Revealing and reducing bias when modelling choice behaviour on imbalanced panel datasets
JOURNAL OF CHOICE MODELLING(2024)
摘要
The emergence of modern tools and technologies gives a unique opportunity to collect large amounts of data for understanding behaviour. However, the generated datasets are often imbalanced, as individuals might contribute to the datasets at different frequencies and periods. Models based on these datasets are challenging to estimate, and the results are not straightforward to interpret without considering the sample structure. This study investigates the issue of handling imbalanced panel datasets for modelling individual behaviour. It first conducts a simulation experiment to study to which degree mixed logit models with and without panel reproduce the population preferences when using imbalanced data. It then investigates how the application of bias reduction strategies, such as subsampling and likelihood weighting, influences model results and finds that combining these techniques helps to find an optimal trade-off between bias and variance of the estimates. Considering the conclusions from the simulation study, a large-scale case study estimates bicycle route choice models with different correction strategies. These strategies are compared in terms of efficiency, weighted fit measures, and computational burden to provide recommendations that fit the modelling purpose. We find that the weighted panel mixed multinomial logit model, estimated on the entire dataset, performs best in terms of minimising the bias -efficiency trade-off in the estimates. Finally, we propose a strategy that ensures equal contribution of each individual to the estimation results, regardless of their representation in the sample, while reducing the computational burden related to estimating models on large datasets.
更多查看译文
关键词
Imbalanced panel,Panel mixed multinomial logit model,Subsampling,Weighting,Bias-efficiency trade-off
AI 理解论文
溯源树
样例
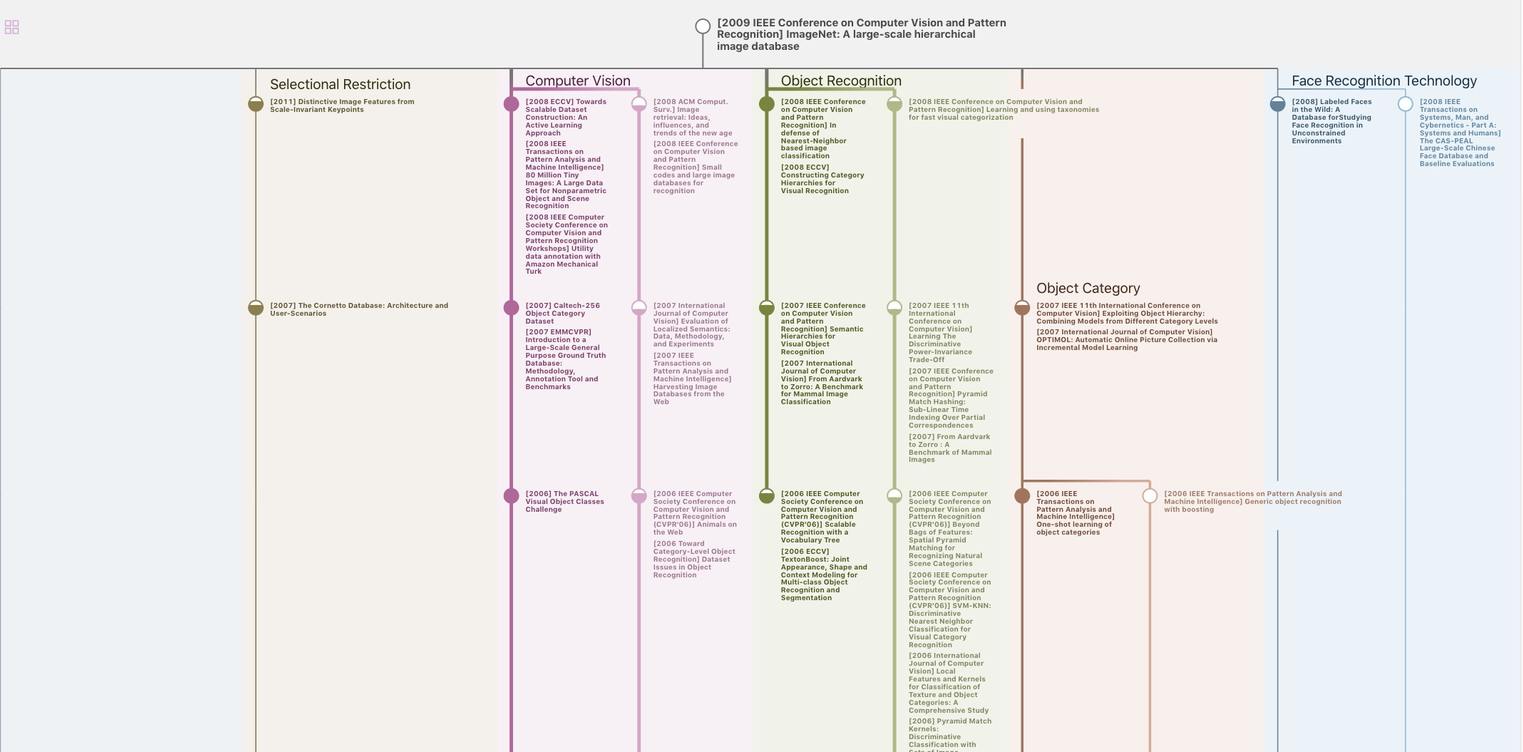
生成溯源树,研究论文发展脉络
Chat Paper
正在生成论文摘要