Cross-domain correlation representation for new fault categories discovery in rolling bearings
INFORMATION PROCESSING & MANAGEMENT(2024)
摘要
Fault diagnosis technology plays a crucial role in preventing faults and mitigating safety hazards, particularly in domains such as nuclear power, aerospace, and manufacturing. However, obtaining an ample number of fault samples is challenging due to the stringent requirements for safe and reliable equipment operation in real production environments. Currently, various deep learning-based techniques have been studied to mitigate this issue. However, these methods often overlook the identification of novel faults that may emerge in the future. In this paper, we present a novel cross-domain correlation representation approach for discovering new fault categories in rolling bearings. Our approach leverages the knowledge acquired through supervised feature extraction to facilitate the discovery process of new fault categories. In particular, we propose a causal inference module to identify fault-related features by eliminating irrelevant information and retaining the primary influential features that contribute to the occurrence of faults. Additionally, we introduce a pseudo-label generation module for the discovery process of unsupervised samples in new fault categories. This module establishes a learning relationship between labeled and unlabeled samples, enabling the utilization of known category knowledge during the discovery of unknown categories. We verify the effectiveness of our model using the CWRU, PU, and MFPT datasets, showing that it outperforms state-of-the-art techniques in terms of clustering accuracy and normalized mutual information.
更多查看译文
关键词
Correlation representation,New fault categories discovery,Causal inference,Pseudo-label generation
AI 理解论文
溯源树
样例
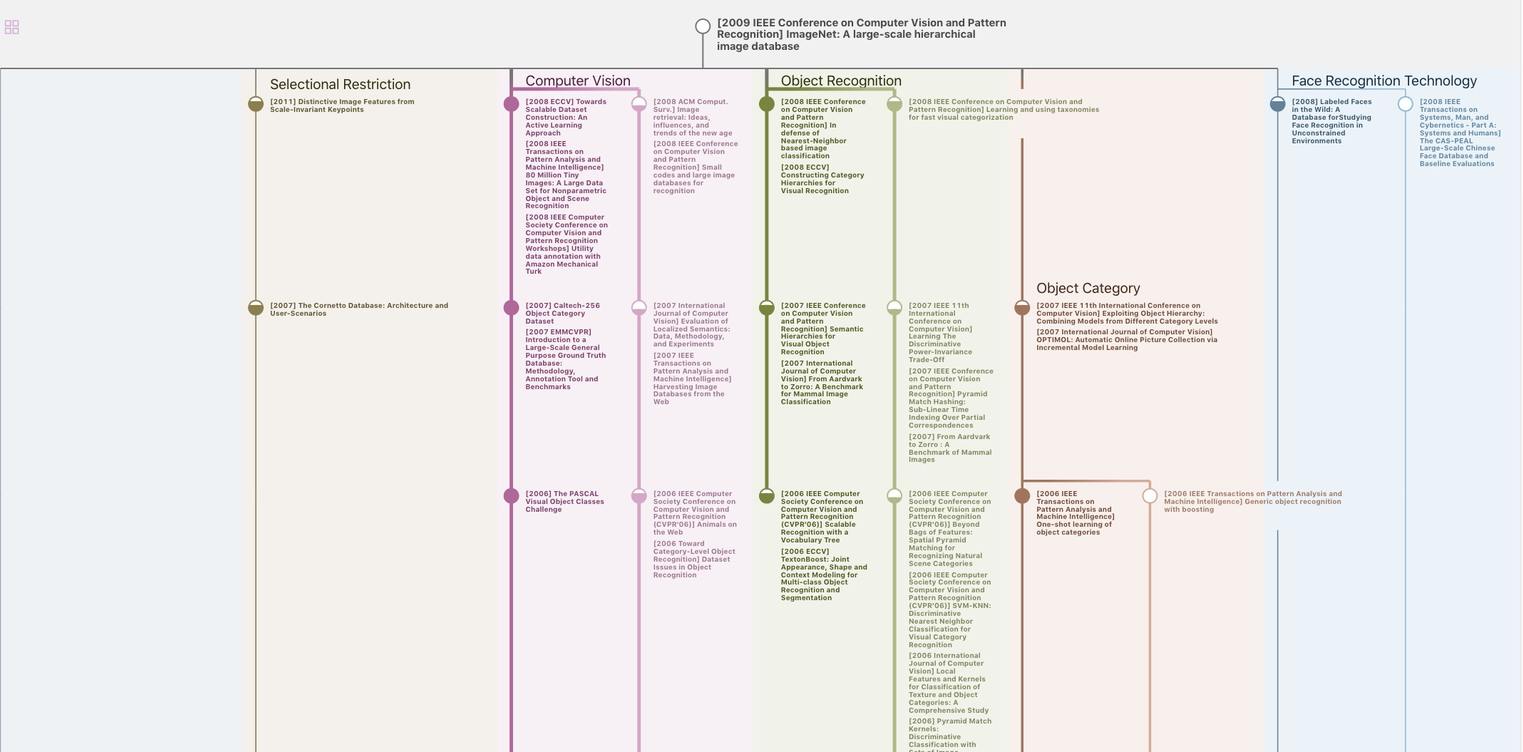
生成溯源树,研究论文发展脉络
Chat Paper
正在生成论文摘要