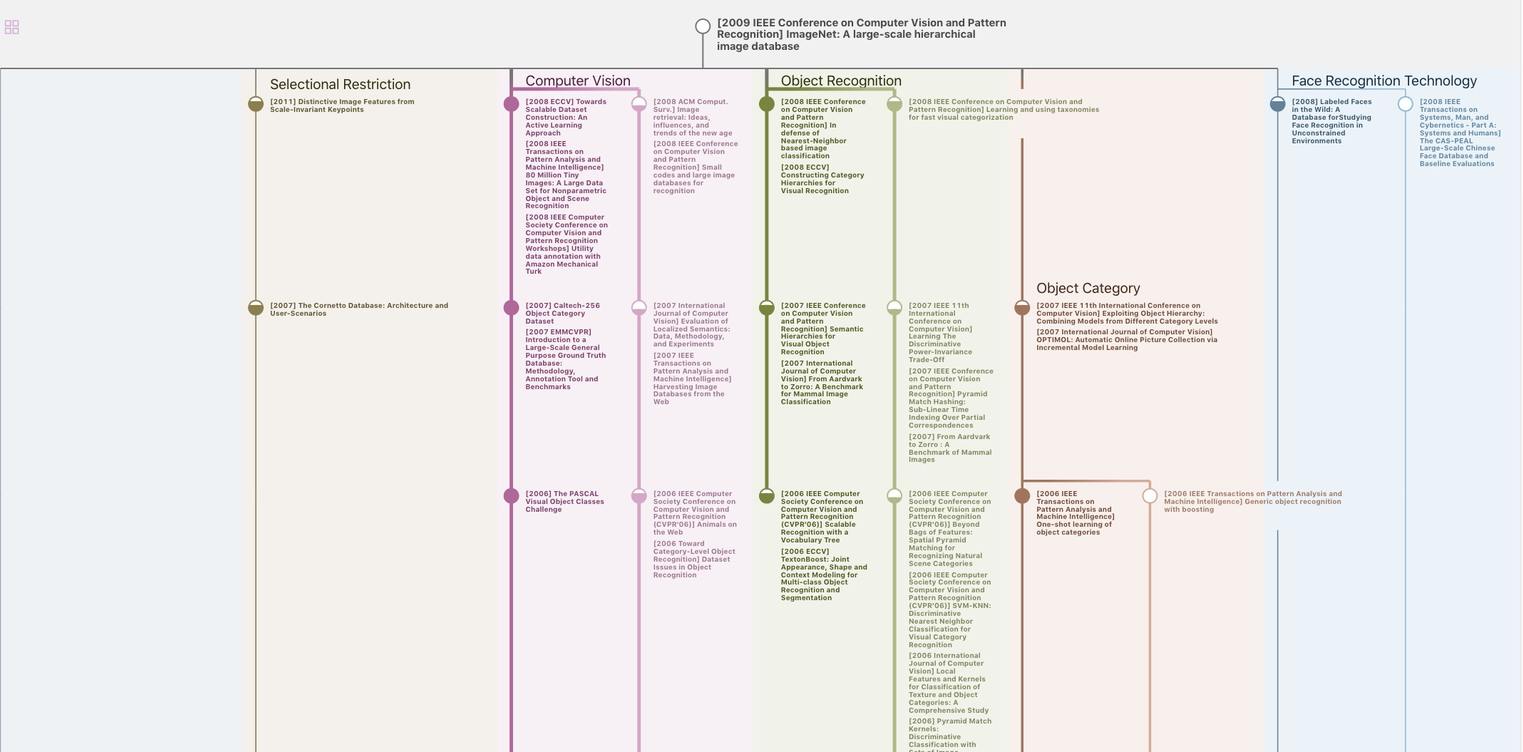
生成溯源树,研究论文发展脉络
Chat Paper
正在生成论文摘要