Learning optimal inter-class margin adaptively for few-shot class-incremental learning via neural collapse-based meta-learning
INFORMATION PROCESSING & MANAGEMENT(2024)
摘要
Few -Shot Class -Incremental Learning (FSCIL) aims to learn new classes incrementally with a limited number of samples per class. It faces issues of forgetting previously learned classes and overfitting on few -shot classes. An efficient strategy is to learn features that are discriminative in both base and incremental sessions. Current methods improve discriminability by manually designing inter -class margins based on empirical observations, which can be suboptimal. The emerging Neural Collapse (NC) theory provides a theoretically optimal inter -class margin for classification, serving as a basis for adaptively computing the margin. Yet, it is designed for closed, balanced data, not for sequential or few -shot imbalanced data. To address this gap, we propose a Meta -learning- and NC -based FSCIL method, MetaNC-FSCIL, to compute the optimal margin adaptively and maintain it at each incremental session. Specifically, we first compute the theoretically optimal margin based on the NC theory. Then we introduce a novel loss function to ensure that the loss value is minimized precisely when the inter -class margin reaches its theoretically best. Motivated by the intuition that "learn how to preserve the margin"matches the meta-learning's goal of "learn how to learn", we embed the loss function in base -session meta -training to preserve the margin for future meta -testing sessions. Experimental results demonstrate the effectiveness of MetaNC-FSCIL, achieving superior performance on multiple datasets. The code is available at https://github.com/qihangran/metaNC-FSCIL.
更多查看译文
关键词
Few-shot class-incremental learning,Neural collapse,Meta-learning
AI 理解论文
溯源树
样例
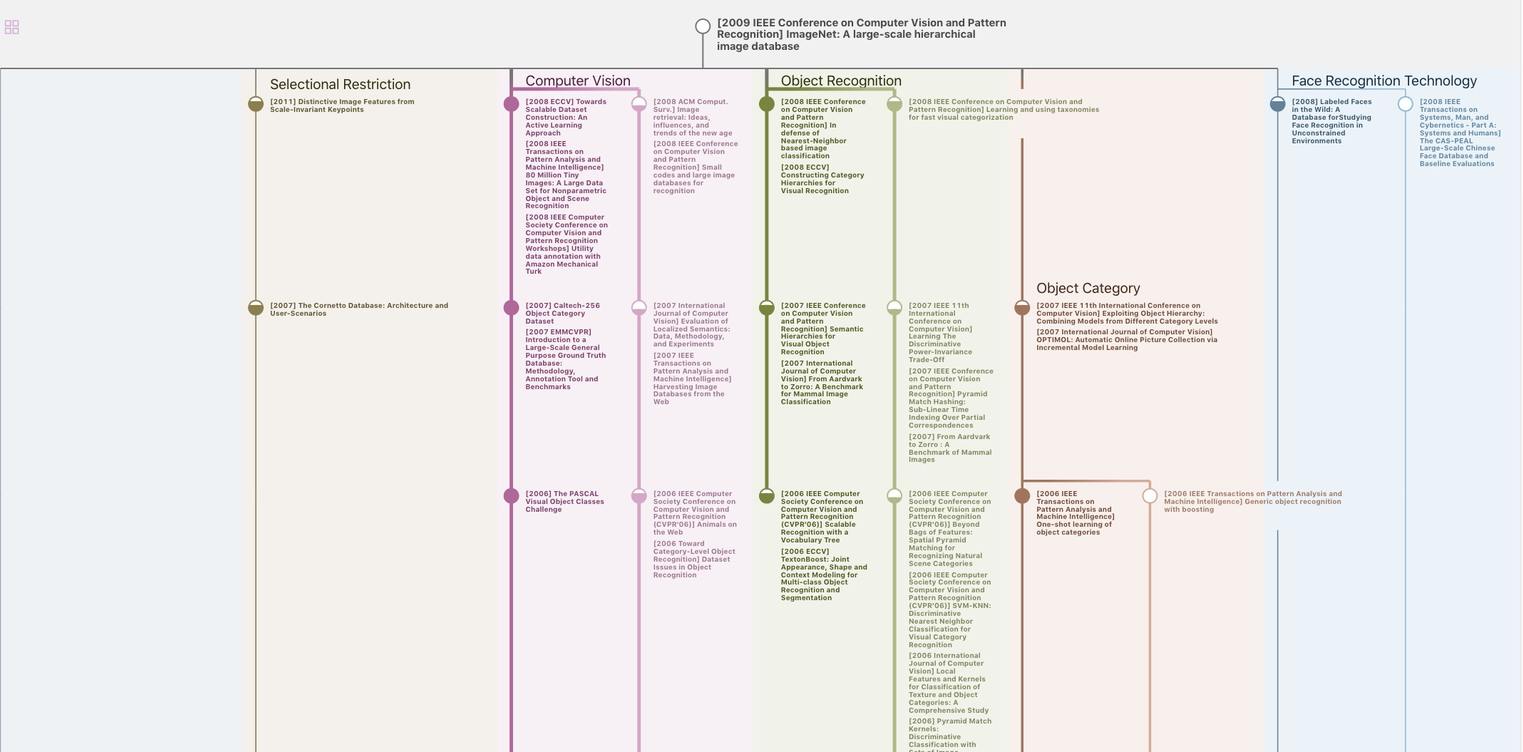
生成溯源树,研究论文发展脉络
Chat Paper
正在生成论文摘要