Deep learning segmentation of the choroid plexus from structural magnetic resonance imaging (MRI): validation and normative ranges across the adult lifespan
FLUIDS AND BARRIERS OF THE CNS(2024)
摘要
Background The choroid plexus functions as the blood-cerebrospinal fluid barrier, plays an important role in neurofluid production and circulation, and has gained increased attention in light of the recent elucidation of neurofluid circulation dysfunction in neurodegenerative conditions. However, methods for routinely quantifying choroid plexus volume are suboptimal and require technical improvements and validation. Here, we propose three deep learning models that can segment the choroid plexus from commonly-acquired anatomical MRI data and report performance metrics and changes across the adult lifespan. Methods Fully convolutional neural networks were trained from 3-D T-1-weighted, 3-D T-2-weighted, and 2-D T-2-weighted FLAIR MRI and gold-standard manual segmentations in healthy and neurodegenerative participants across the lifespan (n=50; age=21-85 years). Dice coefficients, 95% Hausdorff distances, and area-under-curve (AUCs) were calculated for each model and compared to segmentations from FreeSurfer using two-tailed Wilcoxon tests (significance criteria: p<0.05 after false discovery rate multiple comparisons correction). Metrics were regressed against lateral ventricular volume using generalized linear models to assess model performance for varying levels of atrophy. Finally, models were applied to an expanded cohort of healthy adults (n=98; age=21-89 years) to provide an exemplar of choroid plexus volumetry values across the lifespan. Results Deep learning results yielded Dice coefficient=0.72, Hausdorff distance=1.97 mm, AUC=0.87 for T-1-weighted MRI, Dice coefficient=0.72, Hausdorff distance=2.22 mm, AUC=0.87 for T-2-weighted MRI, and Dice coefficient=0.74, Hausdorff distance=1.69 mm, AUC=0.87 for T-2-weighted FLAIR MRI; values did not differ significantly between2 MRI sequences and were statistically improved compared to current commercially-available algorithms (p<0.001). The intraclass coefficients were 0.95, 0.95, and 0.96 between T-1-weighted and T-2-FLAIR, T-1-weighted and T-2-weighted, and T-2-weighted and T-2-FLAIR models, respectively. Mean lateral ventricle choroid plexus volume across all participants was 3.20 +/- 1.4 cm(3); a significant, positive relationship (R-2=0.54; slope=0.047) was observed between participant age and choroid plexus volume for all MRI sequences (p<0.001). Conclusions Findings support comparable performance in choroid plexus delineation between standard, clinically available, non-contrasted anatomical MRI sequences. The software embedding the evaluated models is freely available online and should provide a useful tool for the growing number of studies that desire to quantitatively evaluate choroid plexus structure and function (https://github.com/hettk/chp_seg).
更多查看译文
关键词
Choroid plexus,Deep learning,Glymphatic,Segmentation,Cerebrospinal fluid,Neurofluids
AI 理解论文
溯源树
样例
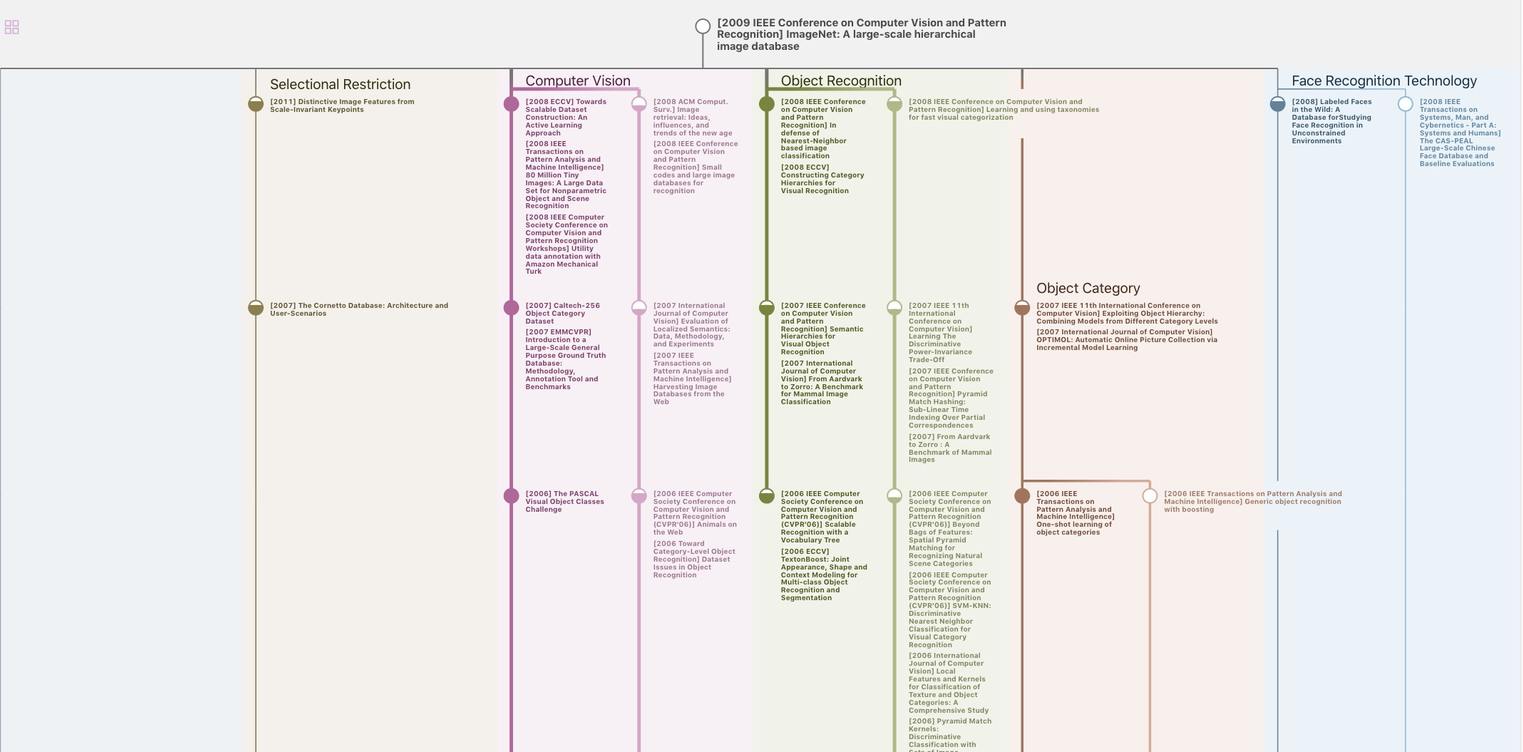
生成溯源树,研究论文发展脉络
Chat Paper
正在生成论文摘要