Sustainable urban energy solutions: Forecasting energy production for hybrid solar-wind systems
ENERGY CONVERSION AND MANAGEMENT(2024)
摘要
In recent years, hybrid Solar-Wind energy system has emerged as a viable solution to achieve sustainable energy generation and alleviate the burden on the power grid. However, enhancing the system configuration to balance energy production and consumption remains a challenging task. In this study, we propose an energy forecasting methodology that leverages transformers as an AI tool to predict energy production from a hybrid PhotovoltaicWind system in an urban environment. The methodology involves pre-processing data, training the transformer model, and generating energy forecasts. The research utilized a one -year dataset, collecting data at 10 -minute intervals, encompassing various parameters like wind speed, wind direction, global horizontal irradiance, direct normal irradiance, and diffuse horizontal irradiance, all for the purpose of wind and solar energy prediction. The study additionally examined how the model's performance is impacted by fine-tuning hyperparameters and revealed that this dependency is inversely proportional to characteristics such as training data, horizon, and learning rate. Hyperparameters such as look back and epoch, on the other hand, were observed to have a direct link with the model's dependency. Multiple simulations have been conducted to fine -tune the hyperparameters of the machine learning model to improve its efficiency. The outcomes exhibited the energy forecasting methodology's effectiveness in predicting energy production for a hybrid Photovoltaic-Wind system in an urban environment. The forecasting accuracy for solar energy and wind speed reached impressive levels, with 90.7% and 90.4% respectively, across various horizons. The time resolution used for forecasting was 10 min, and the data was split into training, testing, and validation sets with ratios of 60-20-20% and 70-15-15%. Leveraging a transformer model for energy forecasting with an accuracy up to 90% holds the promise of streamlining the planning and management of hybrid PV-Wind-Battery storage systems. This, in turn, can play a critical role in advancing the adoption of sustainable and efficient energy solutions for both residential and commercial structures. The code for timeseries data forecasting is available at https://www.kaggle.com/code/ alijavaid43/transformer-model.
更多查看译文
关键词
Hybrid system,Renewable sources,Transformer,Prediction,Artificial intelligence,Islamabad
AI 理解论文
溯源树
样例
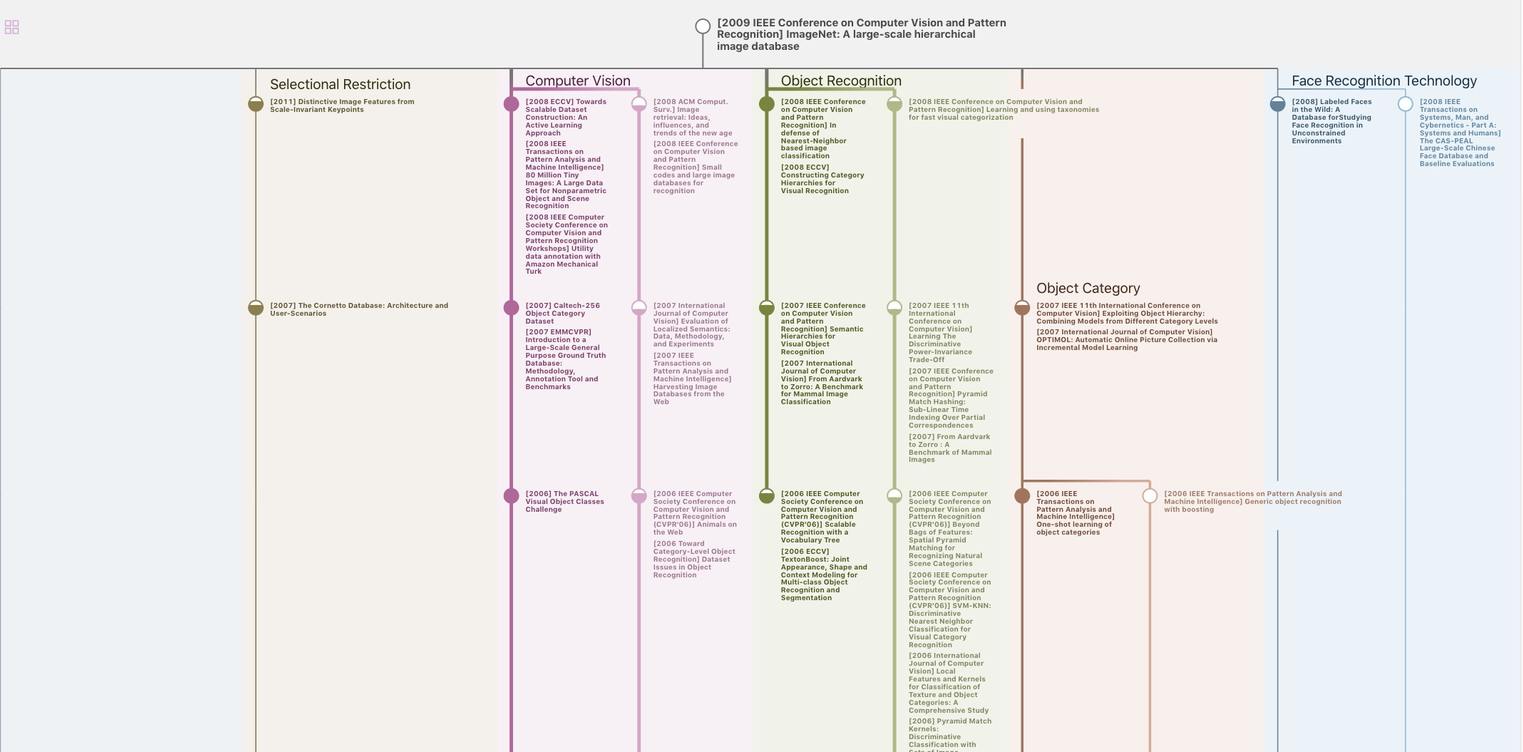
生成溯源树,研究论文发展脉络
Chat Paper
正在生成论文摘要