Assessment of inference accuracy and memory capacity of computation-in-memory enabled neural network due to quantized weights, gradients, input and output signals, and memory non-idealities
JAPANESE JOURNAL OF APPLIED PHYSICS(2024)
摘要
This paper proposes an approach to enhance the efficiency of computation-in-memory (CiM) enabled neural networks. The proposed methods involve partial quantization of learning and inference processes within the neural network to increase the training and inference speed while reducing energy and memory consumption. The impact of quantization due to the usage of CiM is evaluated based on inference accuracy. The effect of non-idealities incurred due to the employment of different memories such as resistive random-access memory on the network accuracy is documented and reported. The results indicate that a certain quantization bit precision threshold is necessary for weights, input/output data, and gradients to maintain an acceptable inference accuracy level. Notably, the experiments demonstrate a modest degradation of approximately 2.8% in inference accuracy compared to the neural network trained without using computation-in-memory, this accuracy trade-off is accompanied by a substantial memory footprint improvement, with memory usage reductions of 62% and 93% during the training and inference phase respectively.
更多查看译文
关键词
computation-in-memory,memory non-idealities,neural networks,quantization,CiM
AI 理解论文
溯源树
样例
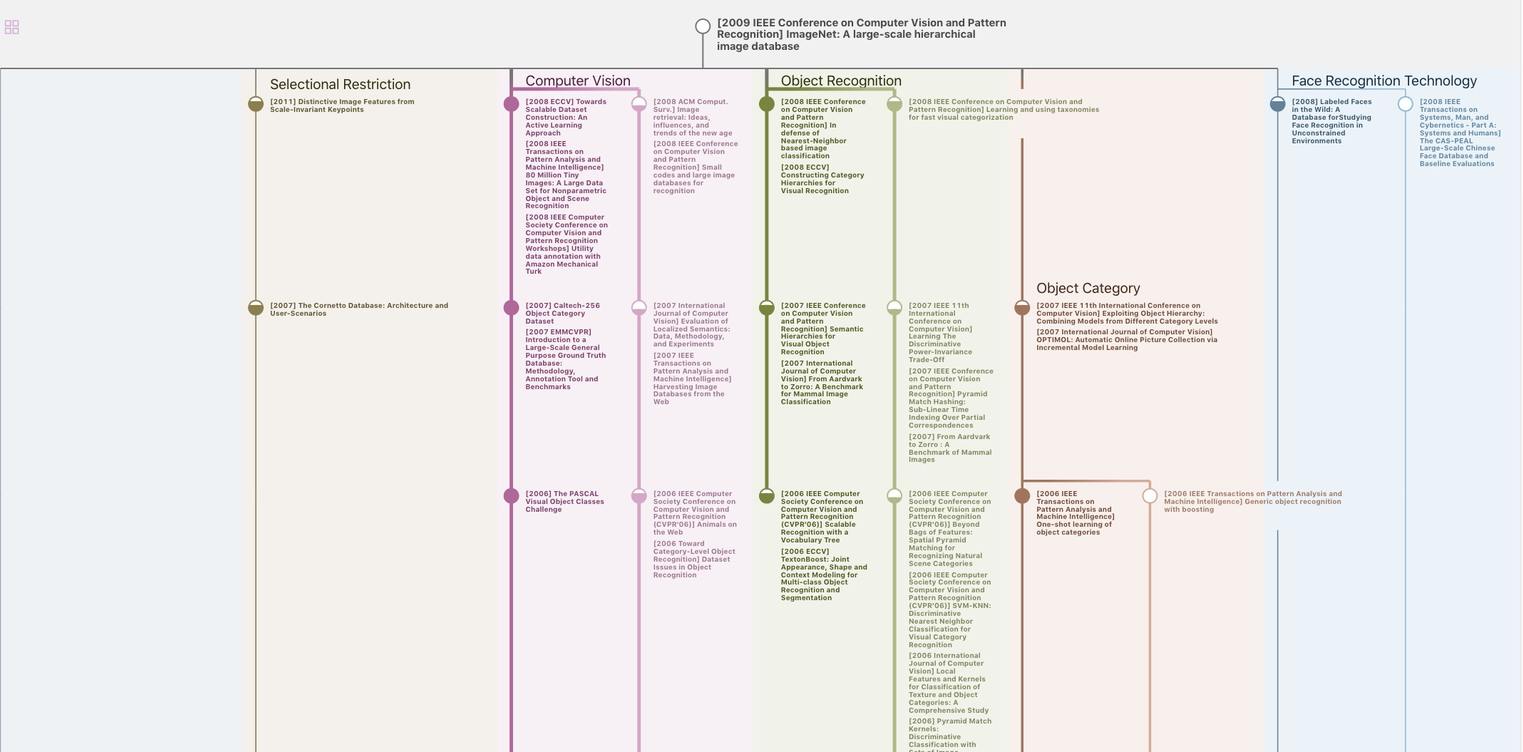
生成溯源树,研究论文发展脉络
Chat Paper
正在生成论文摘要