Simplification of ANFIS based on importance-confidence-similarity measures
FUZZY SETS AND SYSTEMS(2024)
摘要
Adaptive -network -based fuzzy inference system (ANFIS) is a well-known neuro-fuzzy system, which is widely implemented in nonlinear system approximation, prediction, control, and pattern classification. However, either too many fuzzy sets or fuzzy rules are indispensable to establish an accurate ANFIS for a high -dimensional system, which increases the complexity and limits the widespread application of the ANFIS. In this paper, an approach to simplify the ANFIS is developed based on importance -confidence -similarity (ICS) measures. The simplification process consists of selection of fuzzy rules with large importance -confidence (IC) values and reduction of similar fuzzy rules. The importance is defined as the average normalized firing strength of the rule. The confidence which reflects the approximation performance of a T -S fuzzy rule is estimated by the weighted orthogonal distance from the output data to the rule hyperplane (the consequent of a linear T -S fuzzy rule). The IC measures provide a comprehensive basis for the selection of the appropriate fuzzy rules. Furthermore, to the best of our knowledge, an approach to measure similarities of the T -S fuzzy rules including the rule premises and consequents is first proposed in this paper. We also develop a direct and simple method to remove the similar fuzzy rules based on a hierarchical tree and the IC measures. The proposed simplification approach is applied to Mackey -Glass chaotic time series prediction problem and the strip temperature increment prediction problem of the indirect -fired furnace of the annealing heating line. The results show that our proposed method achieves a balance between simplicity and accuracy of the ANFIS for the high -dimensional system.
更多查看译文
关键词
ANFIS,Structure simplification,Grid partition,Fuzzy C-means,Similarity measure,Chaotic time series
AI 理解论文
溯源树
样例
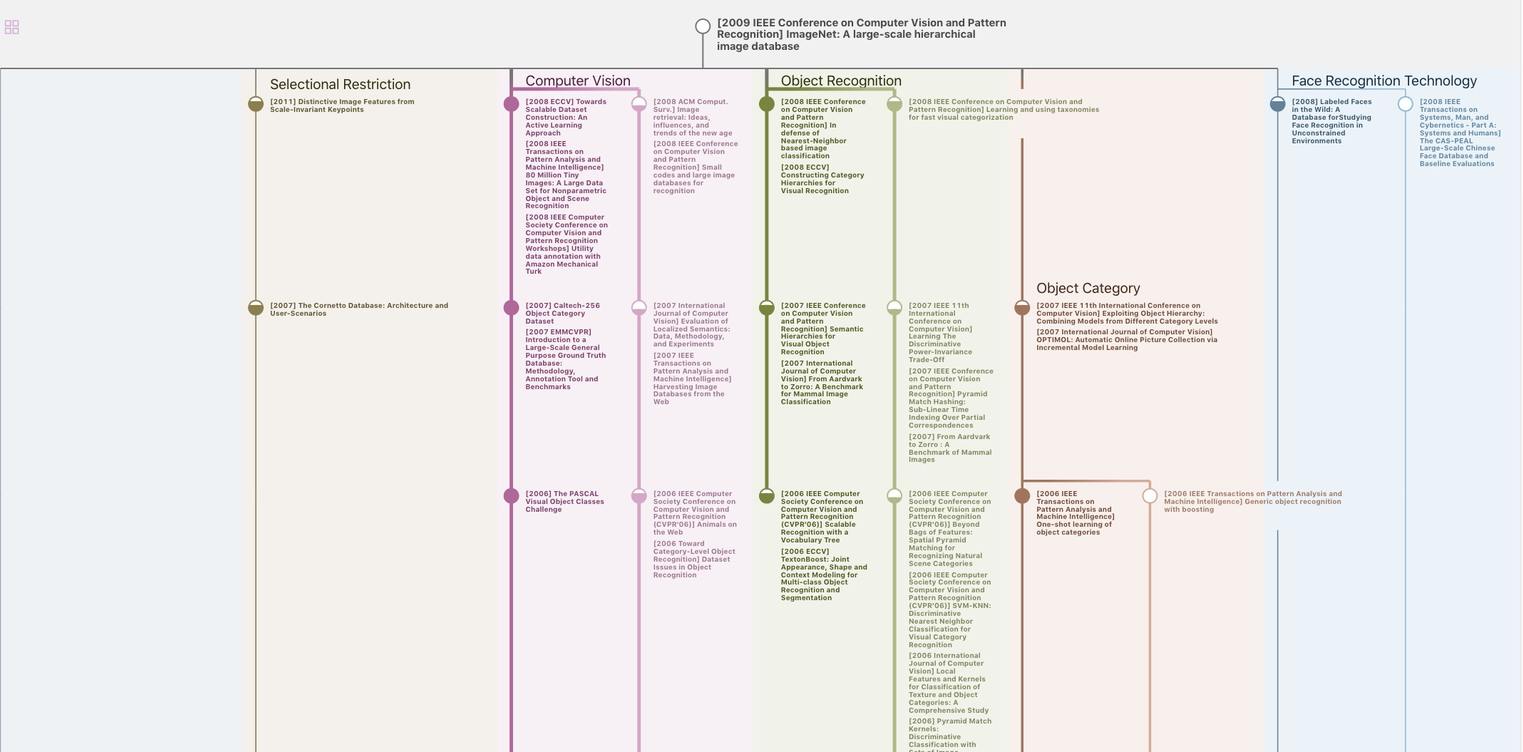
生成溯源树,研究论文发展脉络
Chat Paper
正在生成论文摘要