Dynamic multi-granularity spatial-temporal graph attention network for traffic forecasting
INFORMATION SCIENCES(2024)
摘要
Traffic forecasting, as the cornerstone of the development of intelligent transportation systems, plays a crucial role in facilitating accurate control and management of urban traffic. By treating sensors as nodes in a road network, recent research on modeling complex spatial -temporal graph structures has achieved notable advancements in traffic forecasting. However, limited by the increasing number of sensors and recorded data points, most of the recent studies on spatial -temporal graph neural network (STGNN) research concentrate on aggregating short-term (e.g. recent one -hour) traffic history to predict future data. Furthermore, almost all previous STGNNs neglect to incorporate the cyclical patterns that appear in the traffic historical data. For example, the cyclical patterns of traffic on the same day or hour of each week can help improve the accuracy of future traffic predictions. In this paper, we propose a novel Dynamic Multi -Granularity Spatial -Temporal Graph Attention Network (DmgSTGAT) framework for traffic forecasting, which leverages multi -granularity spatial -temporal correlations across different timescales and variables to efficiently consider cyclical patterns in traffic data. We also design effective temporal encoding and transformer encoding layers to produce meaningful multi -granularity sensor -level, day -level, hour -level, and point -level representations. The multi -granularity spatialtemporal graph attention network can use the produced representations to extract useful but sparsely distributed patterns accurately, which also avoids the influence of extra noise from the long-term history. Experimental results on four real -world traffic datasets show that DmgSTGAT can achieve state-of-the-art performance with the help of multi -granularity cyclical patterns compared with various recent baselines.
更多查看译文
关键词
Spatial-temporal data,Traffic forecasting,Dynamic graph
AI 理解论文
溯源树
样例
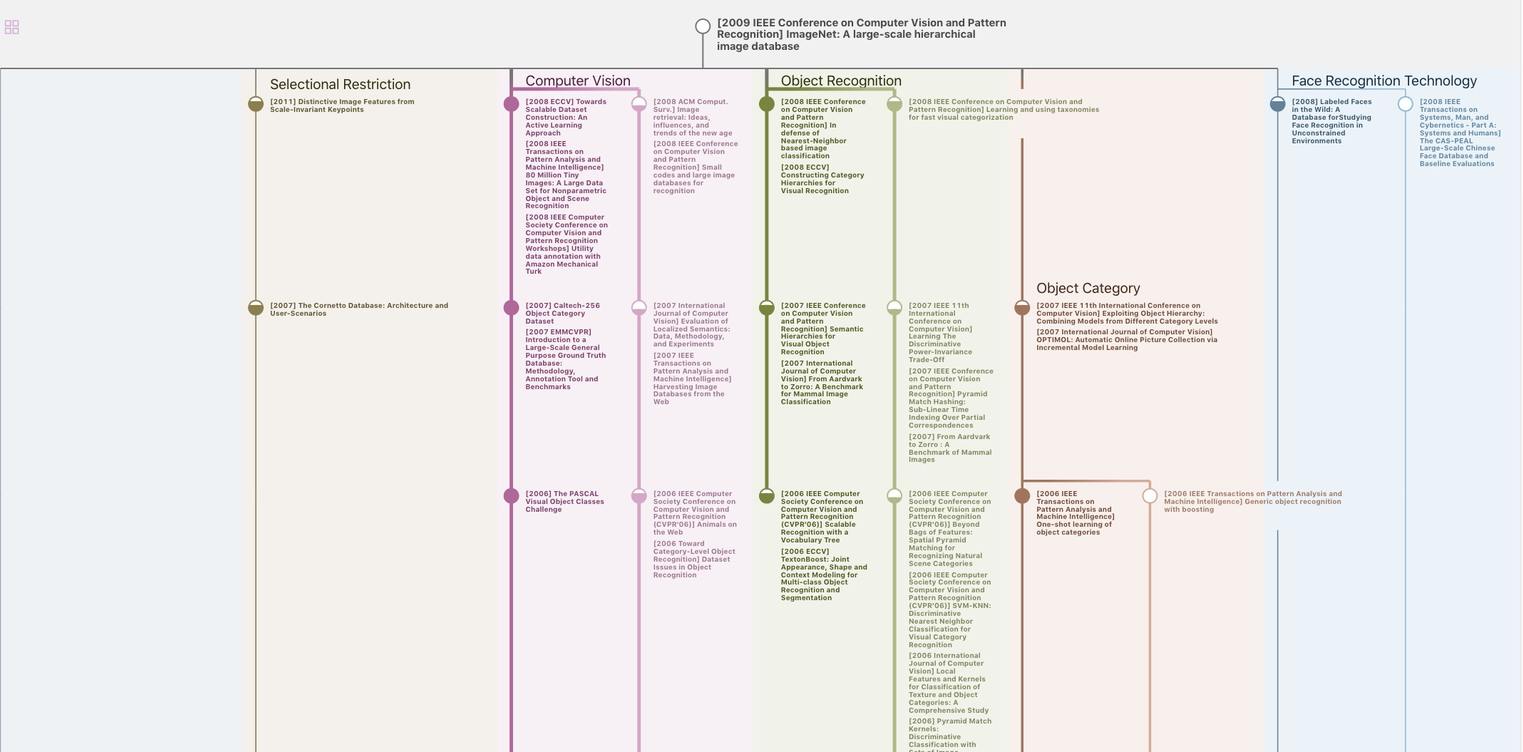
生成溯源树,研究论文发展脉络
Chat Paper
正在生成论文摘要