An extended self-representation model of complex networks for link prediction
INFORMATION SCIENCES(2024)
摘要
As a fundamental problem in network science, link prediction is both theoretically significant and practically useful. Many existing link prediction algorithms rely on predefined assumptions about the link formation mechanism of the network, limiting their generalizability. The recently proposed Network Self-Representation (NSR) model avoids such predefined assumptions by representing the likelihood of link existence between two nodes as a linear transformation of their neighboring connections, resulting in a more generic link prediction approach. To further improve the self-learning capability, this study proposes an Extended Network Self-Representation (ENSR) model. Instead of leveraging a linear transformation as the NSR function, the ENSR model considers the likelihood of link existence as a general function of the adjacency matrix. This study further formulates the ENSR model as an optimization model and analytically derives a critical property of its optimal solution, based on which the optimal ENSR function is approximated by polynomial regression. Experiments on 15 real-world networks show that our proposed ENSR model achieves better link prediction performance than the NSR model as well as several other benchmark algorithms. Notably, the ENSR function's coefficients match the contributions of multi-hop paths to link prediction. In most cases, the self-learned coefficients by the ENSR model align with the predefined assumptions of the second-best link prediction algorithms, which implies that the ENSR model can adaptively discover the underlying link formation mechanisms of complex networks.
更多查看译文
关键词
Link prediction,Network self-representation,Optimization model,Complex network,Polynomial regression
AI 理解论文
溯源树
样例
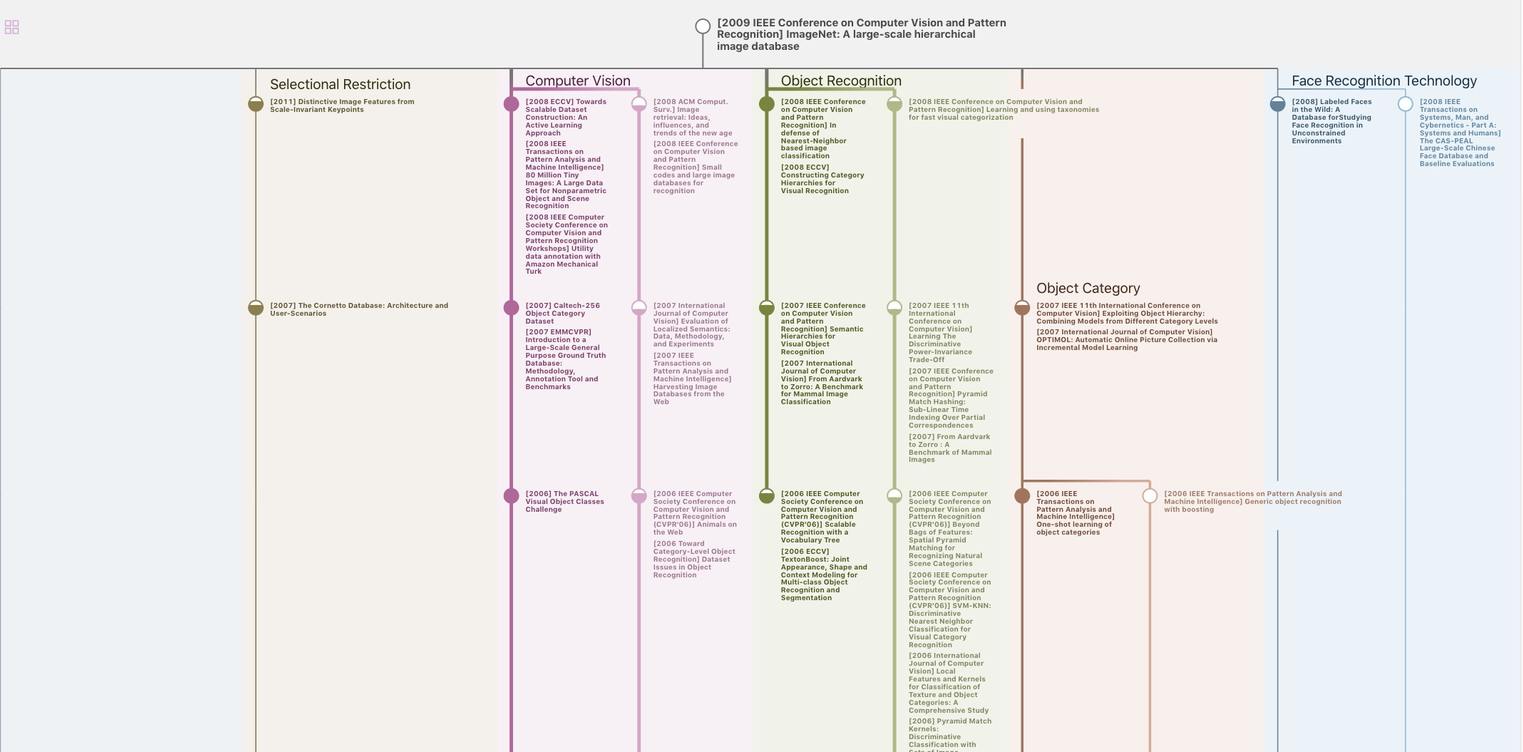
生成溯源树,研究论文发展脉络
Chat Paper
正在生成论文摘要