Characterizing and Improving the Robustness of Predict-Then-Optimize Frameworks
DECISION AND GAME THEORY FOR SECURITY, GAMESEC 2023(2023)
摘要
Optimization tasks situated in incomplete information settings are often preceded by a prediction problem to estimate the missing information; past work shows the traditional predict-then-optimize (PTO) framework can be improved by training a predictive model with respect to the optimization task through a PTO paradigm called decision-focused learning. Little is known, however, about the performance of traditional PTO and decision-focused learning when exposed to adversarial label drift. We provide modifications of traditional PTO and decision-focused learning that attempt to improve robustness by anticipating label drift. When the predictive model is perfectly expressive, we cast these learning problems as Stackelberg games. With these games, we provide a necessary condition for when anticipating label drift can improve the performance of a PTO algorithm: if performance can be improved, then the downstream optimization objective must be asymmetric. We then bound the loss of decision quality in the presence of adversarial label drift to show there may exist a strict gap between the performance of the two algorithms. We verify our theoretical findings empirically in two asymmetric and two symmetric settings. These experimental results demonstrate that robustified decision-focused learning is generally more robust to adversarial label drift than both robust and traditional PTO.
更多查看译文
关键词
predict-then-optimize,adversarial label drift,decision-focused learning
AI 理解论文
溯源树
样例
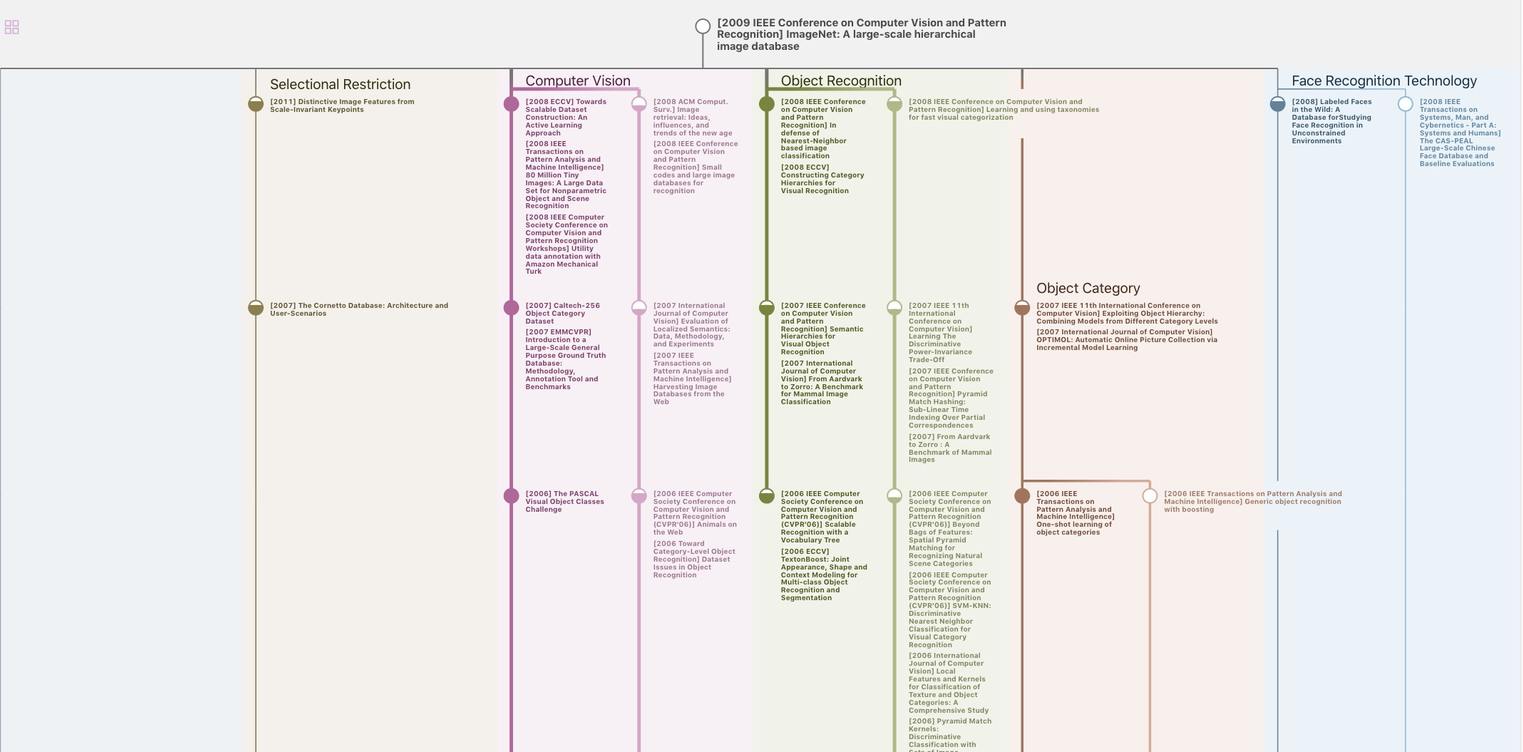
生成溯源树,研究论文发展脉络
Chat Paper
正在生成论文摘要