Rapid and sustainable battery health diagnosis for recycling pretreatment using fast pulse test and random forest machine learning
JOURNAL OF POWER SOURCES(2024)
摘要
This research introduces a novel state of health (SOH) diagnosis method for retired lithium-ion batteries (LIBs) in recycling pretreatment, employing a fast pulse test and random forest machine learning. We highlight the SOH diagnosis for recycling as a highly heterogeneous and no-historical-data issue. A diverse out-of-distribution dataset is collected from 442 retired commercial LIBs, originating from 4 manufacturers, 2 physical formats, 5 capacity designs, and 9 historical usages. Following the pulse test, key features are identified from the voltage response and utilized to instruct machine-learning models. The SOH diagnosis error rates are 1.30% and 1.79%, without any prior knowledge of the historical usage pattern, for training and testing, respectively. Moreover, models can generalize to different capacity designs, cathode materials, and even unseen datasets. Pulse tests substantially curtail the requisite testing time by a minimum of 70%, in comparison to the regular 1A capacity calibration, resulting in a noteworthy economic and environmental advantage. Assuming the SOH of the retired batteries to be 0.5, pulse tests save up to 1443.7 yuan (201.02 dollars) and reduce 65.43kg of CO2 emissions per ton of retired batteries. The findings bolster the efficiency of battery recycling pretreatment, promoting the overall profitability and sustainability of recycling.
更多查看译文
关键词
Battery health diagnosis,Battery recycling,Pulse test,Machine learning,Field data,Economic -environmental analysis
AI 理解论文
溯源树
样例
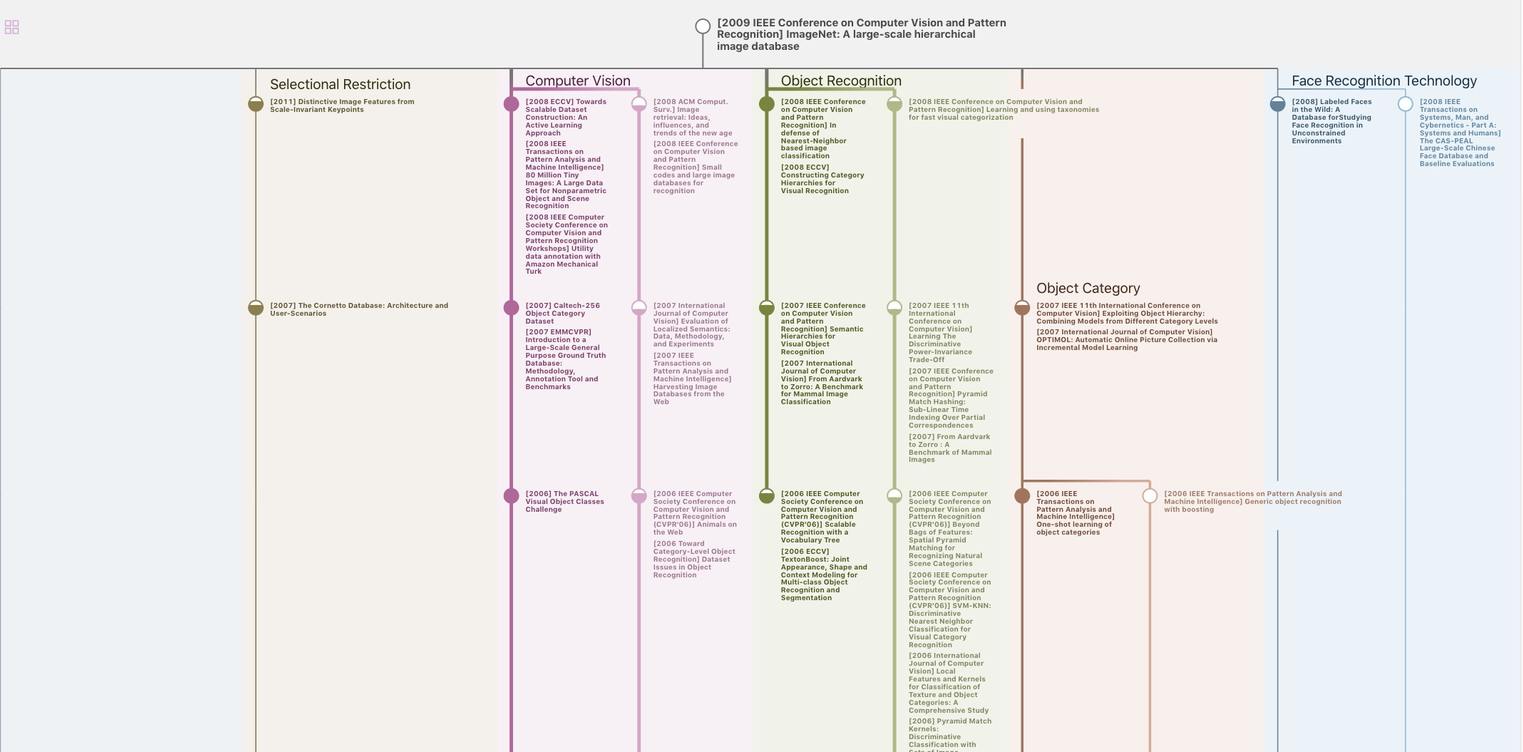
生成溯源树,研究论文发展脉络
Chat Paper
正在生成论文摘要