Deep Learning-Based Classification of Melanoma and Non-Melanoma Skin Cancer
TRAITEMENT DU SIGNAL(2024)
摘要
Melanoma skin cancer is primarily characterized by poor prognostic responses. Surgical treatment can achieve advanced cure rate with early melanoma detection. Manual segmentation of suspected lesions aids early melanoma diagnosis. However, the limitations of manual segmentation include low efficiency and a risk of misclassification. Deep learning, due to its proficiency in image object classification, has gained popularity and is usually used in medical specialties such as ophthalmology, dermatology, and radiology. This paper proposes a deep learning method using a novel light weight convolutional neural networks (LWCNN) and transfer learning techniques (GoogleNet, ResNet-18 & MobilNetv2). These are used to train datasets and features enhancement of skin scan gathered from Kaggle, aiming to distinguish them into two groups: Melanoma and Non-Melanoma cells. By employing these techniques, new datasets with robust features are produced. All CNN models have been tested in two experiments. In firestone, model was tested solely with original datasets and achieved 97.30%, 88.43%, and 48.28% for AC-Training, AC-Testing, and Time (min) respectively. In second experiment, we used the dataset after enhancing the features of skin scan images, which resulted in 99.18%, 91.05%, and 22.54% for ACTraining, AC-Testing, and Time (min) respectively. According to experimental results, the proposed approach provides higher accuracy results for enhanced images than original images, demonstrating its potential in skin cancer classification.
更多查看译文
关键词
image enhancement light wight,convolutional neural networks (LWCNN),,melanoma classification transfer learning
AI 理解论文
溯源树
样例
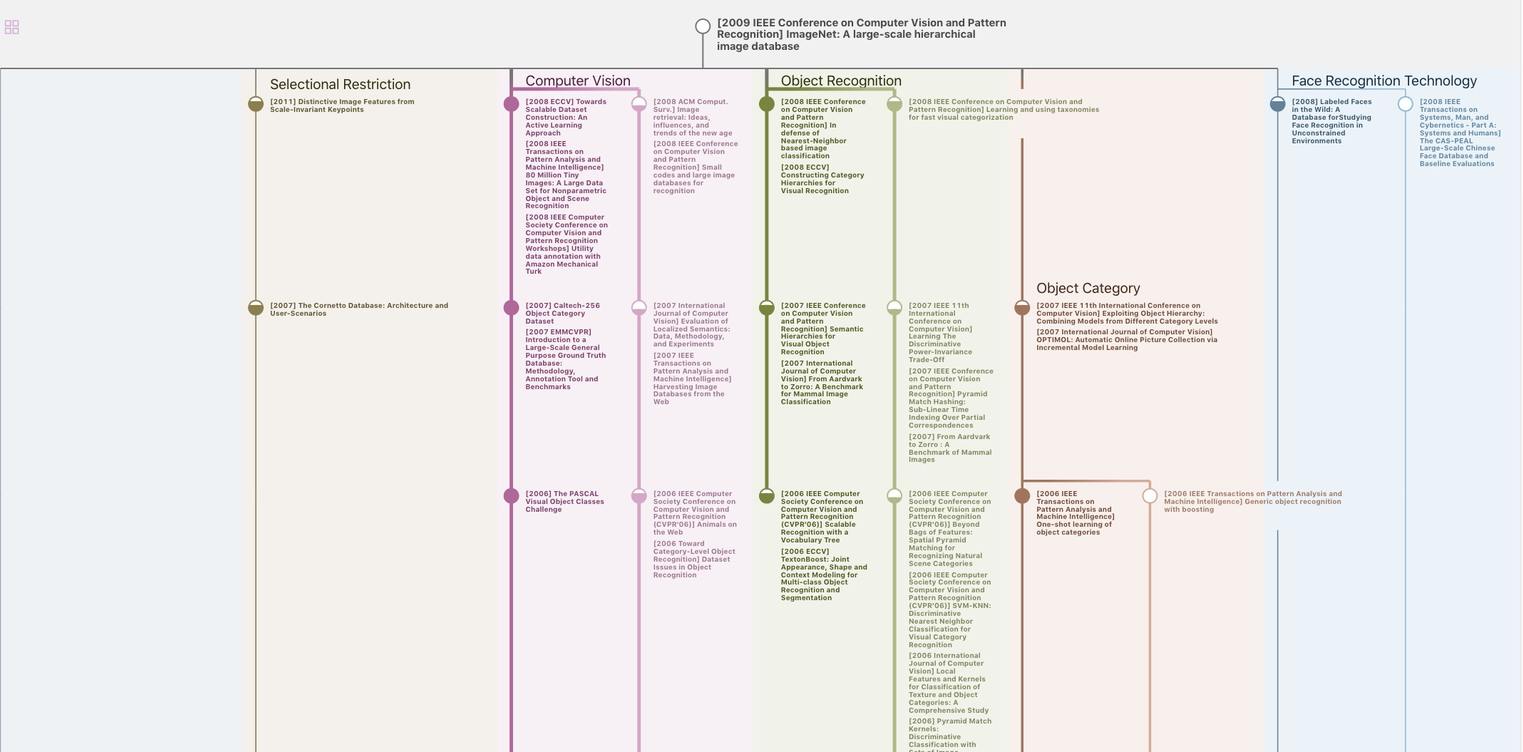
生成溯源树,研究论文发展脉络
Chat Paper
正在生成论文摘要