Discovery of Toxin-Degrading Enzymes with Positive Unlabeled Deep Learning
ACS CATALYSIS(2024)
摘要
Identifying functional enzymes for the catalysis of specific biochemical reactions is a major bottleneck in the de novo design of biosynthesis and biodegradation pathways. Conventional methods based on microbial screening and functional metagenomics require long verification periods and incur high experimental costs; recent data-driven methods apply only to a few common substrates. To enable rapid and high-throughput identification of enzymes for complex and less-studied substrates, we propose a robust enzyme's substrate promiscuity prediction model based on positive unlabeled learning. Using this model, we identified 15 new degrading enzymes specific for the mycotoxins ochratoxin A and zearalenone, of which six could degrade >90% mycotoxin content within 3 h. We anticipate that this model will serve as a useful tool for identifying new functional enzymes and understanding the nature of biocatalysis, thereby advancing the fields of synthetic biology, metabolic engineering, and pollutant biodegradation.
更多查看译文
关键词
biodegradation,synthetic biology,food safety,mycotoxin,machine learning,cheminformatics
AI 理解论文
溯源树
样例
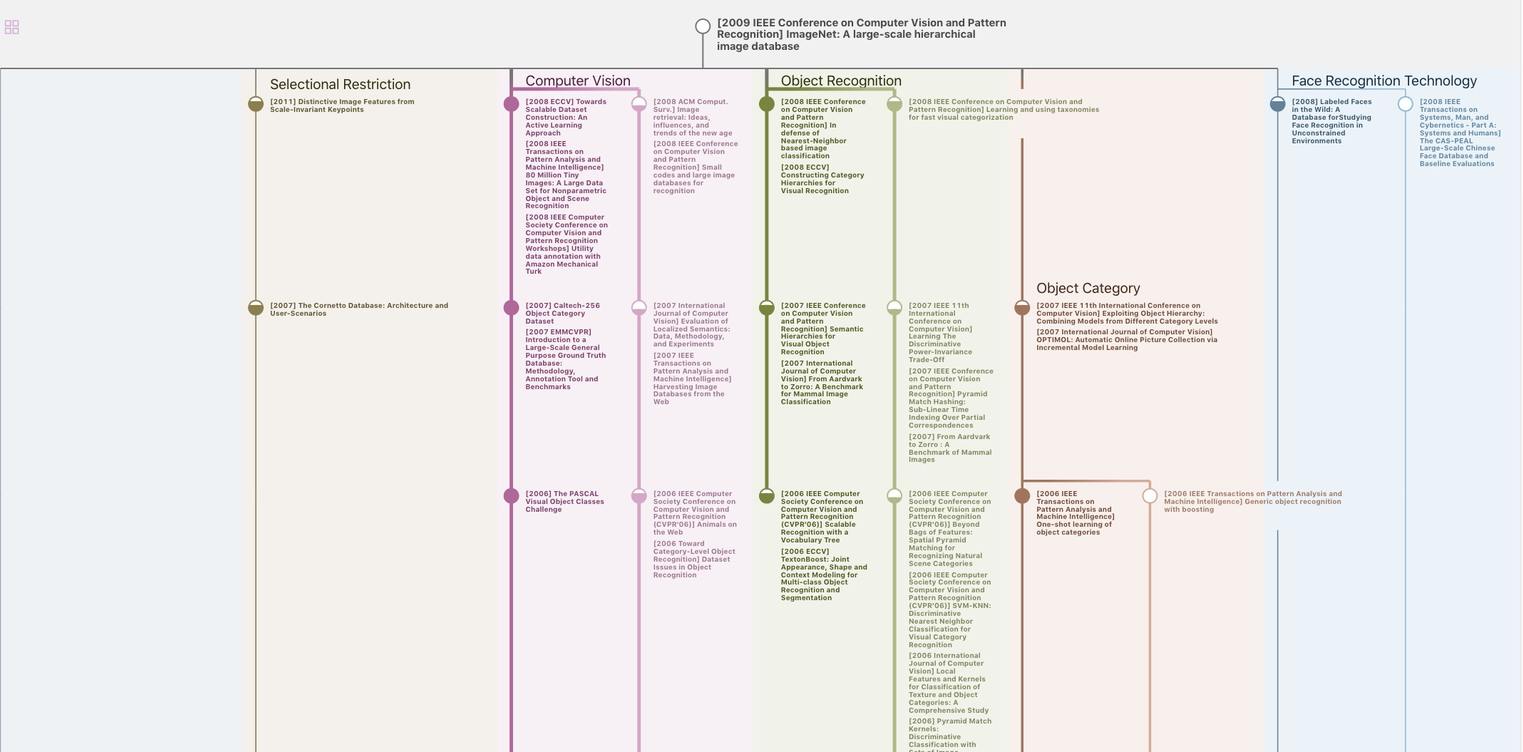
生成溯源树,研究论文发展脉络
Chat Paper
正在生成论文摘要